Weakly Supervised Few-Shot Segmentation via Meta-Learning
IEEE TRANSACTIONS ON MULTIMEDIA(2023)
摘要
Semantic segmentation is a classic computer vision task with multiple applications, which includes medical and remote sensing image analysis. Despite recent advances with deep-based approaches, labeling samples (pixels) for training models is laborious and, in some cases, unfeasible. In this paper, we present two novel meta-learning methods, named WeaSeL and ProtoSeg, for the few-shot semantic segmentation task with sparse annotations. We conducted an extensive evaluation of the proposed methods in different applications (12 datasets) in medical imaging and agricultural remote sensing, which are very distinct fields of knowledge and usually subject to data scarcity. The results demonstrated the potential of our method, achieving suitable results for segmenting both coffee/orange crops and anatomical parts of the human body in comparison with full dense annotation.
更多查看译文
关键词
Agriculture,few-shot,medical imaging analysis,meta learning,remote sensing,semantic segmentation,weakly supervised
AI 理解论文
溯源树
样例
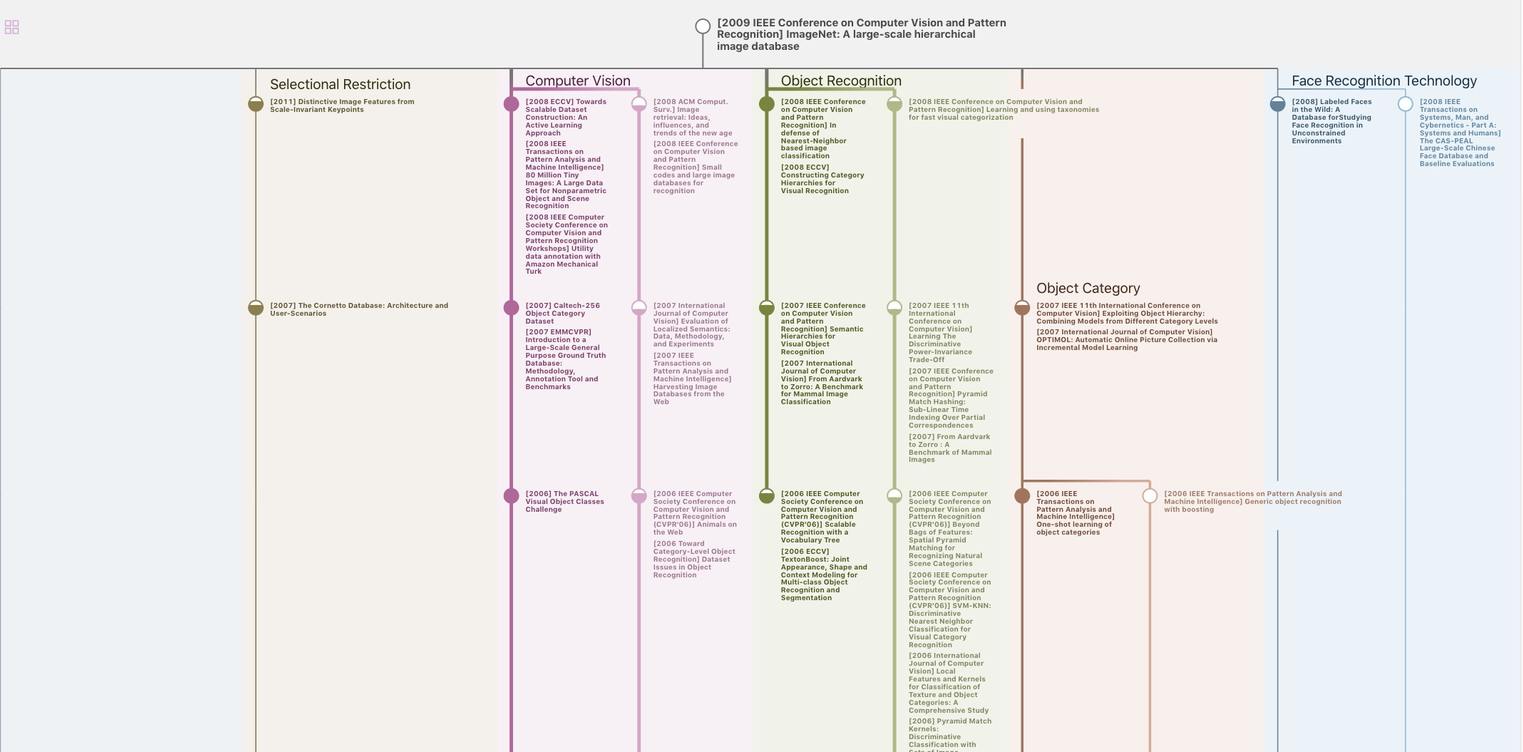
生成溯源树,研究论文发展脉络
Chat Paper
正在生成论文摘要