ConRPG: Paraphrase Generation using Contexts as Regularizer
EMNLP(2021)
Abstract
A long-standing issue with paraphrase generation is how to obtain reliable supervision signals. In this paper, we propose an unsupervised paradigm for paraphrase generation based on the assumption that the probabilities of generating two sentences with the same meaning given the same context should be the same. Inspired by this fundamental idea, we propose a pipelined system which consists of paraphrase candidate generation based on contextual language models, candidate filtering using scoring functions, and paraphrase model training based on the selected candidates. The proposed paradigm offers merits over existing paraphrase generation methods: (1) using the context regularizer on meanings, the model is able to generate massive amounts of high-quality paraphrase pairs; and (2) using human-interpretable scoring functions to select paraphrase pairs from candidates, the proposed framework provides a channel for developers to intervene with the data generation process, leading to a more controllable model. Experimental results across different tasks and datasets demonstrate that the effectiveness of the proposed model in both supervised and unsupervised setups.
MoreTranslated text
Key words
paraphrase generation,contexts
AI Read Science
Must-Reading Tree
Example
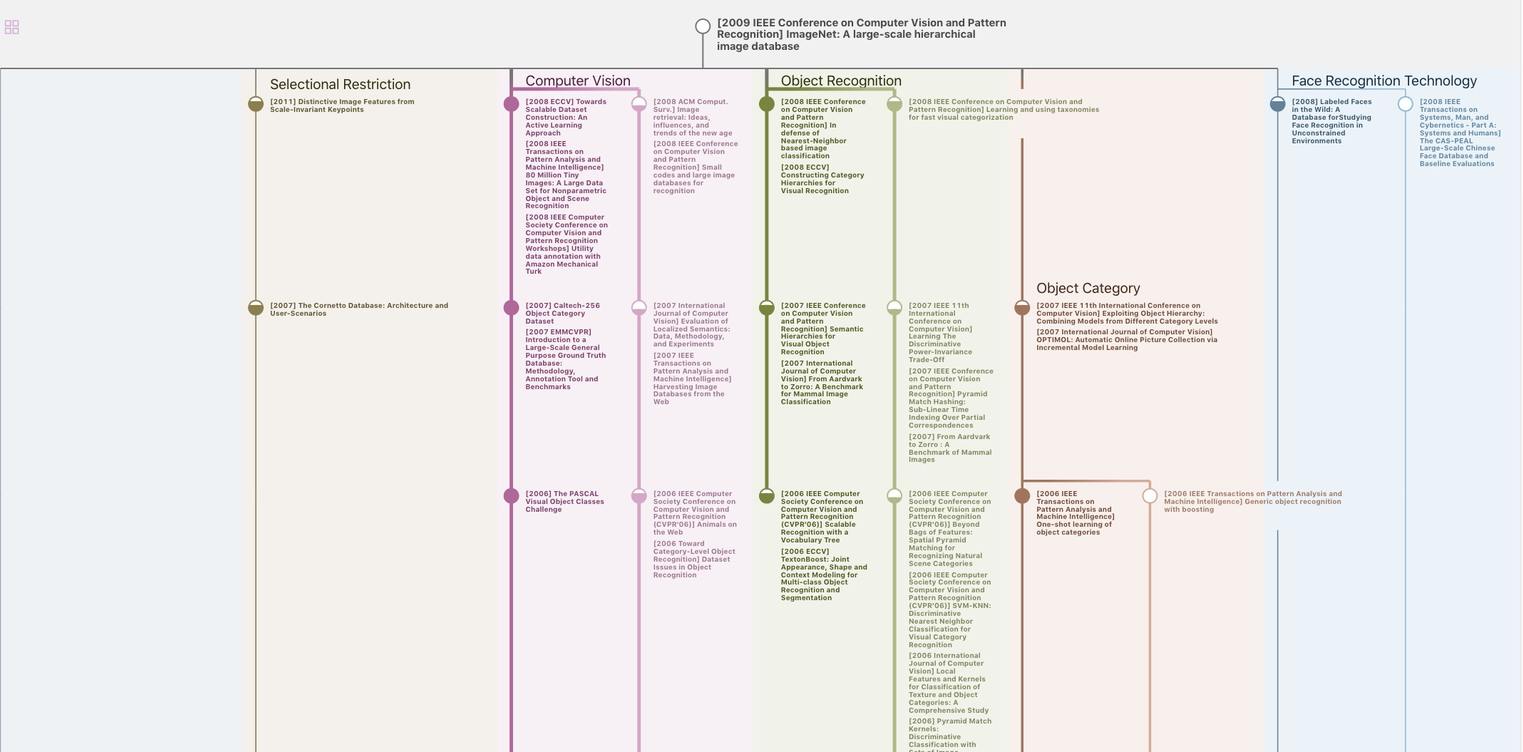
Generate MRT to find the research sequence of this paper
Chat Paper
Summary is being generated by the instructions you defined