BiHPF: Bilateral High-Pass Filters for Robust Deepfake Detection
2022 IEEE WINTER CONFERENCE ON APPLICATIONS OF COMPUTER VISION (WACV 2022)(2022)
Abstract
The advancement in numerous generative models has a two fold effect: a simple and easy generation of realistic synthesized images, but also an increased risk of malicious abuse of those images. Thus, it is important to develop a generalized detector for synthesized images of any GAN model or object category, including those unseen during the training phase. However, the conventional methods heavily depend on the training settings, which cause a dramatic decline in performance when tested with unknown domains. To resolve the issue and obtain a generalized detection ability, we propose Bilateral High-Pass Filters (BiHPF), which amplify the effect of the frequency-level artifacts that are generally found in the synthesized images of generative models. Also, to find the properties of the general frequency-level artifacts, we develop an additional method to adversarially extract the artifact compression map. Numerous experimental results validate that our method outperforms other state-of-the-art methods, even when tested with unseen domains.
MoreTranslated text
AI Read Science
Must-Reading Tree
Example
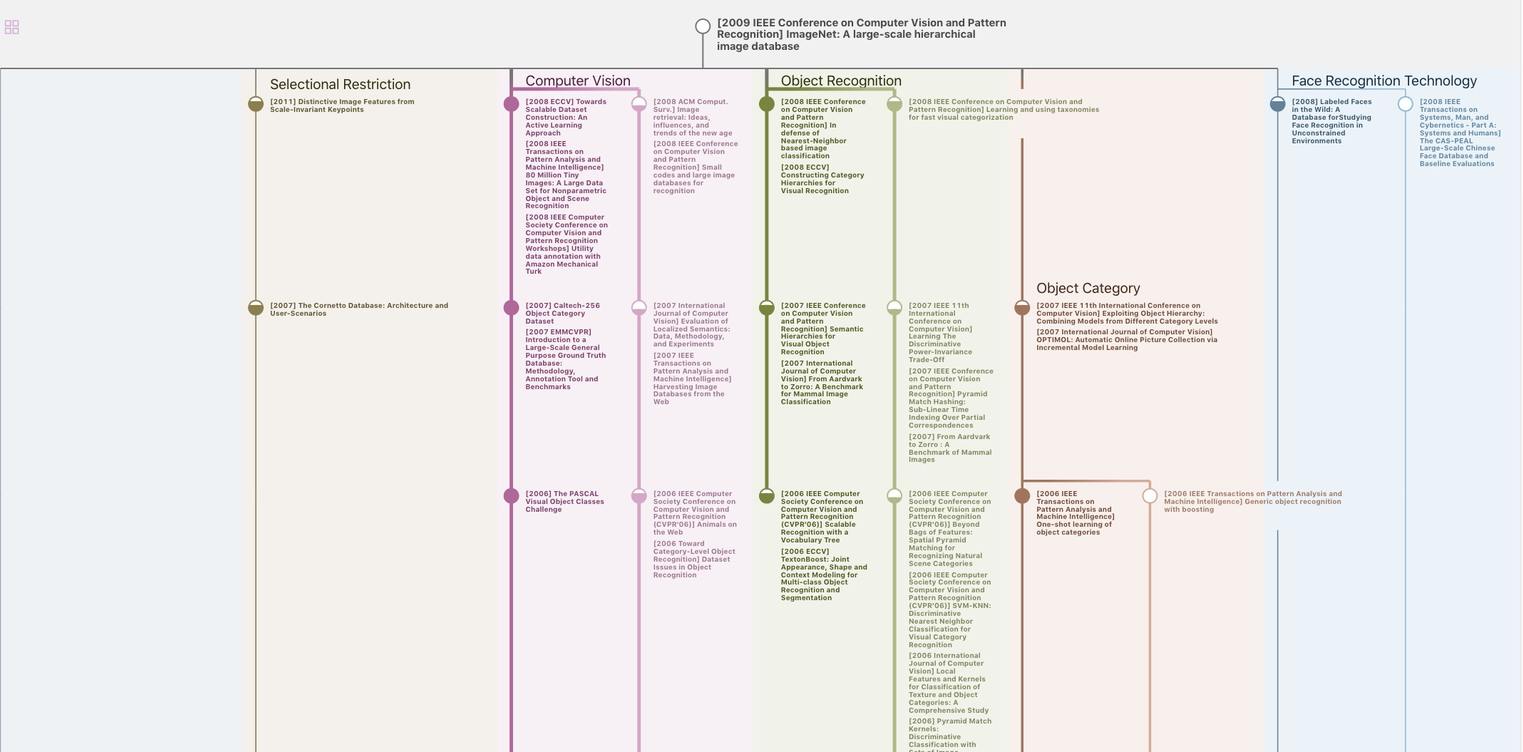
Generate MRT to find the research sequence of this paper
Chat Paper
Summary is being generated by the instructions you defined