Reiterative Domain Aware Multi-target Adaptation
PATTERN RECOGNITION, DAGM GCPR 2022(2022)
摘要
Multi-Target Domain Adaptation (MTDA) is a recently popular powerful setting in which a single classifier is learned for multiple unlabeled target domains. A popular MTDA approach is to sequentially adapt one target domain at a time. While only one pass is made through each target domain, the adaptation process for each target domain may consist of many iterations. Inspired by the spaced learning in neuroscience, we instead propose a reiterative approach where we make several passes/reiterations through each target domain. This leads to a better episodic learning that effectively retains features for multiple targets. The reiterative approach does not increase total number of training iterations, as we simply decrease the number of iterations per domain per reiteration. To build a multi-target classifier, it is also important to have a backbone feature extractor that generalizes well across domains. Towards this, we adopt Transformer as a feature extraction backbone. We perform extensive experiments on three popular MTDA datasets: Office-Home, Office-31, and DomainNet, a large-scale dataset. Our experiments separately show the benefits of both reiterative approach and superior Transformer-based feature extractor backbone.
更多查看译文
关键词
Domain adaptation,Multi-domain analysis,Spaced learning,Transformers
AI 理解论文
溯源树
样例
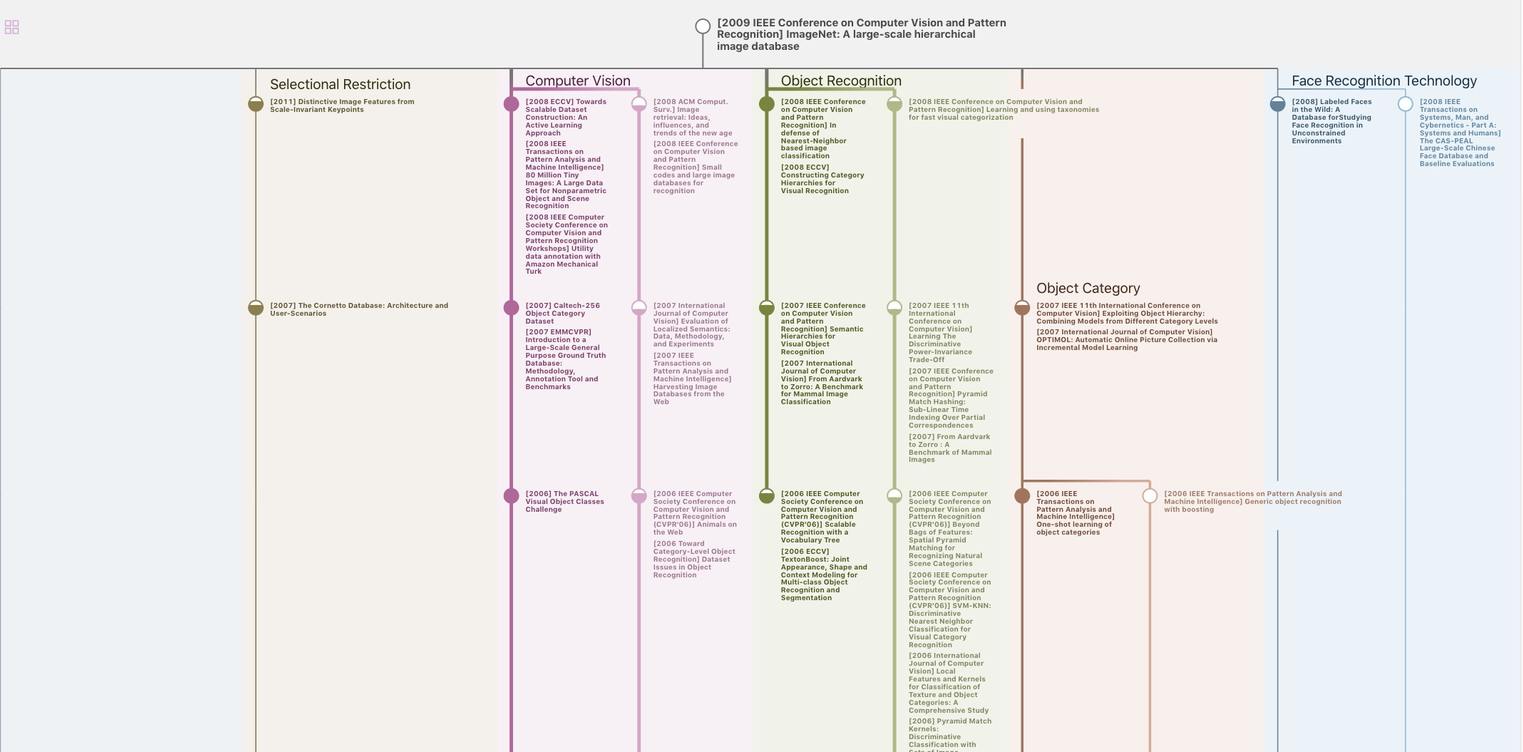
生成溯源树,研究论文发展脉络
Chat Paper
正在生成论文摘要