Change Detection in The Covariance Structure of High-Dimensional Gaussian Low-Rank Models
2021 IEEE Statistical Signal Processing Workshop (SSP)(2021)
摘要
This paper is devoted to the problem of testing equality between the covariance matrices of L multivariate Gaussian time series with dimension M, in the context where each of the L covariance matrices is the sum of a low-rank K component and the identity matrix. Assuming N
1
, …, N
L
samples are available for each time series, a new test statistic, based on the eigenvalues of the L sample covariance matrices (SCM) of each time series as well as the eigenvalues of a pooled SCM mixing the N
1
+…+N
L
available samples, is pro-posed and proved to be consistent in the high dimensional regime in which M, N
1
, …, N
L
converge to infinity at the same rate, while K and L are kept fixed. Numerical simulations show that the proposed test statistic is competitive with other relevant methods for moderate values of M, N
1
, …, N
L
.
更多查看译文
关键词
Change detection,covariance,spiked models,random matrix theory
AI 理解论文
溯源树
样例
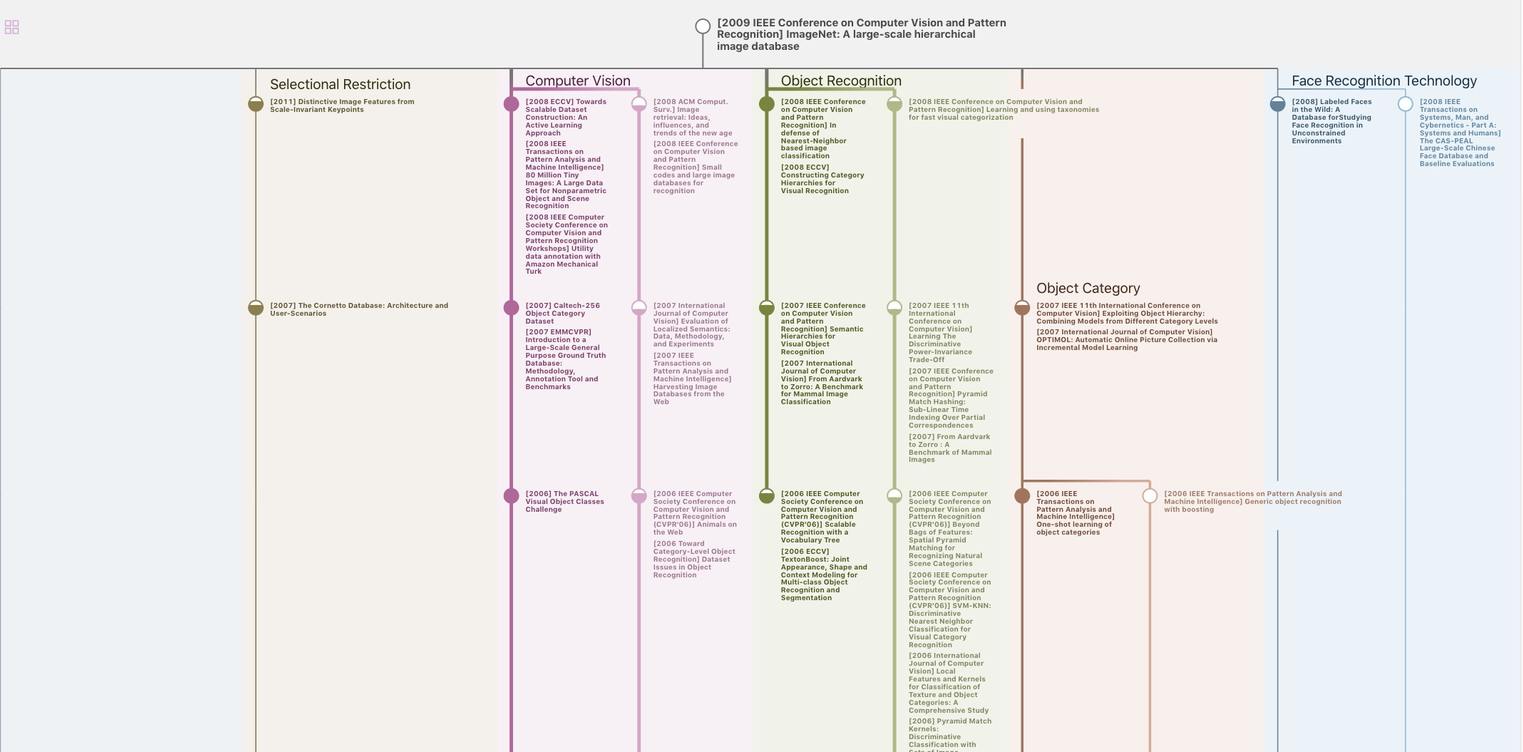
生成溯源树,研究论文发展脉络
Chat Paper
正在生成论文摘要