Tune It or Don't Use It: Benchmarking Data-Efficient Image Classification
2021 IEEE/CVF INTERNATIONAL CONFERENCE ON COMPUTER VISION WORKSHOPS (ICCVW 2021)(2021)
摘要
Data-efficient image classification using deep neural networks in settings, where only small amounts of labeled data are available, has been an active research area in the recent past. However; an objective comparison between published methods is difficult, since existing works use different datasets for evaluation and often compare against untuned baselines with default hyper-parameters. We design a benchmark for data-efficient image classification consisting of six diverse datasets spanning various domains (e.g., natural images, medical imagery, satellite data) and data types (RGB, grayscale, multispectral). Using this benchmark, we re-evaluate the standard cross-entropy baseline and eight methods for data-efficient deep learning published between 2017 and 2021 at renowned venues. For a fair and realistic comparison, we carefully tune the hyper parameters of all methods on each dataset. Surprisingly, we find that tuning learning rate, weight decay, and batch size on a separate validation split results in a highly competitive baseline, which outperforms all but one specialized method and performs competitively to the remaining one.
更多查看译文
关键词
data-efficient image classification,satellite data,data-efficient deep learning,diverse datasets,data types,tuning learning rate,weight decay,batch size
AI 理解论文
溯源树
样例
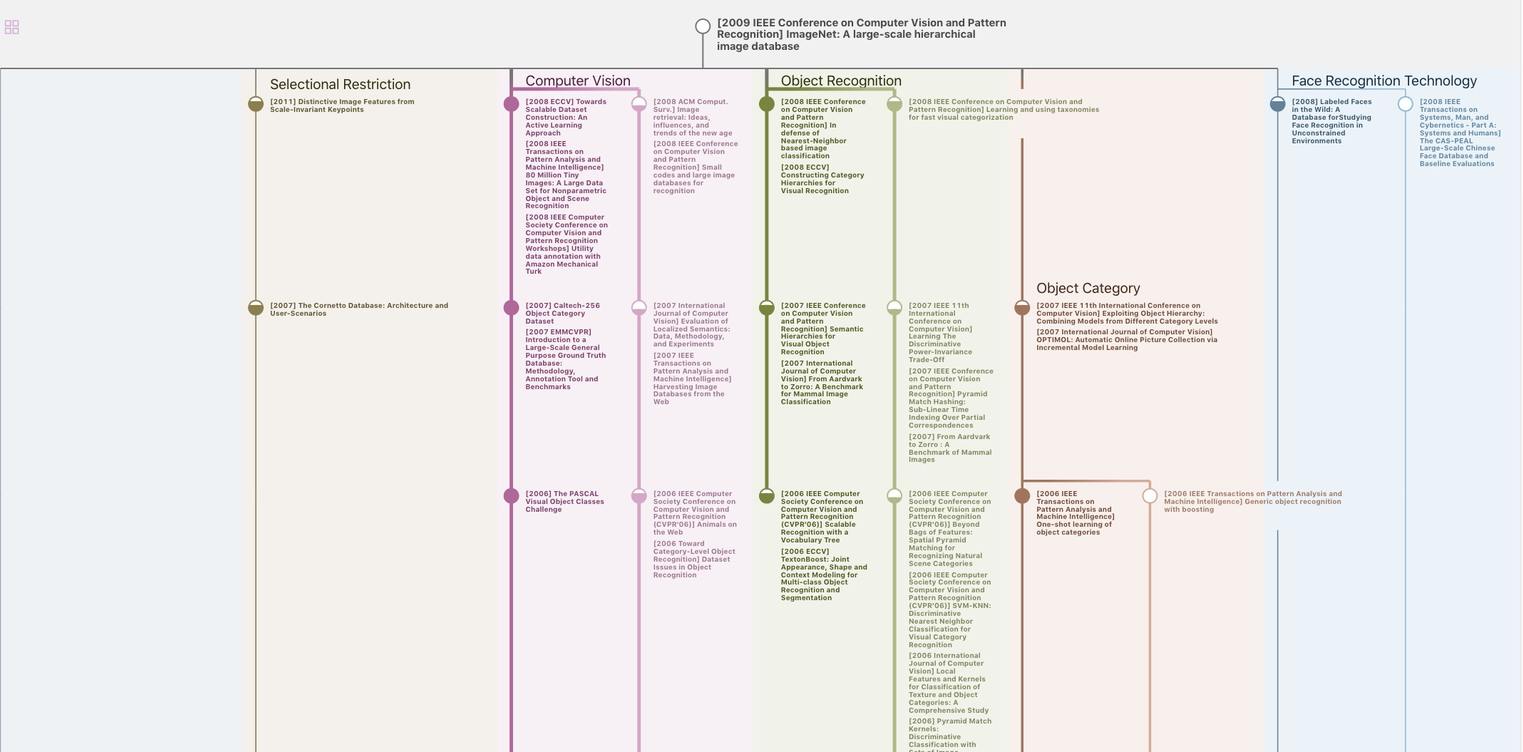
生成溯源树,研究论文发展脉络
Chat Paper
正在生成论文摘要