Federated conditional generative adversarial nets imputation method for air quality missing data
Knowledge-Based Systems(2021)
摘要
The air quality is a topic of extreme concern that attracts a lot of attention in the world. Many intelligent air quality monitoring networks have been deployed in various places, especially in big cities. These monitoring networks collect air quality data with some missing data for some reasons which pose an obstacle for air quality publishing and studies. Generative adversarial nets (GAN) methods have achieved state-of-the-art performance in missing data imputation. GAN-based imputation method needs enough training data while one monitoring network has just a few and poor quality monitoring data and these data sets do not meet the independent identical distribution (IID) condition. Therefore, one monitoring network side needs to utilize more monitoring data from other sides as far as possible. However, in the real world, these air quality monitoring networks are owned by different organizations — companies, the government even some secret units. Many of them cannot share detailed monitoring data due to security, privacy, and industrial competition. In this paper, it is the first time to propose a conditional GAN imputation method under a federated learning framework to solve the data sets that come from diverse data-owners without sharing. Furthermore, we improve the vanilla conditional GAN performance with Wasserstein distance and “Hint mask” trick. The experimental results show that our GAN-based imputation methods can achieve the best performance. And our federated GAN imputation method outperforms the GAN imputation method trained locally for each participant which means our imputation model can work. Our proposed federated GAN method can benefit model quality by increasing access to air quality data through private multi-institutional collaborations. We further investigate the effects of data geographical distribution across collaborating participants on model quality and, interestingly, we find that the GAN training process with a federated learning framework performs more stable.
更多查看译文
关键词
Air pollutants,Conditional GAN imputation,Federated learning,Privacy-preserving machine learning
AI 理解论文
溯源树
样例
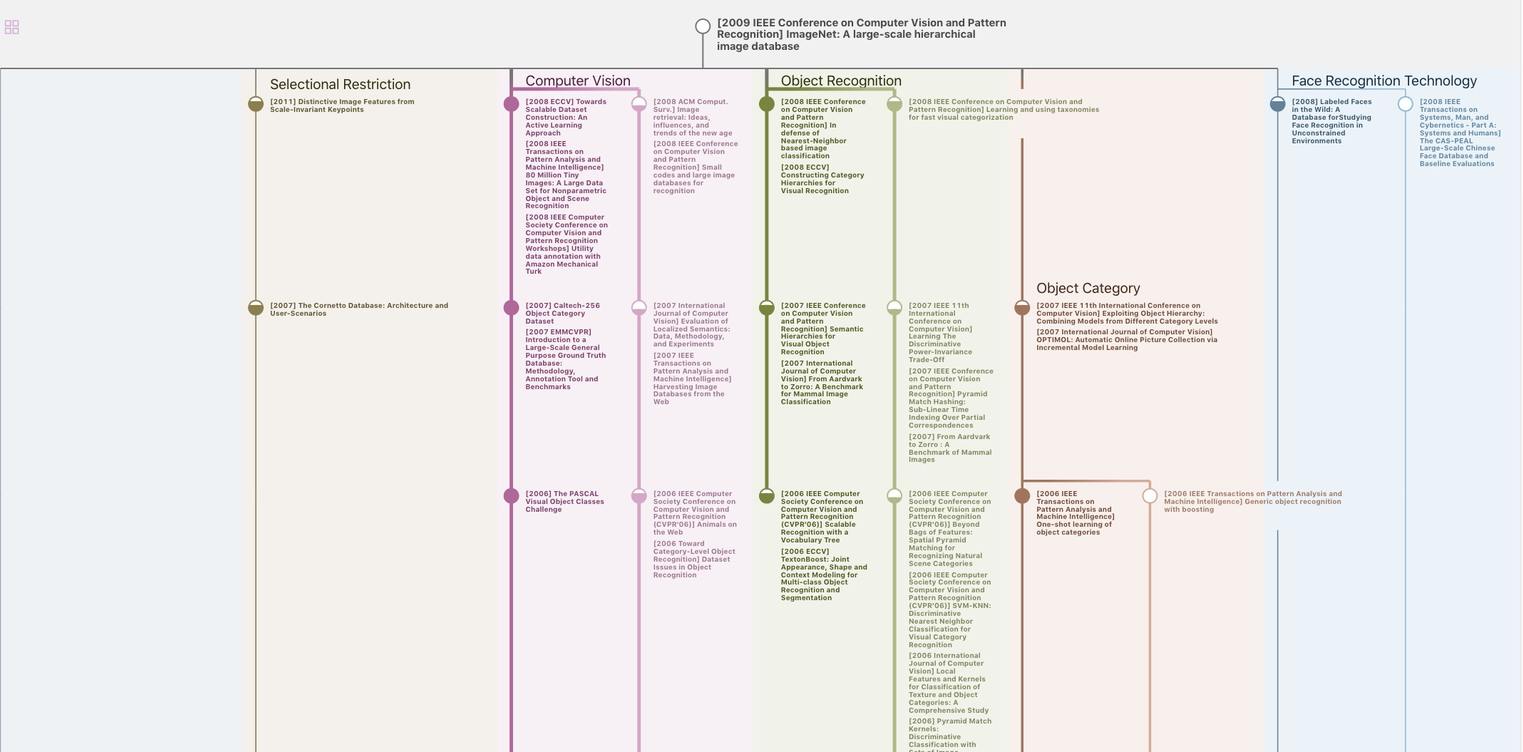
生成溯源树,研究论文发展脉络
Chat Paper
正在生成论文摘要