Self-supervised deep metric learning for ancient papyrus fragments retrieval
INTERNATIONAL JOURNAL ON DOCUMENT ANALYSIS AND RECOGNITION(2021)
摘要
This work focuses on document fragments association using deep metric learning methods. More precisely, we are interested in ancient papyri fragments that need to be reconstructed prior to their analysis by papyrologists. This is a challenging task to automatize using machine learning algorithms because labeled data is rare, often incomplete, imbalanced and of inconsistent conservation states. However, there is a real need for such software in the papyrology community as the process of reconstructing the papyri by hand is extremely time-consuming and tedious. In this paper, we explore ways in which papyrologists can obtain useful matching suggestion on new data using Deep Convolutional Siamese-Networks . We emphasize on low-to-no human intervention for annotating images. We show that the from-scratch self-supervised approach we propose is more effective than using knowledge transfer from a large dataset, the former achieving a top-1 accuracy score of 0.73 on a retrieval task involving 800 fragments.
更多查看译文
关键词
Ancient document reconstruction, Deep metric learning, Information retrieval, Self-supervised learning, Domain adaptation
AI 理解论文
溯源树
样例
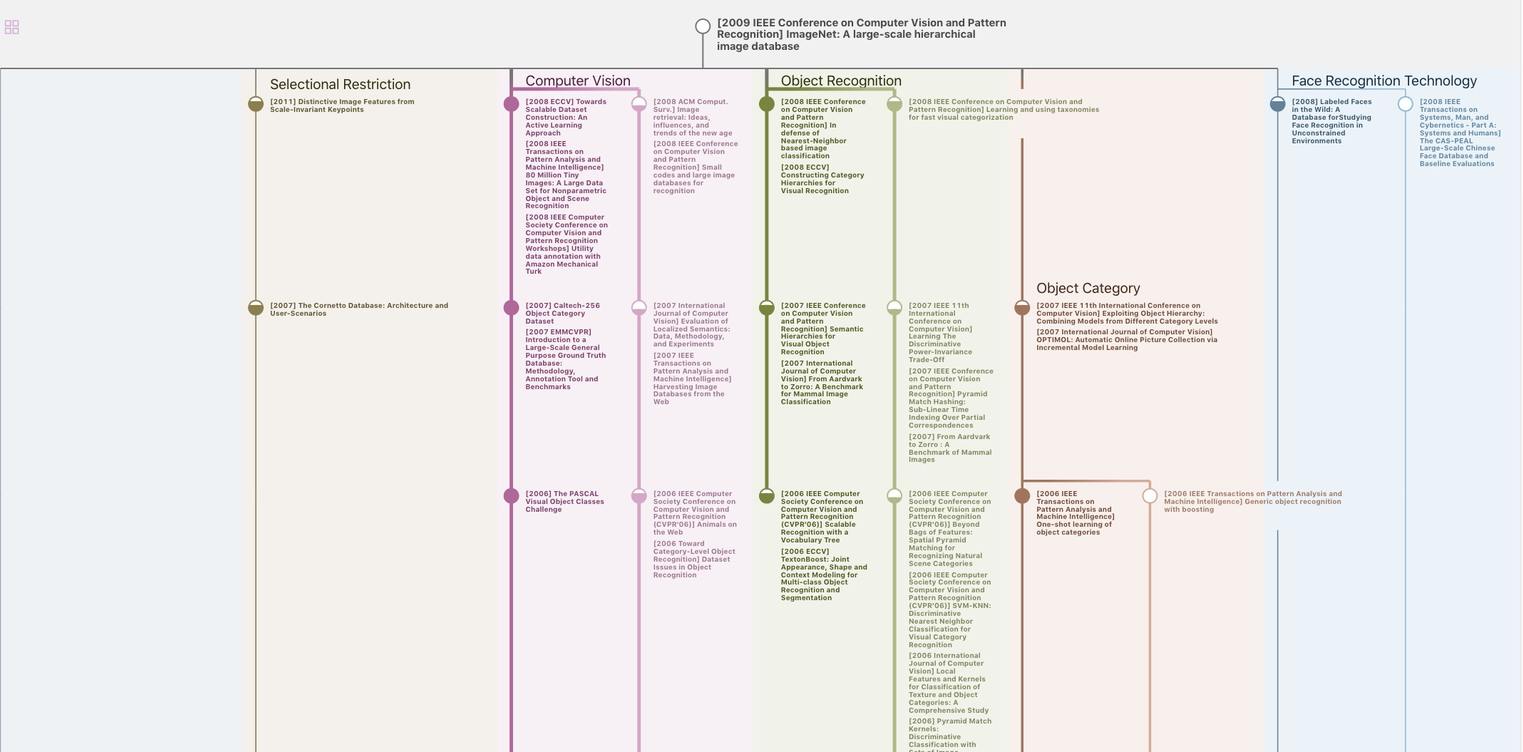
生成溯源树,研究论文发展脉络
Chat Paper
正在生成论文摘要