Wasserstein generative adversarial uncertainty quantification in physics-informed neural networks
Journal of Computational Physics(2022)
摘要
In this paper, we study a physics-informed algorithm for Wasserstein Generative Adversarial Networks (WGANs) for uncertainty quantification in solutions of partial differential equations. By using groupsort activation functions in adversarial network discriminators, network generators are utilized to learn the uncertainty in solutions of partial differential equations observed from the initial/boundary data. Under mild assumptions, we show that the generalization error of the computed generator converges to the approximation error of the network with high probability, when the number of samples are sufficiently taken. According to our established error bound, we also find that our physics-informed WGANs have higher requirement for the capacity of discriminators than that of generators. Numerical results on synthetic examples of partial differential equations are reported to validate our theoretical results and demonstrate how uncertainty quantification can be obtained for solutions of partial differential equations and the distributions of initial/boundary data. However, the quality or the accuracy of the uncertainty quantification theory in all the points in the interior is still the theoretical vacancy, and required for further research.
更多查看译文
关键词
Wasserstein generative adversarial networks,Simi-supervised learning,Data-driven scientific computing,Machine learning,Uncertainty quantifications,Generalization theory
AI 理解论文
溯源树
样例
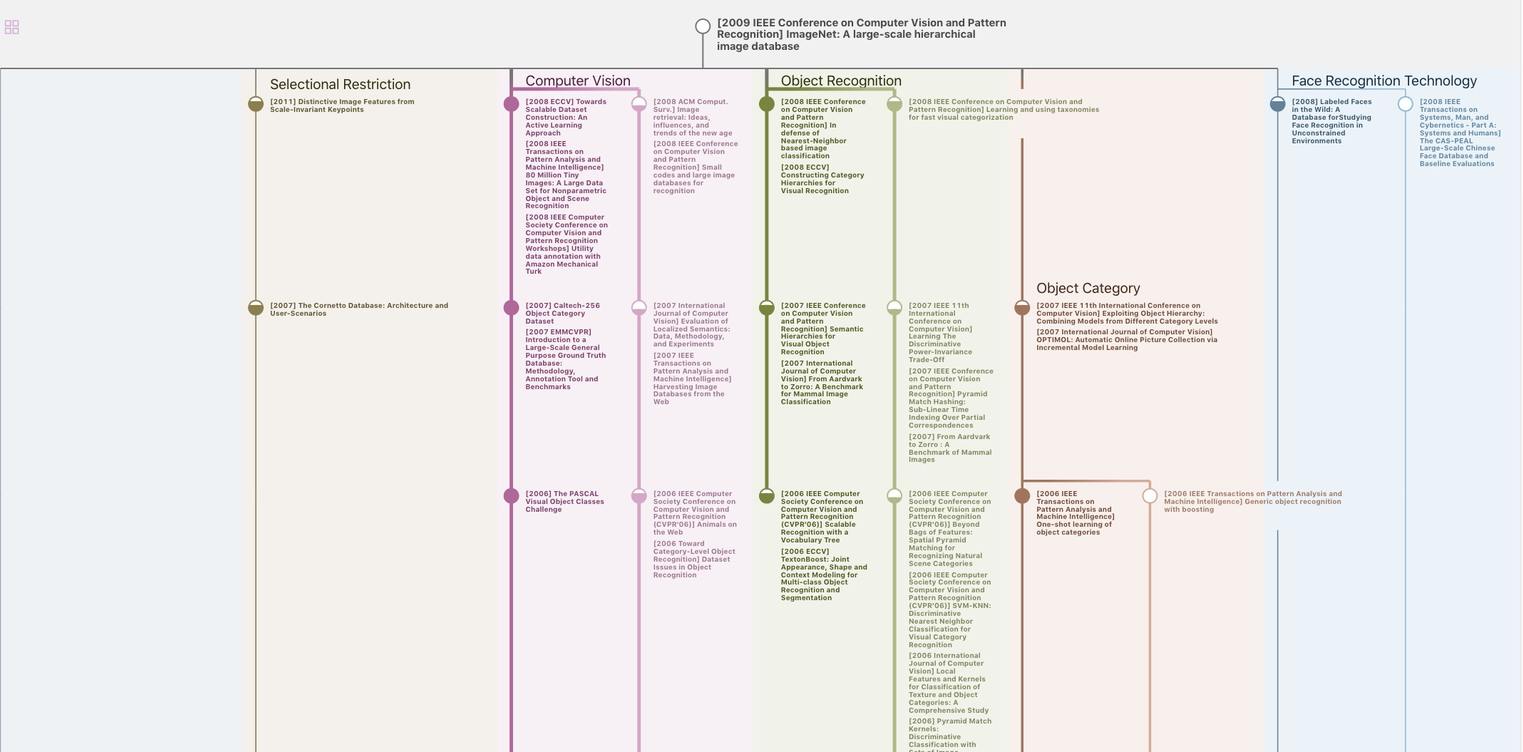
生成溯源树,研究论文发展脉络
Chat Paper
正在生成论文摘要