Double level set segmentation model based on mutual exclusion of adjacent regions with application to brain MR images
Knowledge-Based Systems(2021)
摘要
Accurately segmenting adjacent regions in images is one of the greatest challenges in image processing. In this paper, we introduce a double level set segmentation model based on mutual exclusion, which can accurately and independently segment adjacent regions. In order to avoid the creation of intersection regions and ensure the independence of neighboring regions, the model uses two level set functions, and defines the mutual exclusion term of neighboring regions according to the area of the joint region segmented by the two level set functions. Furthermore, we manually set the approximate contours of the target region as a prior information, defining prior constraints in the energy functional to ensure that the model only segments the organization we wanted. Combining the data term, the mutual exclusion term, the prior constraint term, the length term and the regularization term, we give the level set formulation of this double level sets energy functional and apply the gradient descent algorithm to solve its energy minimization problem. Applying the double level sets model to brain MR images, the experimental results show that the model can accurately and independently segment adjacent tissues in brain. The comparison experimental results with other models show that the double level sets model has a higher accuracy than the classical models in segmenting adjacent tissues. In addition, experiments on synthetic images show that the double level sets model can accurately segment images with intensity inhomogeneity and is robust to noise. Code is avaliable at https://github.com/ruicx/Mutual-Exclusion-Level-Set.
更多查看译文
关键词
Mutual exclusion term,Active contour,Image segmentation,Level set method
AI 理解论文
溯源树
样例
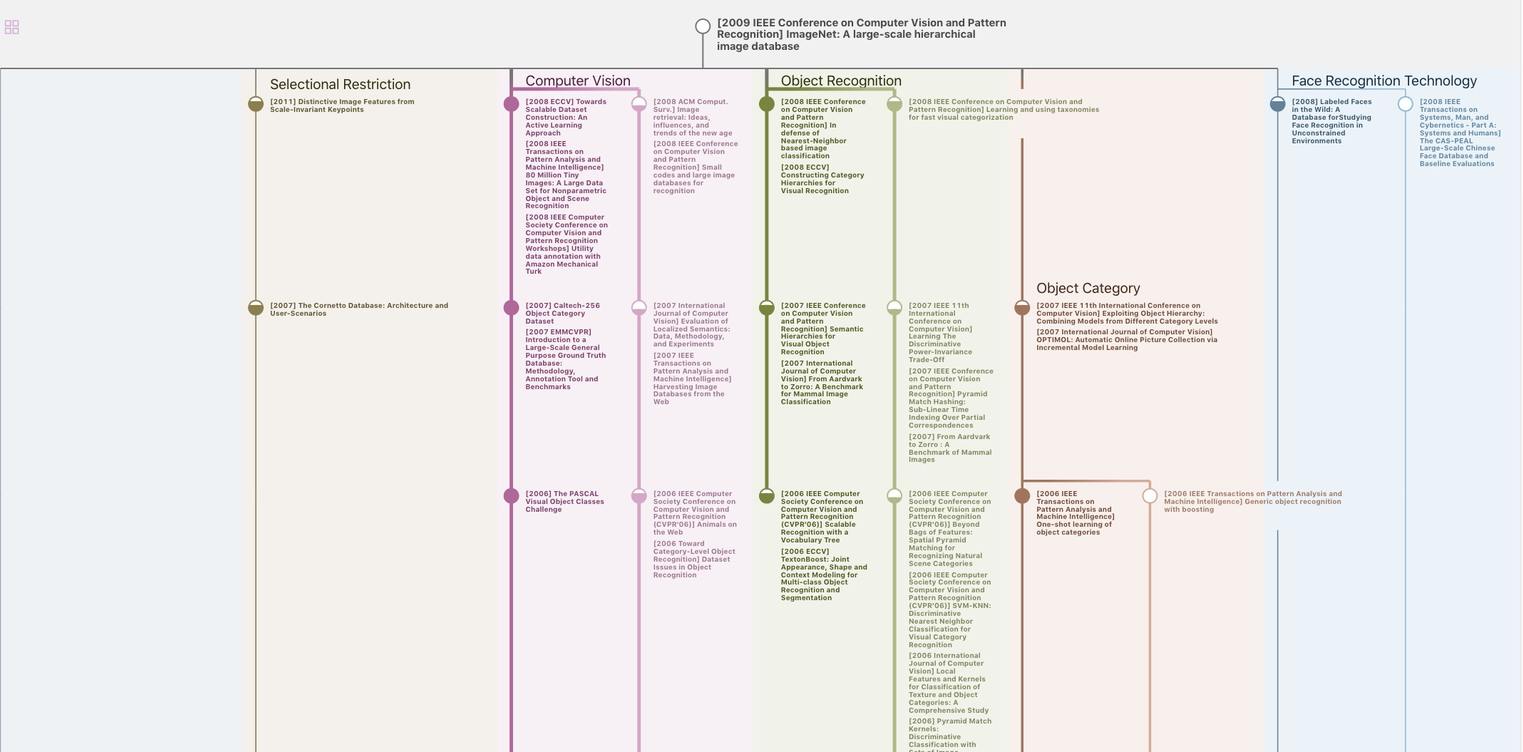
生成溯源树,研究论文发展脉络
Chat Paper
正在生成论文摘要