Adversarial imitation learning with mixed demonstrations from multiple demonstrators
Neurocomputing(2021)
Abstract
The aim of generative adversarial imitation learning (GAIL) is to allow an agent to learn an optimal policy from demonstrations via an adversarial training process. However, previous works have not considered a realistic setting for complex continuous control tasks such as robot manipulation, in which the available demonstrations are imperfect and possibly originate from different policies. Such a setting poses significant challenges for the application of the GAIL-related methods. This paper proposes a novel imitation learning (IL) algorithm, MD2-GAIL, to enable an agent to learn effectively from imperfect demonstrations by multiple demonstrators. Instead of training the policy from scratch, unsupervised pretraining is used to speed up the adversarial learning process. Confidence scores representing the quality of the demonstrations are utilized to reconstruct the objective function for off-policy adversarial training, making the policy match the optimal occupancy measure. Based on the Soft Actor Critic (SAC) algorithm, MD2-GAIL incorporates the idea of maximum entropy into the process of optimizing the objective function. Meanwhile, a reshaped reward function is adopted to update the agent policy to avoid falling into local optima.Experiments were conducted based on robotic simulation tasks, and the results show that our method can efficiently learn from the available demonstrations and achieves better performance than other state-of-the-art methods.
MoreTranslated text
Key words
Adversarial imitation learning,Robot learning,Imperfect demonstrations,Multiple demonstrators
AI Read Science
Must-Reading Tree
Example
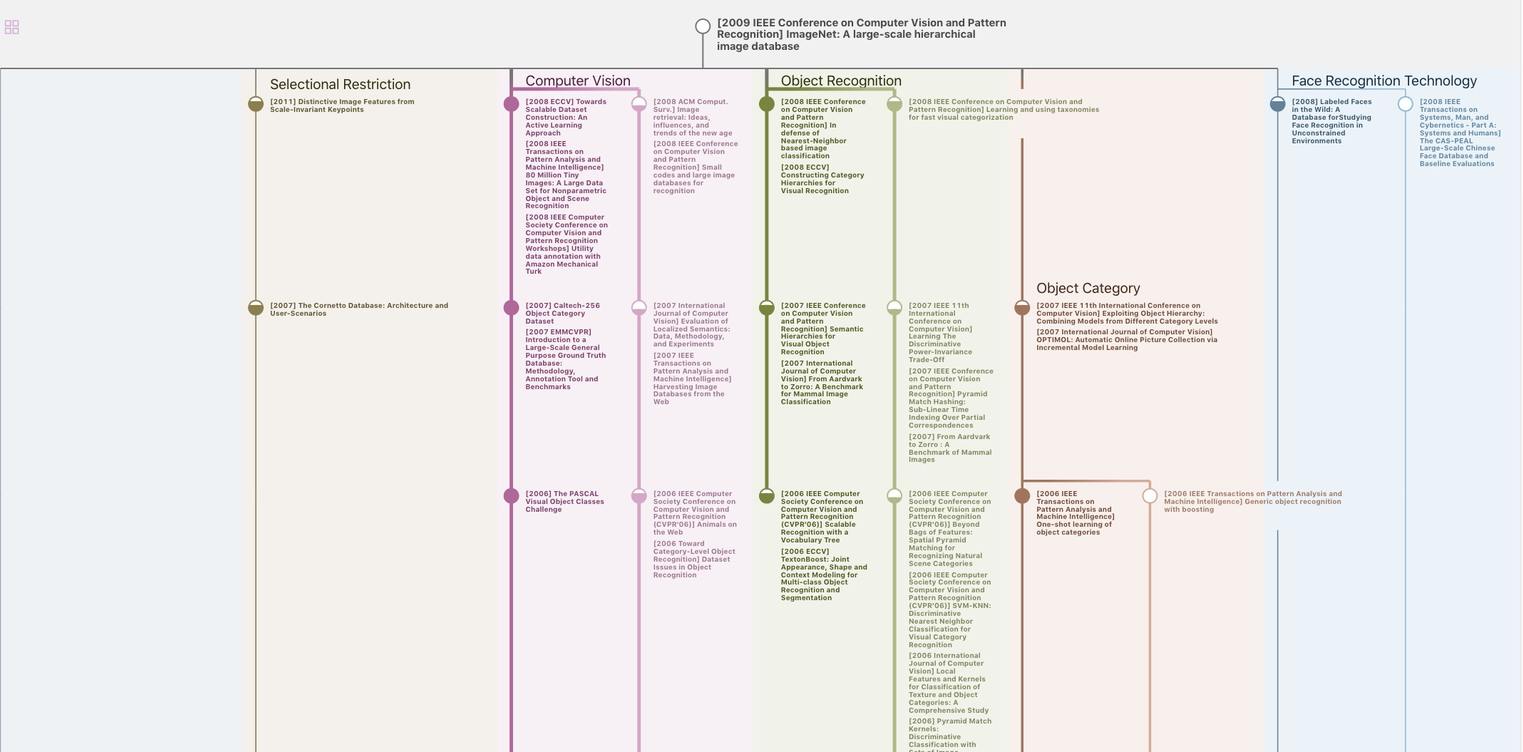
Generate MRT to find the research sequence of this paper
Chat Paper
Summary is being generated by the instructions you defined