Cross-model consensus of explanations and beyond for image classification models: an empirical study
arxiv(2023)
摘要
Existing explanation algorithms have found that, even if deep models make the same correct predictions on the same image, they might rely on different sets of input features for classification. However, among these features, some common features might be used by the majority of models. In this paper, we are wondering what the common features used by various models for classification are and whether the models with better performance may favor those common features . For this purpose, our work uses an explanation algorithm to attribute the importance of features (e.g., pixels or superpixels) as explanations and proposes the cross-model consensus of explanations to capture the common features. Specifically, we first prepare a set of deep models as a committee , then deduce the explanation for every model, and obtain the consensus of explanations across the entire committee through voting . With the cross-model consensus of explanations, we conduct extensive experiments using 80+ models on five datasets/tasks. We find three interesting phenomena as follows: (1) the consensus obtained from image classification models is aligned with the ground truth of semantic segmentation; (2) we measure the similarity of the explanation result of each model in the committee to the consensus (namely consensus score ), and find positive correlations between the consensus score and model performance; and (3) the consensus score potentially correlates to the interpretability.
更多查看译文
关键词
Interpretability,Explanations of deep neural networks,Semantic segmentation,and Visualization
AI 理解论文
溯源树
样例
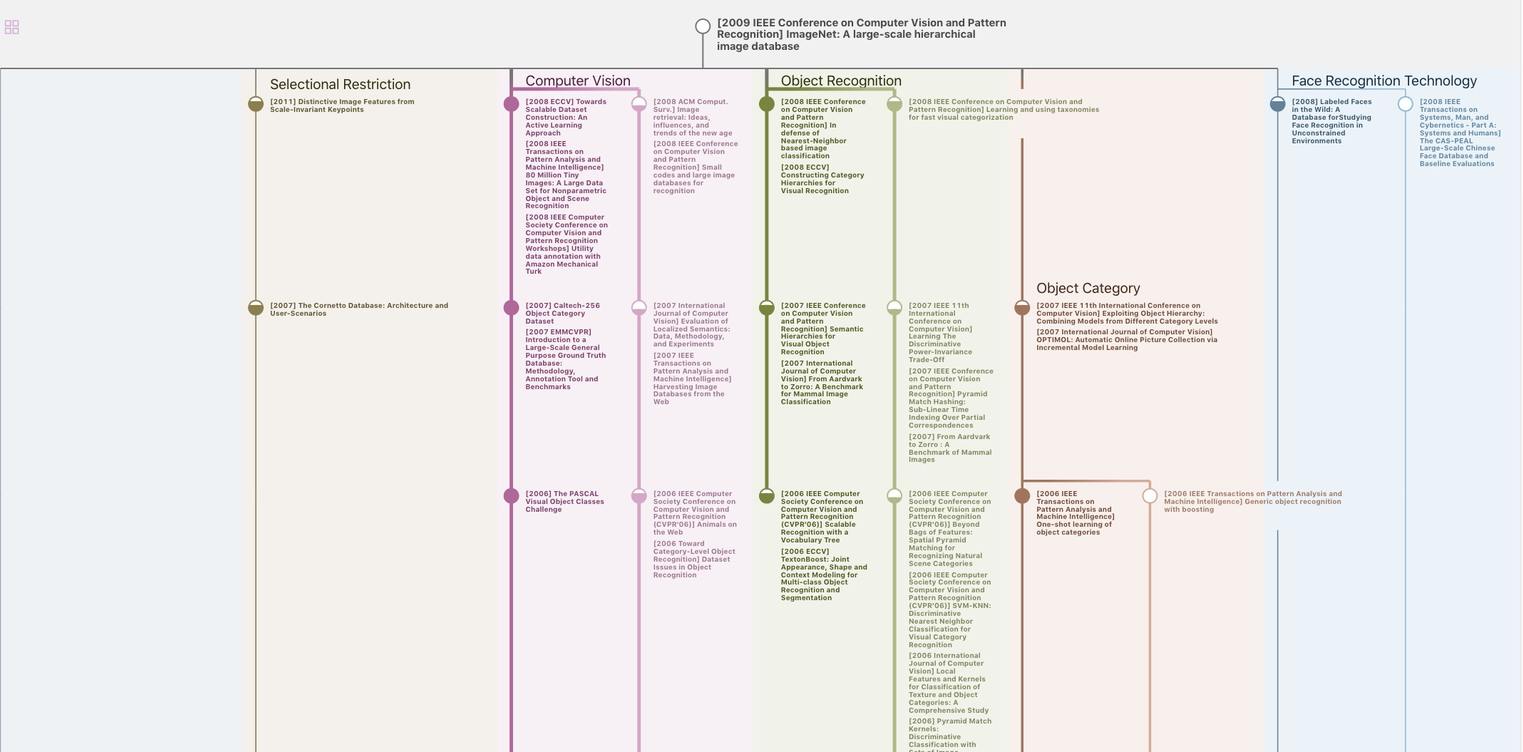
生成溯源树,研究论文发展脉络
Chat Paper
正在生成论文摘要