Incremental Learning for Personalized Recommender Systems
arxiv(2021)
摘要
Ubiquitous personalized recommender systems are built to achieve two seemingly conflicting goals, to serve high quality content tailored to individual user's taste and to adapt quickly to the ever changing environment. The former requires a complex machine learning model that is trained on a large amount of data; the latter requires frequent update to the model. We present an incremental learning solution to provide both the training efficiency and the model quality. Our solution is based on sequential Bayesian update and quadratic approximation. Our focus is on large-scale personalized logistic regression models, with extensions to deep learning models. This paper fills in the gap between the theory and the practice by addressing a few implementation challenges that arise when applying incremental learning to large personalized recommender systems. Detailed offline and online experiments demonstrated our approach can significantly shorten the training time while maintaining the model accuracy. The solution is deployed in LinkedIn and directly applicable to industrial scale recommender systems.
更多查看译文
关键词
personalized recommender systems,learning
AI 理解论文
溯源树
样例
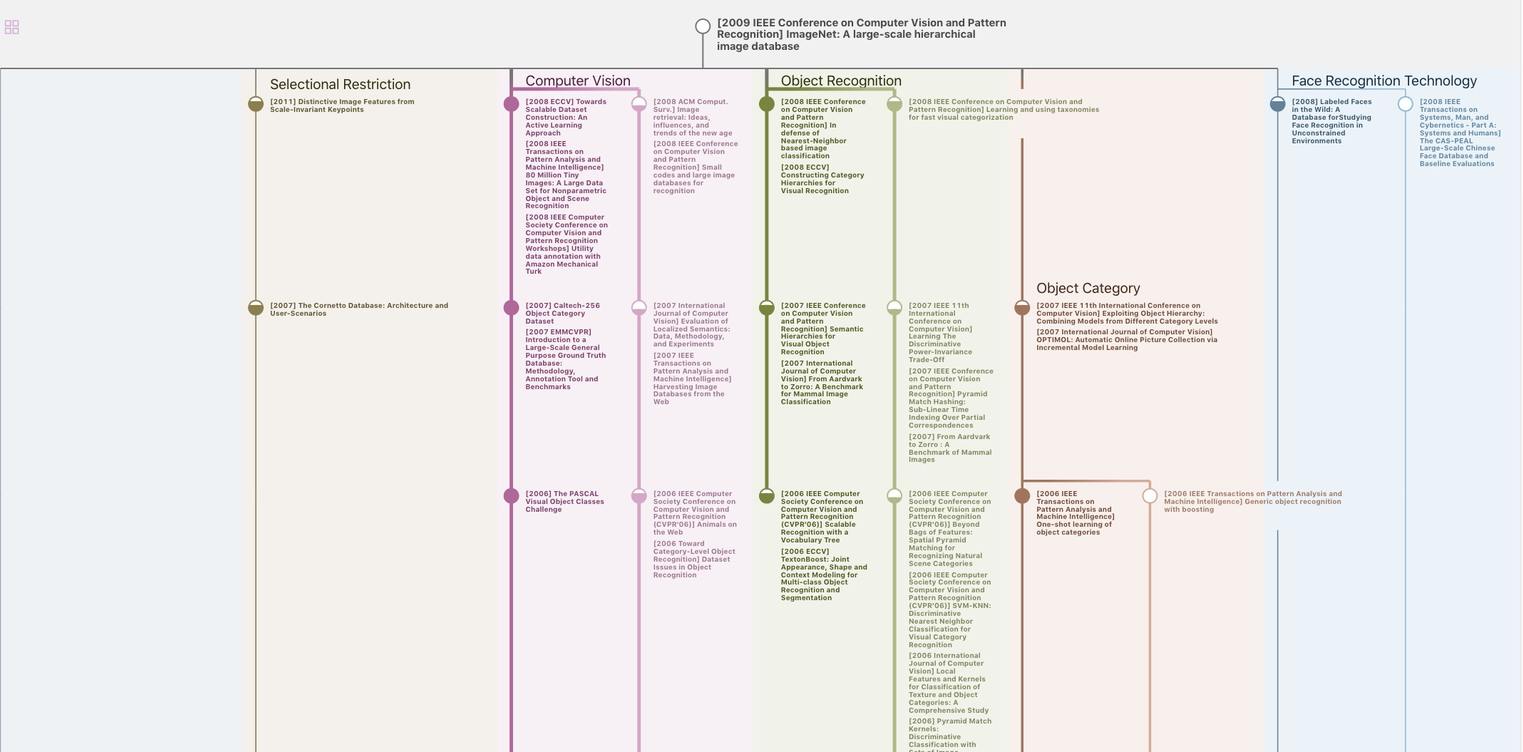
生成溯源树,研究论文发展脉络
Chat Paper
正在生成论文摘要