UNMAS: Multiagent Reinforcement Learning for Unshaped Cooperative Scenarios
IEEE transactions on neural networks and learning systems(2023)
摘要
Multiagent reinforcement learning methods, such as VDN, QMIX, and QTRAN, that adopt centralized training with decentralized execution (CTDE) framework have shown promising results in cooperation and competition. However, in some multiagent scenarios, the number of agents and the size of the action set actually vary over time. We call these unshaped scenarios, and the methods mentioned above fail in performing satisfyingly. In this article, we propose a new method, called Unshaped Networks for Multiagent Systems (UNMAS), which adapts to the number and size changes in multiagent systems. We propose the self-weighting mixing network to factorize the joint action-value. Its adaption to the change in agent number is attributed to the nonlinear mapping from each-agent Q value to the joint action-value with individual weights. Besides, in order to address the change in an action set, each agent constructs an individual action-value network that is composed of two streams to evaluate the constant environment-oriented subset and the varying unit-oriented subset. We evaluate UNMAS on various StarCraft II micromanagement scenarios and compare the results with several state-of-the-art MARL algorithms. The superiority of UNMAS is demonstrated by its highest winning rates especially on the most difficult scenario 3s5z_vs_3s6z. The agents learn to perform effectively cooperative behaviors, while other MARL algorithms fail. Animated demonstrations and source code are provided in https://sites.google.com/view/unmas.
更多查看译文
关键词
Multi-agent systems,Training,Task analysis,Reinforcement learning,Sun,Learning systems,Semantics,Centralized training with decentralized execution (CTDE),multiagent,reinforcement learning,StarCraft II
AI 理解论文
溯源树
样例
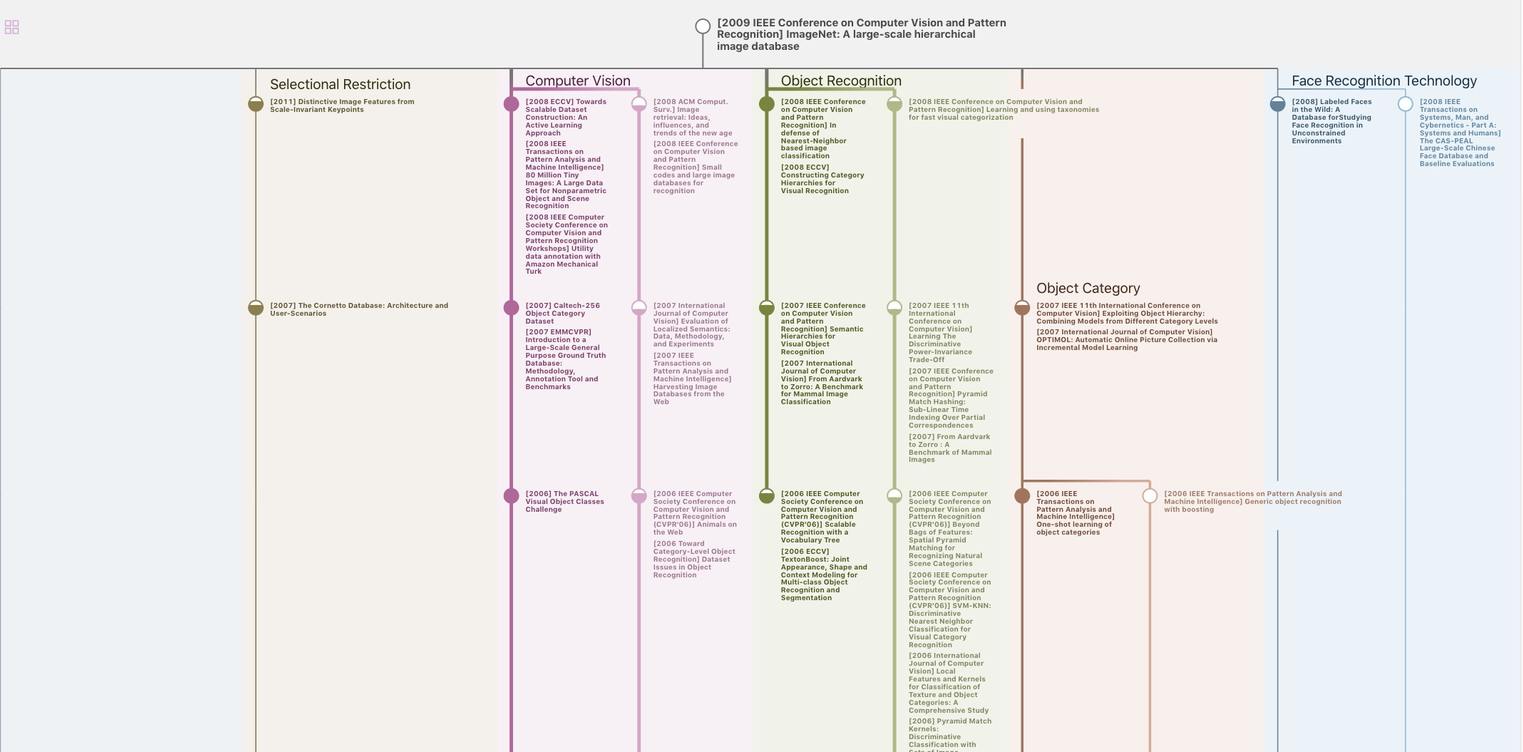
生成溯源树,研究论文发展脉络
Chat Paper
正在生成论文摘要