An Asymptotically Optimal Algorithm For Classification of Data Vectors with Independent Non-Identically Distributed Elements
2021 IEEE INTERNATIONAL SYMPOSIUM ON INFORMATION THEORY (ISIT)(2021)
摘要
In this paper, we propose a classifier for classification of data vectors with mutually independent but not identically distributed elements. For the proposed classifier, we prove that the error probability goes to zero as the length of the data vectors goes to infinity, even when there is only one training data vector per label available. Finally, we present numerical examples where we show that the performance of the proposed classifier outperforms conventional classification algorithms when the number of training data is small.
更多查看译文
关键词
Classification, Error probability, Independent but not identically distribution
AI 理解论文
溯源树
样例
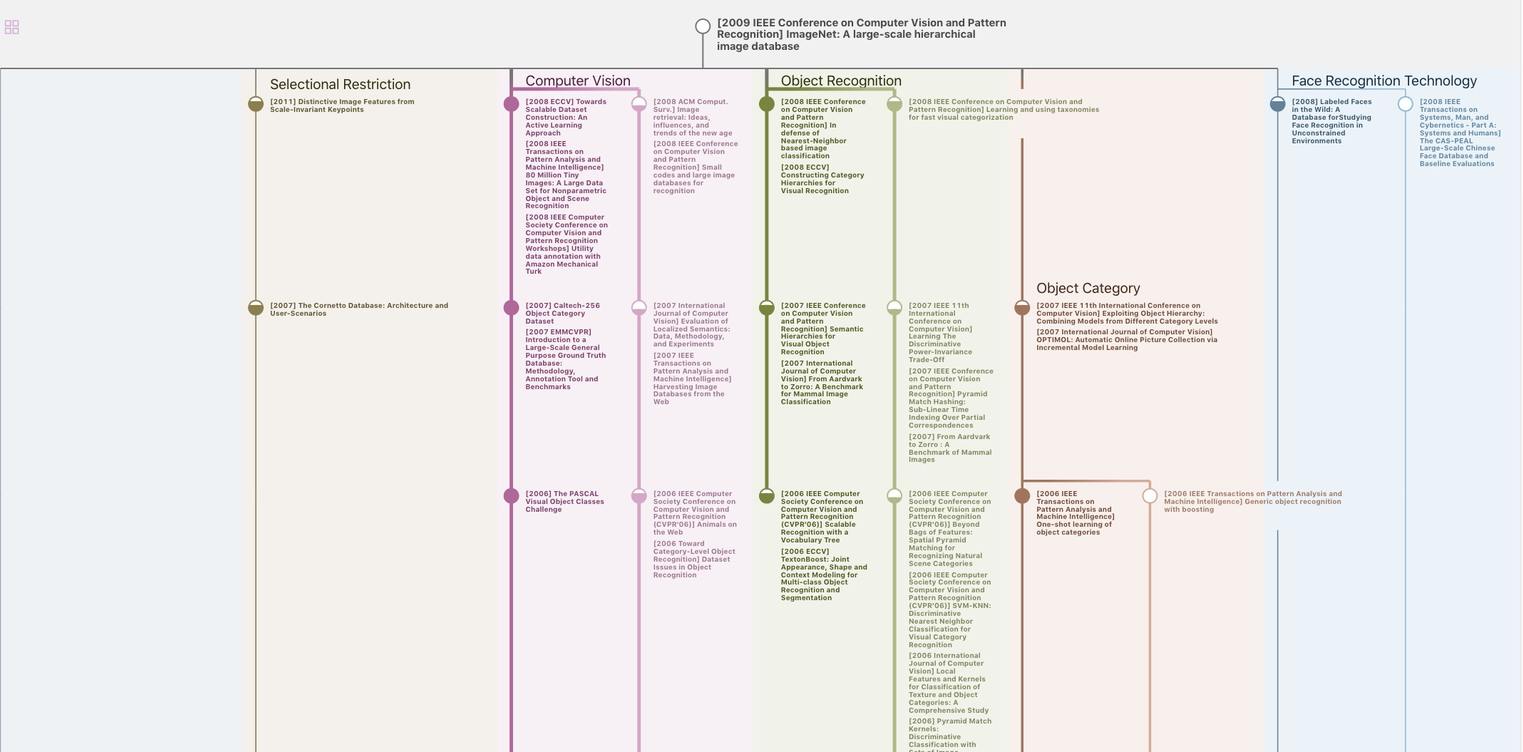
生成溯源树,研究论文发展脉络
Chat Paper
正在生成论文摘要