Translation Error Detection as Rationale Extraction
FINDINGS OF THE ASSOCIATION FOR COMPUTATIONAL LINGUISTICS (ACL 2022)(2022)
Abstract
Recent Quality Estimation (QE) models based on multilingual pre-trained representations have achieved very competitive results in predicting the overall quality of translated sentences. However, detecting specifically which translated words are incorrect is a more challenging task, especially when dealing with limited amounts of training data. We hypothesize that, not unlike humans, successful QE models rely on translation errors to predict overall sentence quality. By exploring a set of feature attribution methods that assign relevance scores to the inputs to explain model predictions, we study the behaviour of state-of-theart sentence-level QE models and show that explanations (i.e. rationales) extracted from these models can indeed be used to detect translation errors. We therefore (i) introduce a novel semi-supervised method for word-level QE; and (ii) propose to use the QE task as a new benchmark for evaluating the plausibility of feature attribution, i.e. how interpretable model explanations are to humans.
MoreTranslated text
Key words
rationale extraction,translation
AI Read Science
Must-Reading Tree
Example
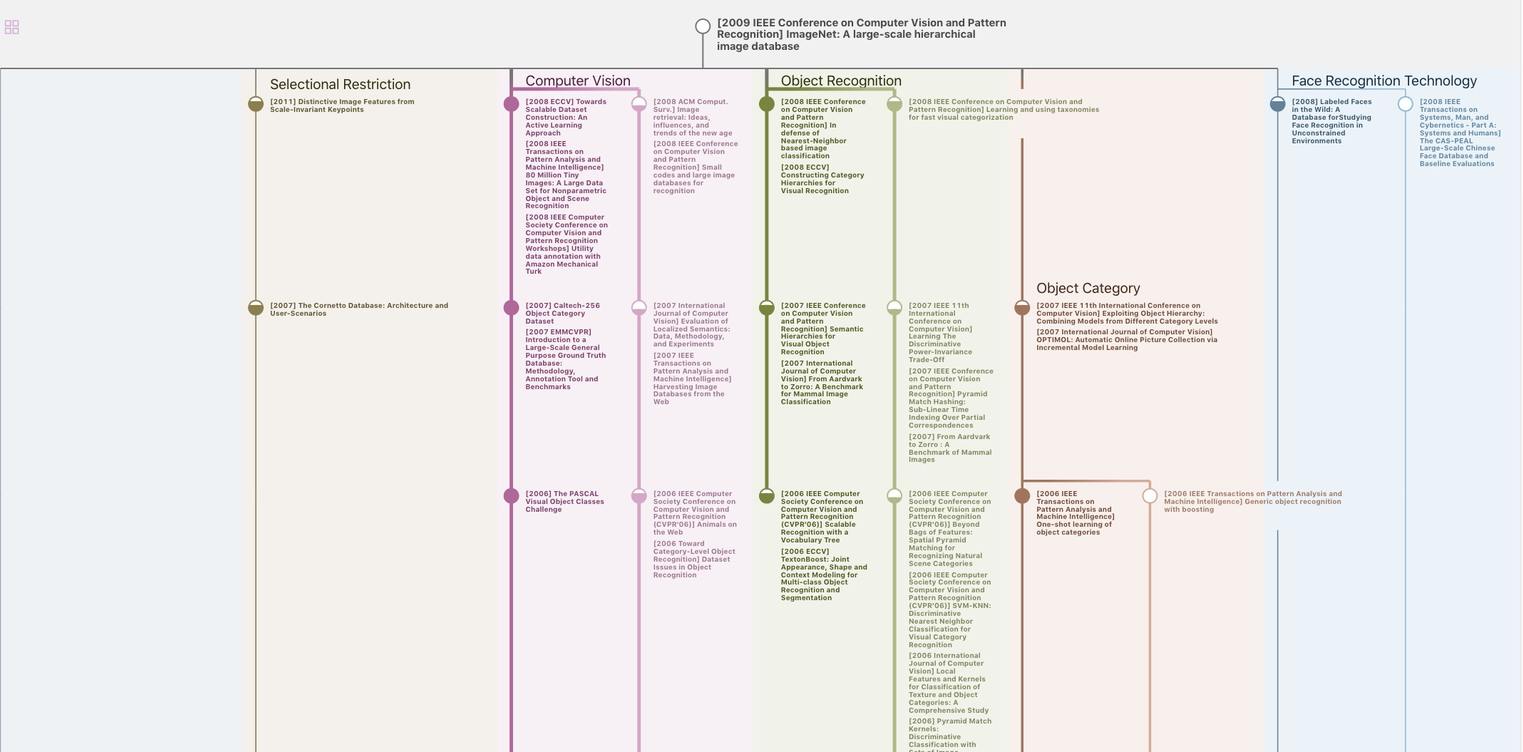
Generate MRT to find the research sequence of this paper
Chat Paper
Summary is being generated by the instructions you defined