v2e: From Video Frames to Realistic DVS Events
2021 IEEE/CVF CONFERENCE ON COMPUTER VISION AND PATTERN RECOGITION WORKSHOPS (CVPRW 2021)(2021)
摘要
To help meet the increasing need for dynamic vision sensor (DVS) event camera data, this paper proposes the v2e toolbox that generates realistic synthetic DVS events from intensity frames. It also clarifies incorrect claims about DVS motion blur and latency characteristics in recent literature. Unlike other toolboxes, v2e includes pixel-level Gaussian event threshold mismatch, finite intensity-dependent bandwidth, and intensity-dependent noise. Realistic DVS events are useful in training networks for uncontrolled lighting conditions. The use of v2e synthetic events is demonstrated in two experiments. The first experiment is object recognition with N-Caltech 101 dataset. Results show that pretraining on various v2e lighting conditions improves generalization when transferred on real DVS data for a ResNet model. The second experiment shows that for night driving, a car detector trained with v2e events shows an average accuracy improvement of 40% compared to the YOLOv3 trained on intensity frames.
更多查看译文
关键词
latency characteristics,DVS event camera data,N-Caltech 101 dataset,finite intensity-dependent bandwidth,ResNet model,v2e lighting conditions,v2e synthetic events,intensity-dependent noise,pixel-level Gaussian event threshold mismatch,DVS motion blur,intensity frames,realistic synthetic DVS events,dynamic vision sensor event camera data,realistic DVS events,video frames
AI 理解论文
溯源树
样例
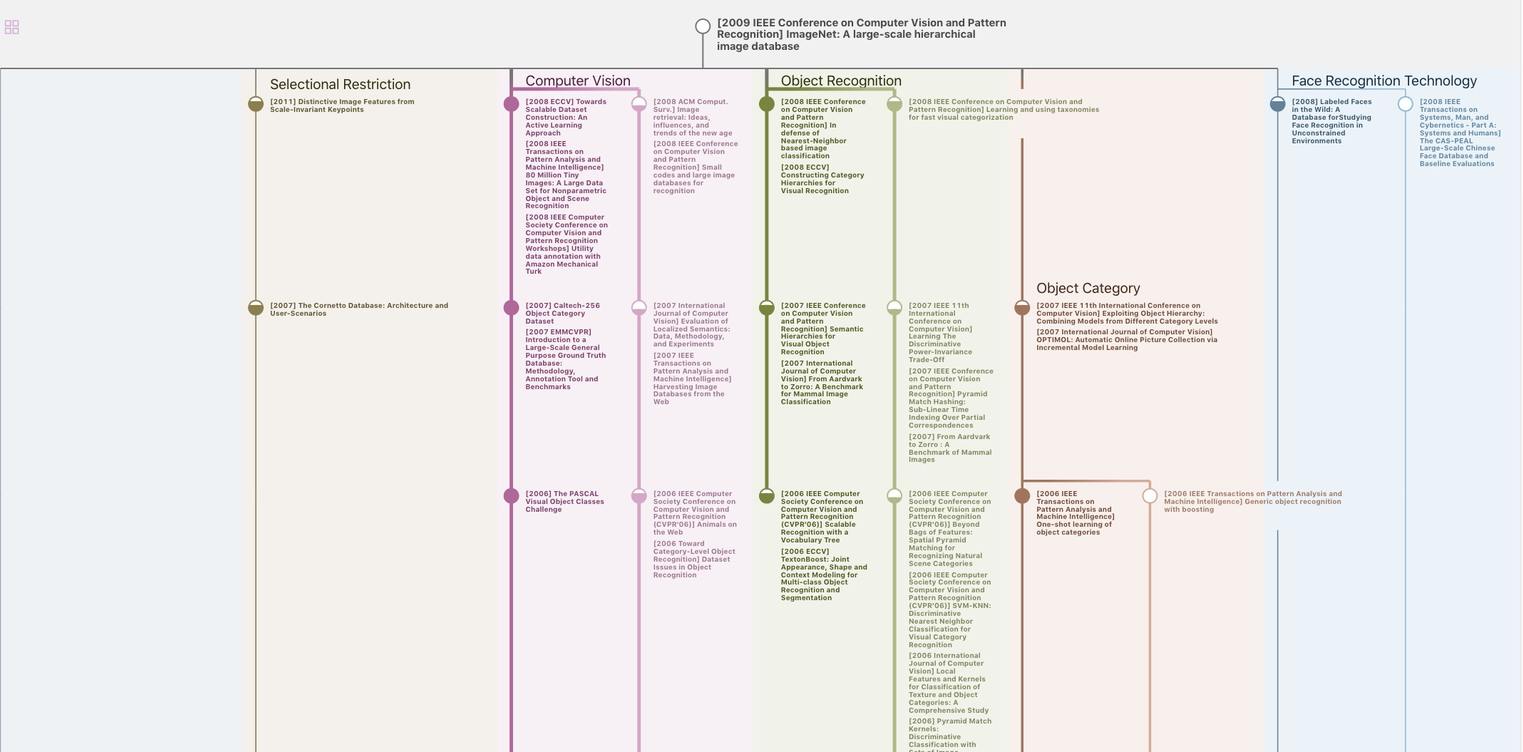
生成溯源树,研究论文发展脉络
Chat Paper
正在生成论文摘要