A Physics-Data-Driven Bayesian Method for Heat Conduction Problems
arXiv (Cornell University)(2021)
摘要
In this study, a novel physics-data-driven Bayesian method named Heat Conduction Equation assisted Bayesian Neural Network (HCE-BNN) is proposed. The HCE-BNN is constructed based on the Bayesian neural network, it is a physics-informed machine learning strategy. Compared with the existed pure data driven method, to acquire physical consistency and better performance of the data-driven model, the heat conduction equation is embedded into the loss function of the HCE-BNN as a regularization term. Hence, the proposed method can build a more reliable model by physical constraints with less data. The HCE-BNN can handle the forward and inverse problems consistently, that is, to infer unknown responses from known partial responses, or to identify boundary conditions or material parameters from known responses. Compared with the exact results, the test results demonstrate that the proposed method can be applied to both heat conduction forward and inverse problems successfully. In addition, the proposed method can be implemented with the noisy data and gives the corresponding uncertainty quantification for the solutions.
更多查看译文
关键词
bayesian method,physics-data-driven
AI 理解论文
溯源树
样例
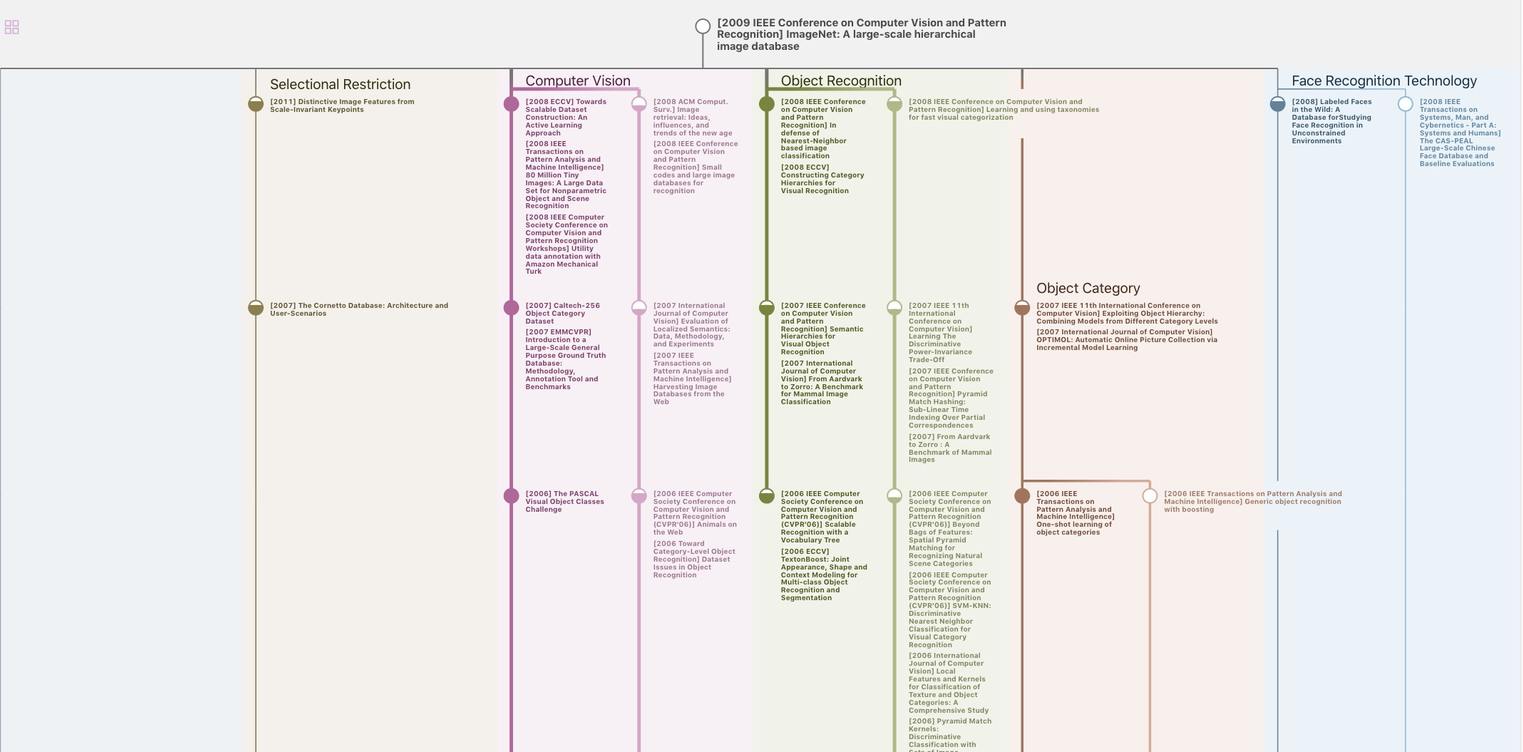
生成溯源树,研究论文发展脉络
Chat Paper
正在生成论文摘要