Lossless AI: Toward Guaranteeing Consistency between Inferences Before and After Quantization via Knowledge Distillation
2021 17th International Conference on Machine Vision and Applications (MVA)(2021)
摘要
Deep learning model compression is necessary for real-time inference on edge devices, which have limited hardware resources. Conventional methods have only focused on suppressing degradation in terms of accuracy. Even if a compressed model has almost equivalent accuracy to its reference model, the inference results may change when we focus on individual samples or objects. Such a change is a crucial challenge for the quality assurance of embedded products because of unexpected behavior for specific applications on edge devices. Therefore, we propose a concept called “Loss-less AI” to guarantee consistency between the inference results of reference and compressed models. In this paper, we propose a training method to align inference results between reference and quantized models by applying knowledge distillation that batch normalization statistics are frozen at moving average values from the middle of training. We evaluated the proposed method on several classification datasets and network architectures. In all cases, our method suppressed the inferred class mismatch between reference and quantized models whereas conventional quantization-aware training did not.
更多查看译文
关键词
lossless AI,knowledge distillation,deep learning model compression,real-time inference,edge devices,degradation suppression,reference model,training method,quantized models,conventional quantization-aware training,embedded product quality assurance,batch normalization statistics,classification datasets,network architecture,inferred class mismatch suppression
AI 理解论文
溯源树
样例
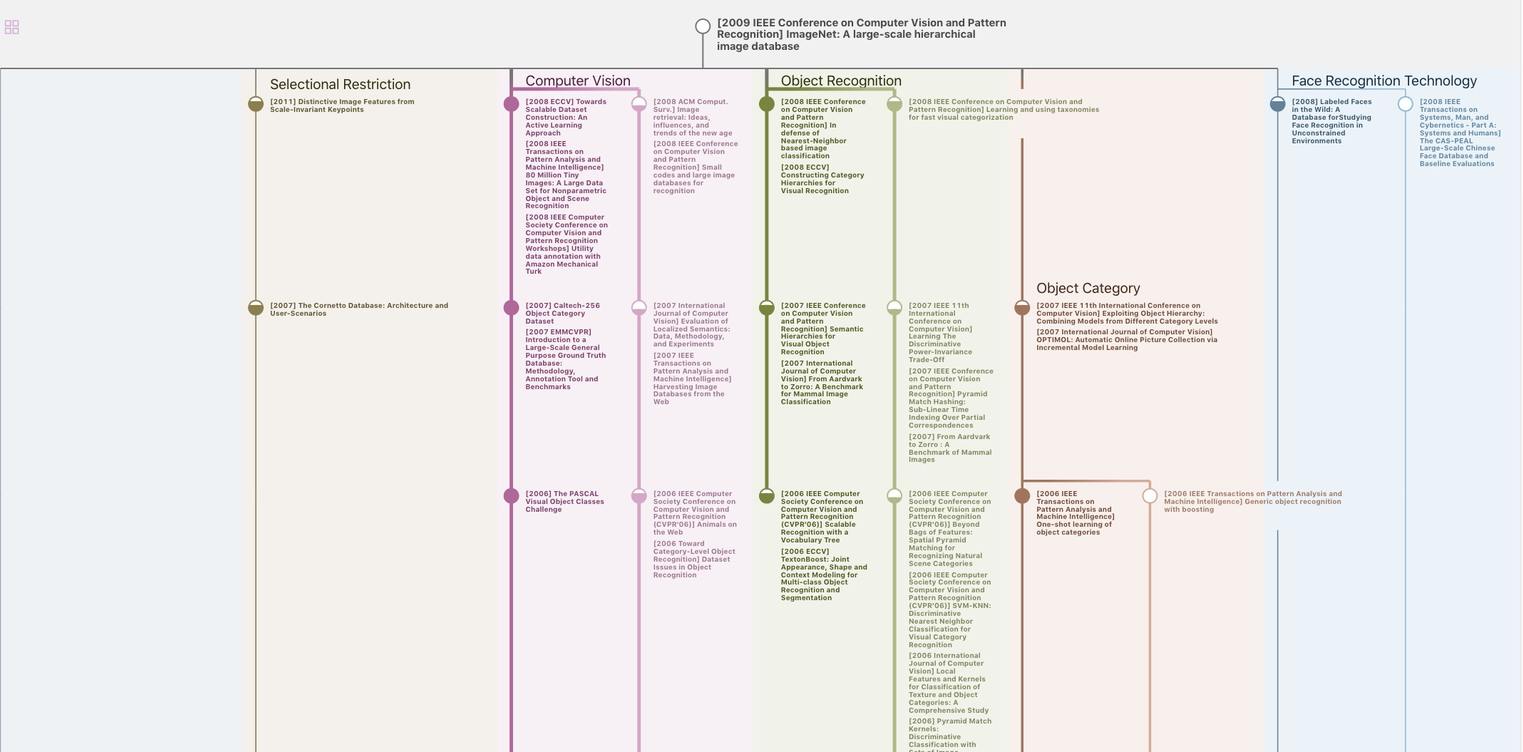
生成溯源树,研究论文发展脉络
Chat Paper
正在生成论文摘要