Noisy Labels for Weakly Supervised Gamma Hadron Classification
arxiv(2021)
摘要
Gamma hadron classification, a central machine learning task in gamma ray astronomy, is conventionally tackled with supervised learning. However, the supervised approach requires annotated training data to be produced in sophisticated and costly simulations. We propose to instead solve gamma hadron classification with a noisy label approach that only uses unlabeled data recorded by the real telescope. To this end, we employ the significance of detection as a learning criterion which addresses this form of weak supervision. We show that models which are based on the significance of detection deliver state-of-the-art results, despite being exclusively trained with noisy labels; put differently, our models do not require the costly simulated ground-truth labels that astronomers otherwise employ for classifier training. Our weakly supervised models exhibit competitive performances also on imbalanced data sets that stem from a variety of other application domains. In contrast to existing work on class-conditional label noise, we assume that only one of the class-wise noise rates is known.
更多查看译文
AI 理解论文
溯源树
样例
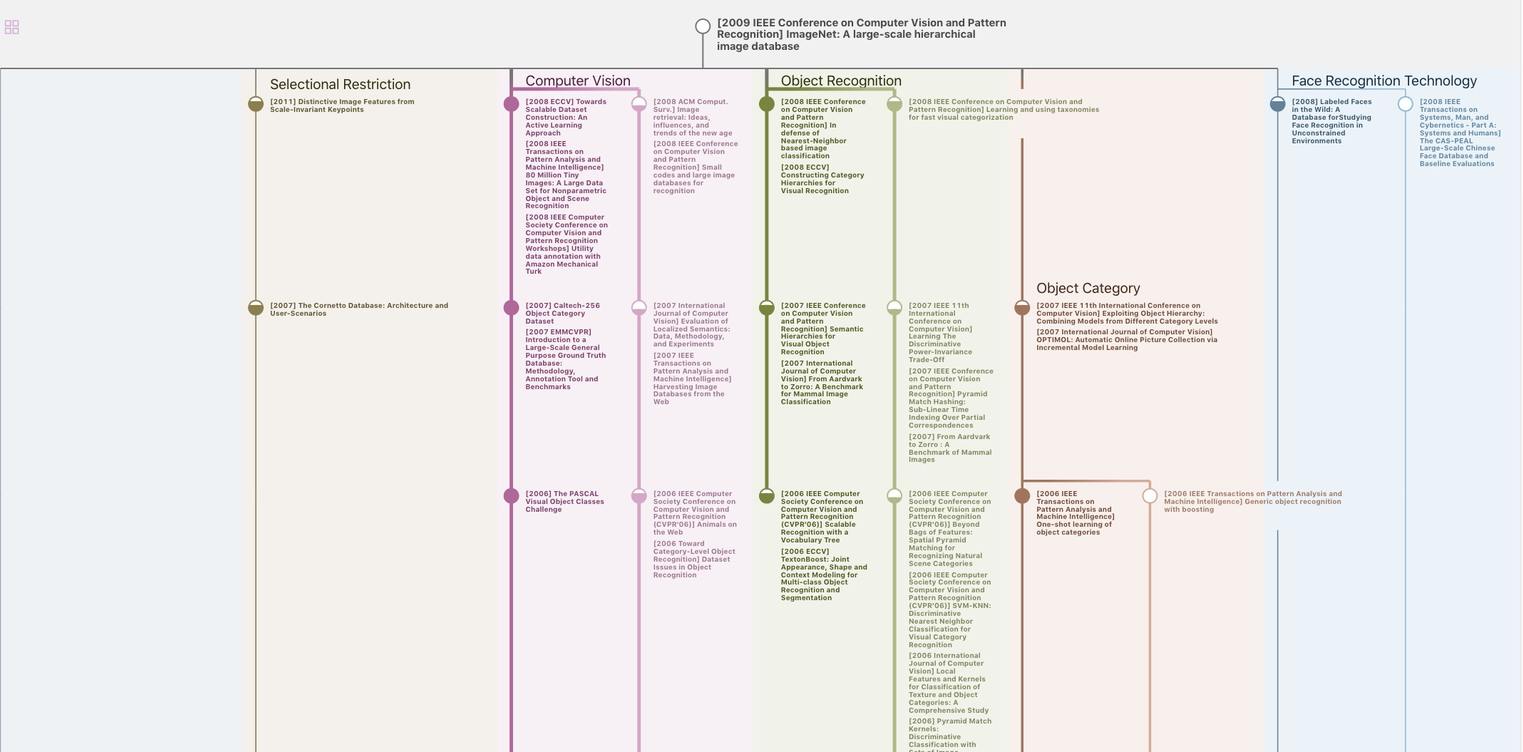
生成溯源树,研究论文发展脉络
Chat Paper
正在生成论文摘要