Real-Time Volumetric-Semantic Exploration and Mapping: An Uncertainty-Aware Approach
2021 IEEE/RSJ INTERNATIONAL CONFERENCE ON INTELLIGENT ROBOTS AND SYSTEMS (IROS)(2021)
摘要
In this work we propose a holistic framework for autonomous aerial inspection tasks, using semantically-aware, yet, computationally efficient planning and mapping algorithms. The system leverages state-of-the-art receding horizon exploration techniques for next-best-view (NBV) planning with geometric and semantic segmentation information provided by state-of-the-art deep convolutional neural networks (DCNNs), with the goal of enriching environment representations. The contributions of this article are threefold, first we propose an efficient sensor observation model, and a reward function that encodes the expected information gains from the observations taken from specific view points. Second, we extend the reward function to incorporate not only geometric but also semantic probabilistic information, provided by a DCNN for semantic segmentation that operates in real-time. The incorporation of semantic information in the environment representation allows biasing exploration towards specific objects, while ignoring task-irrelevant ones during planning. Finally, we employ our approaches in an autonomous drone shipyard inspection task. A set of simulations in realistic scenarios demonstrate the efficacy and efficiency of the proposed framework when compared with the state-of-the-art.
更多查看译文
关键词
autonomous aerial inspection tasks,mapping algorithms,semantic segmentation,deep convolutional neural networks,environment representation,sensor observation model,reward function,information gains,autonomous drone shipyard inspection task,semantic probabilistic information,DCNN,realtime volumetric-semantic exploration,next-best-view planning,NBV planning
AI 理解论文
溯源树
样例
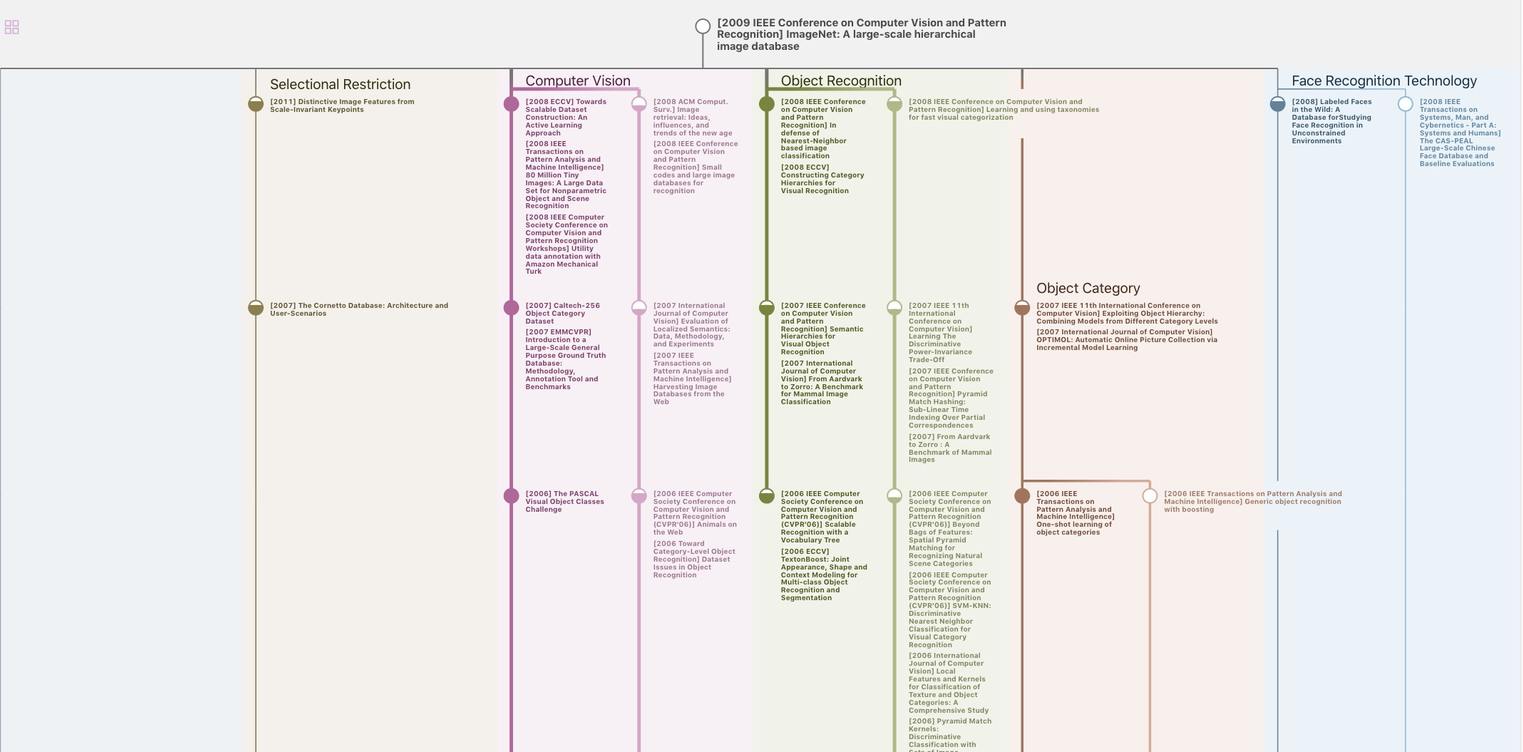
生成溯源树,研究论文发展脉络
Chat Paper
正在生成论文摘要