Unsupervised Reconstruction of Sea Surface Currents from AIS Maritime Traffic Data Using Trainable Variational Models
REMOTE SENSING(2021)
Abstract
The estimation of ocean dynamics is a key challenge for applications ranging from climate modeling to ship routing. State-of-the-art methods relying on satellite-derived altimetry data can hardly resolve spatial scales below similar to 100 km. In this work we investigate the relevance of AIS data streams as a new mean for the estimation of the surface current velocities. Using a physics-informed observation model, we propose to solve the associated the ill-posed inverse problem using a trainable variational formulation. The latter exploits variational auto-encoders coupled with neural ODE to represent sea surface dynamics. We report numerical experiments on a real AIS dataset off South Africa in a highly dynamical ocean region. They support the relevance of the proposed learning-based AIS-driven approach to significantly improve the reconstruction of sea surface currents compared with state-of-the-art methods, including altimetry-based ones.
MoreTranslated text
Key words
AIS,inverse problems,data assimilation,deep learning,neural ODEs
AI Read Science
Must-Reading Tree
Example
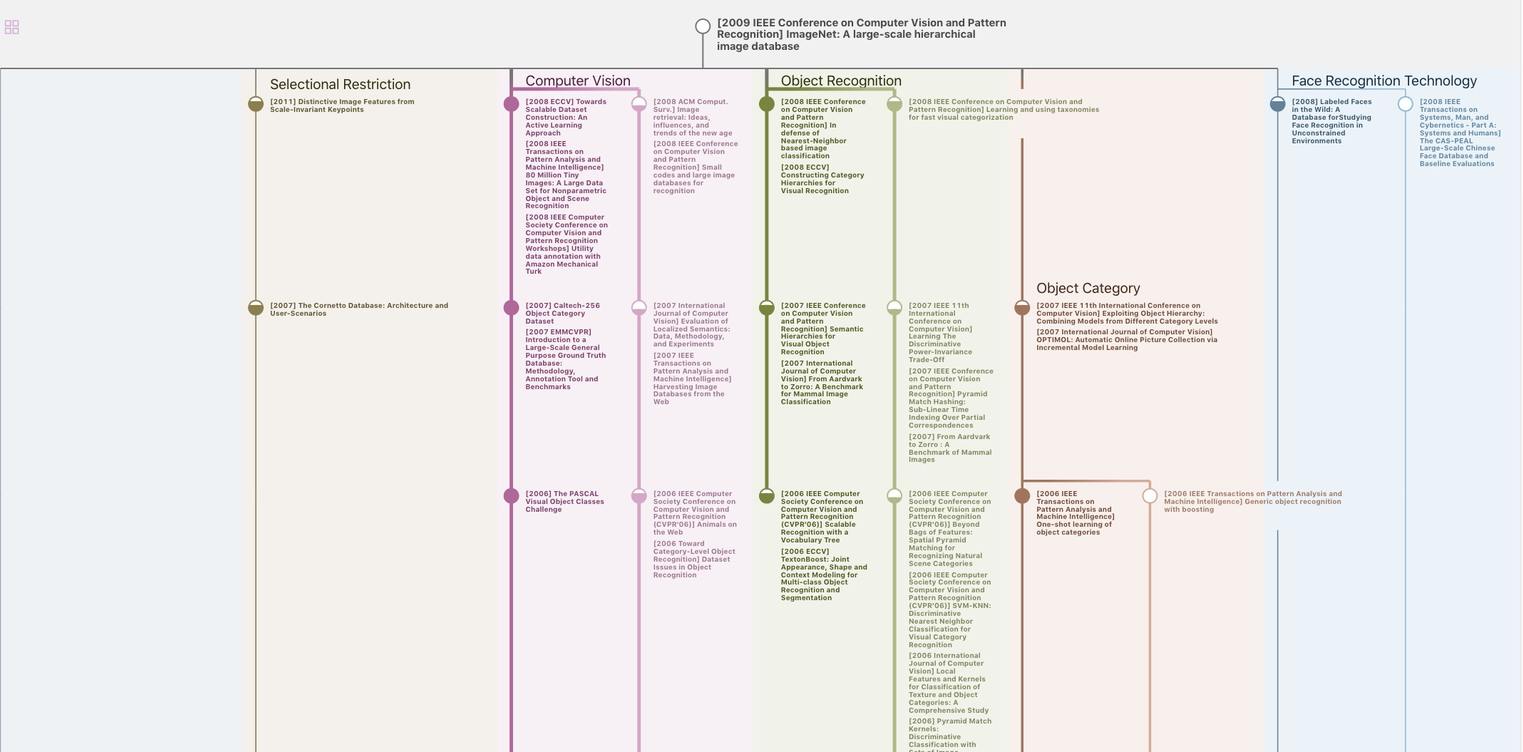
Generate MRT to find the research sequence of this paper
Chat Paper
Summary is being generated by the instructions you defined