Jdsnmf: Joint Deep Semi-Non-Negative Matrix Factorization For Learning Integrative Representation Of Molecular Signals In Alzheimer'S Disease
JOURNAL OF PERSONALIZED MEDICINE(2021)
摘要
High dimensional multi-omics data integration can enhance our understanding of the complex biological interactions in human diseases. However, most studies involving unsupervised integration of multi-omics data focus on linear integration methods. In this study, we propose a joint deep semi-non-negative matrix factorization (JDSNMF) model, which uses a hierarchical non-linear feature extraction approach that can capture shared latent features from the complex multi-omics data. The extracted latent features obtained from JDSNMF enabled a variety of downstream tasks, including prediction of disease and module analysis. The proposed model is applicable not only to sample-matched multiple data (e.g., multi-omics data from one cohort) but also to feature-matched multiple data (e.g., omics data from multiple cohorts), and therefore it can be flexibly applied to various cases. We demonstrate the capabilities of JDSNMF using sample-matched simulated data and feature-matched multi-omics data from Alzheimer's disease cohorts, evaluating the feature extraction performance in the context of classification. In a test application, we identify AD- and age-related modules from the latent matrices using an explainable artificial intelligence and regression model. These results show that the JDSNMF model is effective in identifying latent features having a complex interplay of potential biological signatures.
更多查看译文
关键词
multi-omics, deep learning, Alzheimer's disease, matrix factorization, feature reduction, feature engineering
AI 理解论文
溯源树
样例
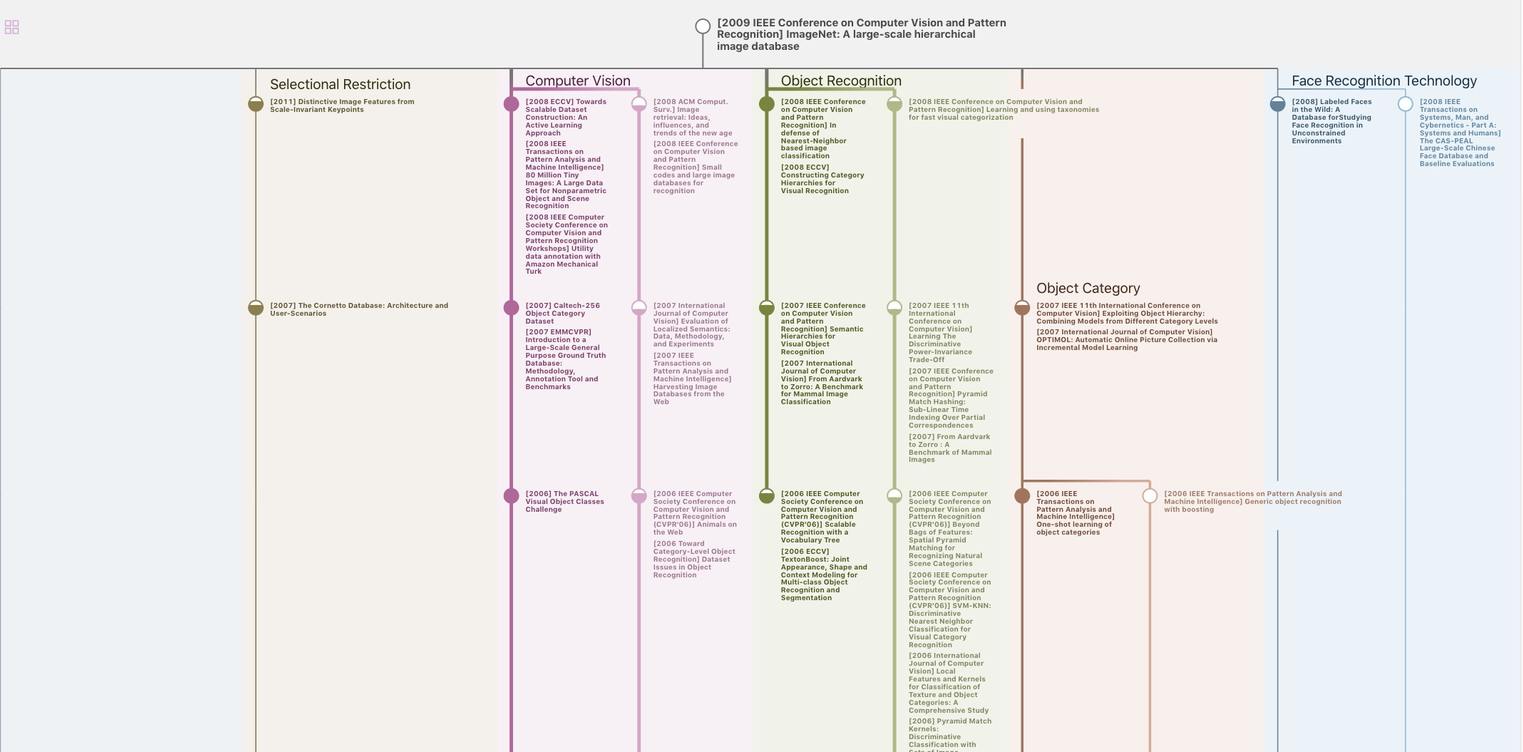
生成溯源树,研究论文发展脉络
Chat Paper
正在生成论文摘要