Video Pose Distillation for Few-Shot, Fine-Grained Sports Action Recognition.
ICCV(2021)
摘要
Human pose is a useful feature for fine-grained sports action understanding. However, pose estimators are often unreliable when run on sports video due to domain shift and factors such as motion blur and occlusions. This leads to poor accuracy when downstream tasks, such as action recognition, depend on pose. End-to-end learning circumvents pose, but requires more labels to generalize. We introduce Video Pose Distillation (VPD), a weakly-supervised technique to learn features for new video domains, such as individual sports that challenge pose estimation. Under VPD, a student network learns to extract robust pose features from RGB frames in the sports video, such that, whenever pose is considered reliable, the features match the output of a pretrained teacher pose detector. Our strategy retains the best of both pose and end-to-end worlds, exploiting the rich visual patterns in raw video frames, while learning features that agree with the athletes' pose and motion in the target video domain to avoid over-fitting to patterns unrelated to athletes' motion. VPD features improve performance on few-shot, fine-grained action recognition, retrieval, and detection tasks in four real-world sports video datasets, without requiring additional ground-truth pose annotations.
更多查看译文
关键词
Transfer/Low-shot/Semi/Unsupervised Learning,Action and behavior recognition,Gestures and body pose,Representation learning,Video analysis and understanding
AI 理解论文
溯源树
样例
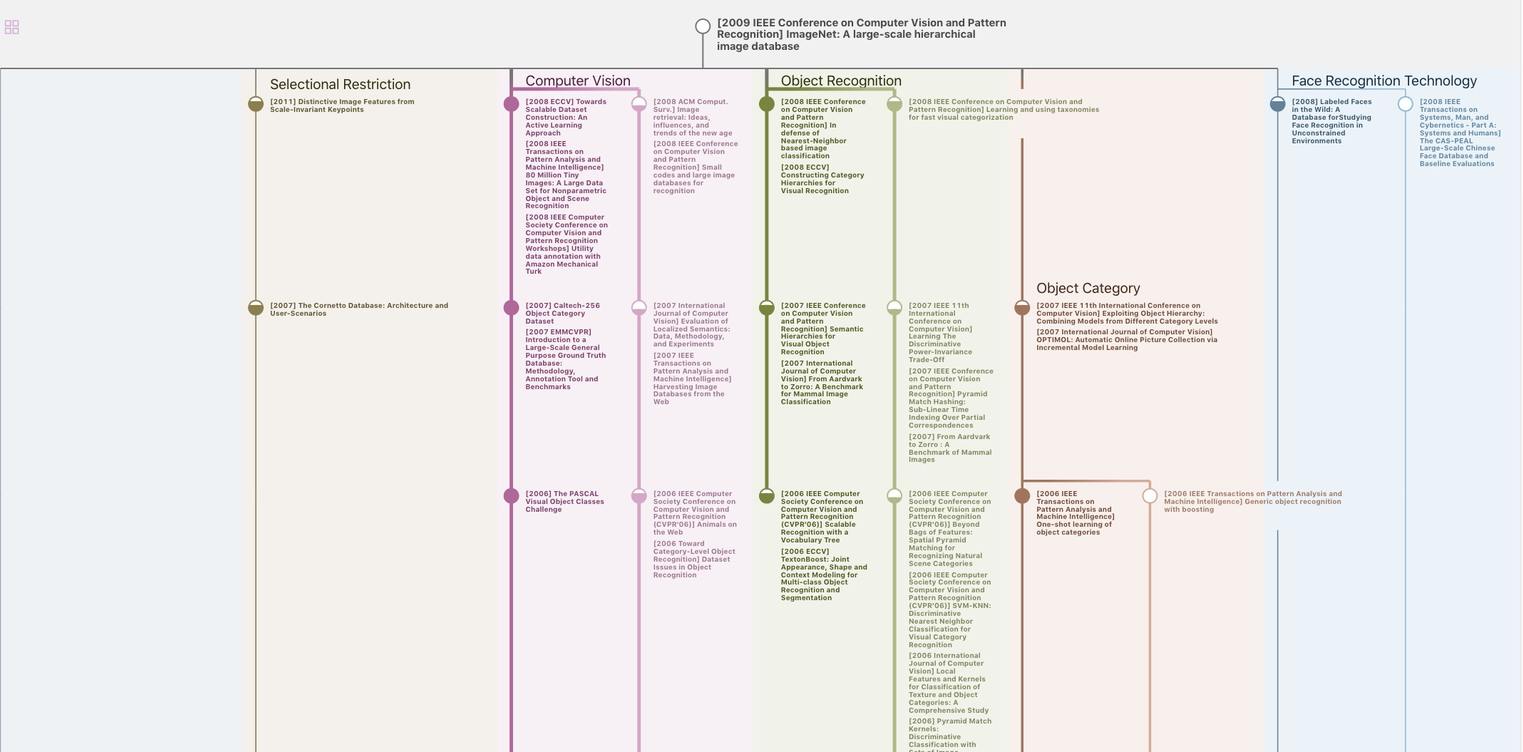
生成溯源树,研究论文发展脉络
Chat Paper
正在生成论文摘要