ALPS: Adaptive Quantization of Deep Neural Networks with GeneraLized PositS
2021 IEEE/CVF CONFERENCE ON COMPUTER VISION AND PATTERN RECOGITION WORKSHOPS (CVPRW 2021)(2021)
摘要
In this paper, a new adaptive quantization algorithm for generalized posit format is presented, to optimally represent the dynamic range and distribution of deep neural network parameters. Adaptation is achieved by minimizing the intra-layer posit quantization error with a compander. The efficacy of the proposed quantization algorithm is studied within a new low-precision framework, ALPS, on ResNet-50 and EfficientNet models for classification tasks. Results assert that the accuracy and energy dissipation of low-precision DNNs using generalized posits outperform other well-known numerical formats, including standard posits.
更多查看译文
关键词
standard posits,deep neural networks,generalized posits,adaptive quantization algorithm,generalized posit format,dynamic range,deep neural network parameters,low-precision framework,ALPS,numerical formats,low-precision DNN,intralayer posit quantization error,ResNet-50,EfficientNet models,classification tasks
AI 理解论文
溯源树
样例
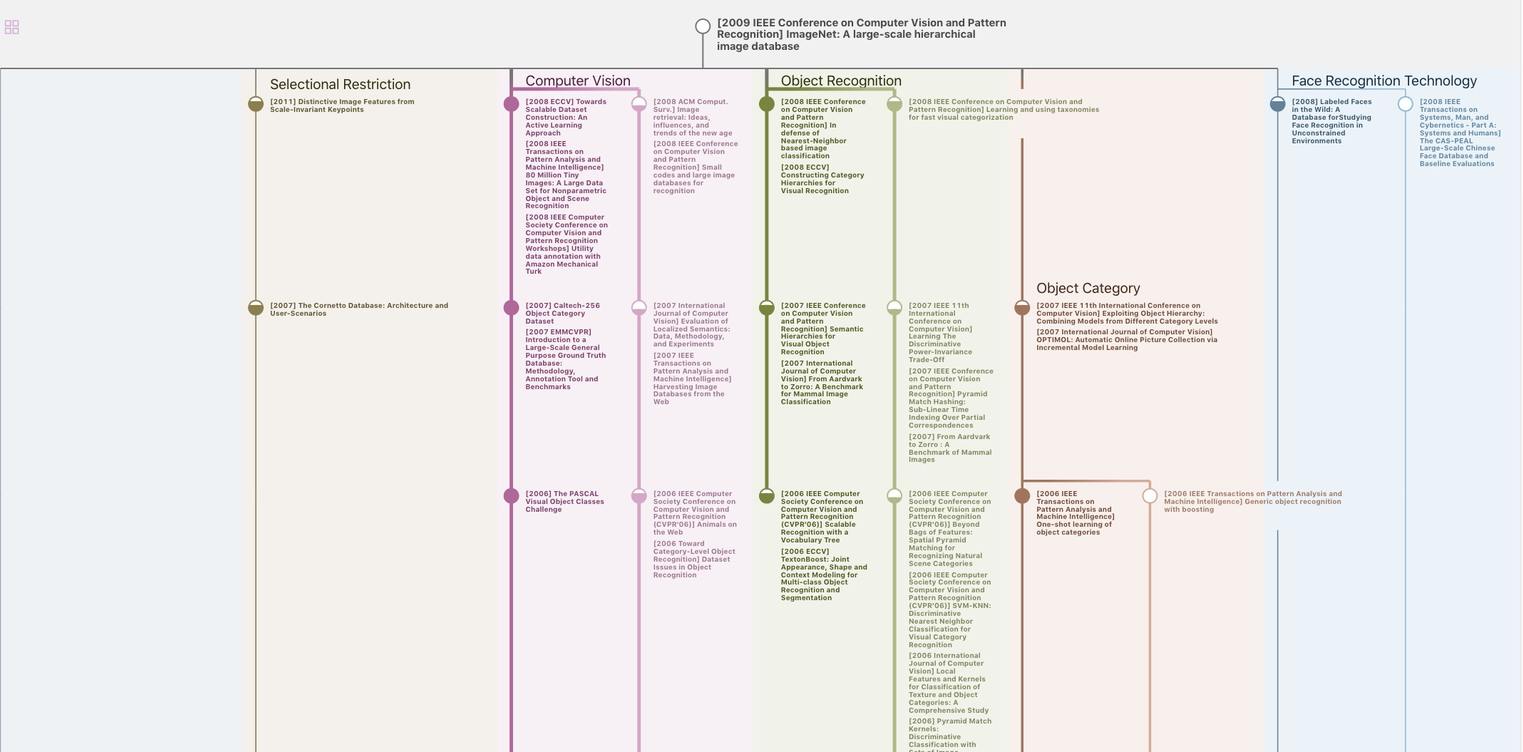
生成溯源树,研究论文发展脉络
Chat Paper
正在生成论文摘要