Textual analysis of traitor-based dataset through semi supervised machine learning
Future Generation Computer Systems(2021)
摘要
Insider threats are one of the most challenging and growing security threats which the government agencies, organizations, and institutions face. In such scenarios, malicious (red) activities are performed by the authorized individuals within the company. Because of which, an insider threat has become a taxing and difficult task to identify among other attacks. Along with other monitoring parameters; email logs play a vital role in many research areas such as stalking Insider Threat involving Collaborating Traitors, Textual Analysis, and Social Media exploration. This paper presents a semi-supervised machine learning framework which embraces the pre-processing and classification techniques together for unlabeled dataset i.e. emails. Enron Corporation dataset has been used for experiments and TWOS for evaluation of the proposed framework. Initially, dataset is transformed into vector form using Term Frequency–Inverse Document Frequency (TF–IDF). Thereafter, K-Means is used to classify emails based on message content. Finally, Machine Learning algorithm Decision Tree (DT) is applied to classify the malicious activities. The proposed framework has also been tested with other algorithms such as Logistic Regression (LR), Naive Bayes (NB), KNN, Support Vector Machine (SVM), Random Forest (RF) and Neural Network (NN). However, Decision Tree (DT) combined with pre-processing steps has given the desired results with 99.96% Accuracy and 0.994 AUC for identification of malicious content.
更多查看译文
关键词
Malicious emails,Insider threat,Machine learning,Enron dataset,TWOS dataset,Text classification
AI 理解论文
溯源树
样例
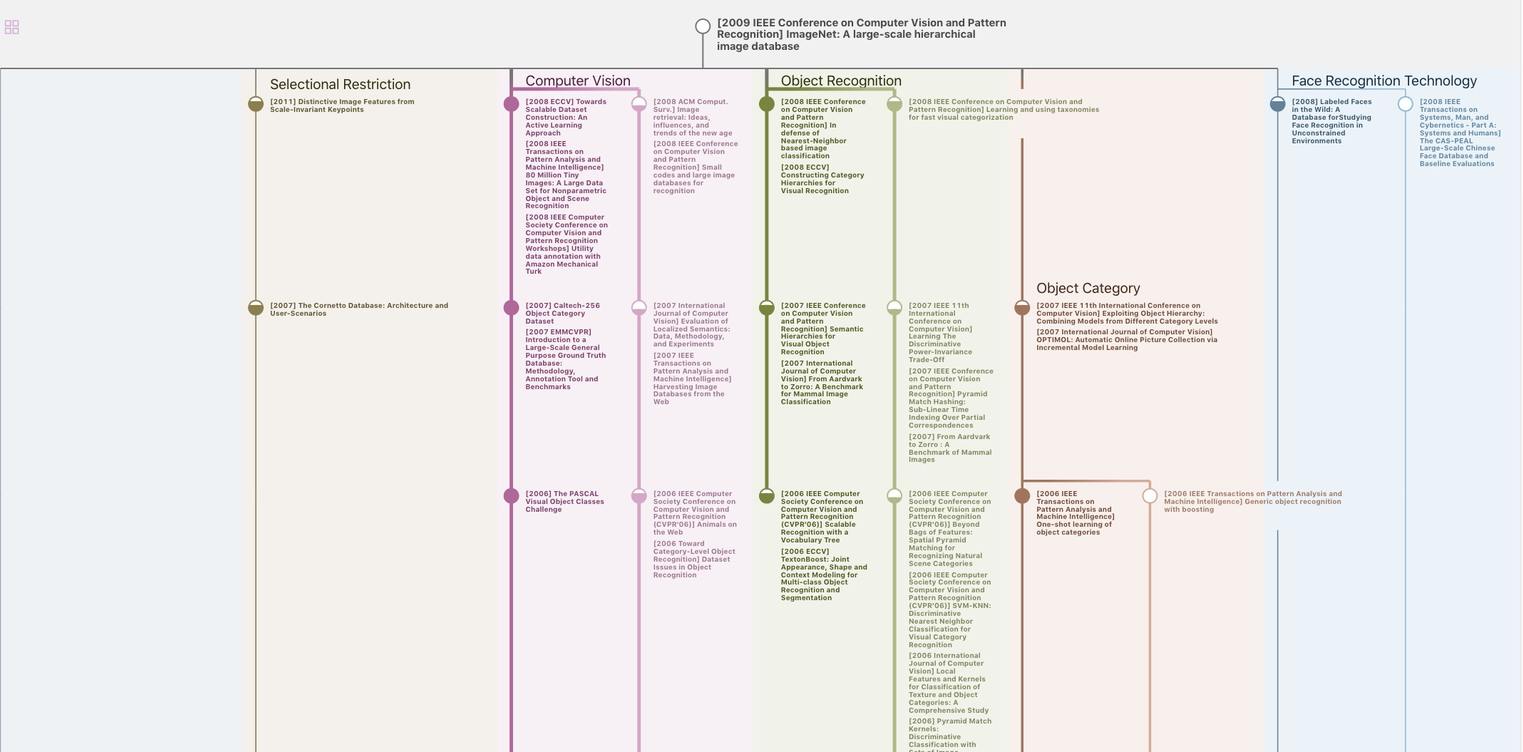
生成溯源树,研究论文发展脉络
Chat Paper
正在生成论文摘要