Deep learning based dictionary learning and tomographic image reconstruction
SIAM Journal on Imaging Sciences(2021)
摘要
This work presents an approach for image reconstruction in clinical low-dose tomography that combines principles from sparse signal processing with ideas from deep learning. First, we describe sparse signal representation in terms of dictionaries from a statistical perspective and interpret dictionary learning as a process of aligning distribution that arises from a generative model with empirical distribution of true signals. As a result we can see that sparse coding with learned dictionaries resembles a specific variational autoencoder, where the decoder is a linear function and the encoder is a sparse coding algorithm. Next, we show that dictionary learning can also benefit from computational advancements introduced in the context of deep learning, such as parallelism and as stochastic optimization. Finally, we show that regularization by dictionaries achieves competitive performance in computed tomography (CT) reconstruction comparing to state-of-the-art model based and data driven approaches.
更多查看译文
关键词
dictionary learning,generative model,deep learning,image reconstruction,computed tomography
AI 理解论文
溯源树
样例
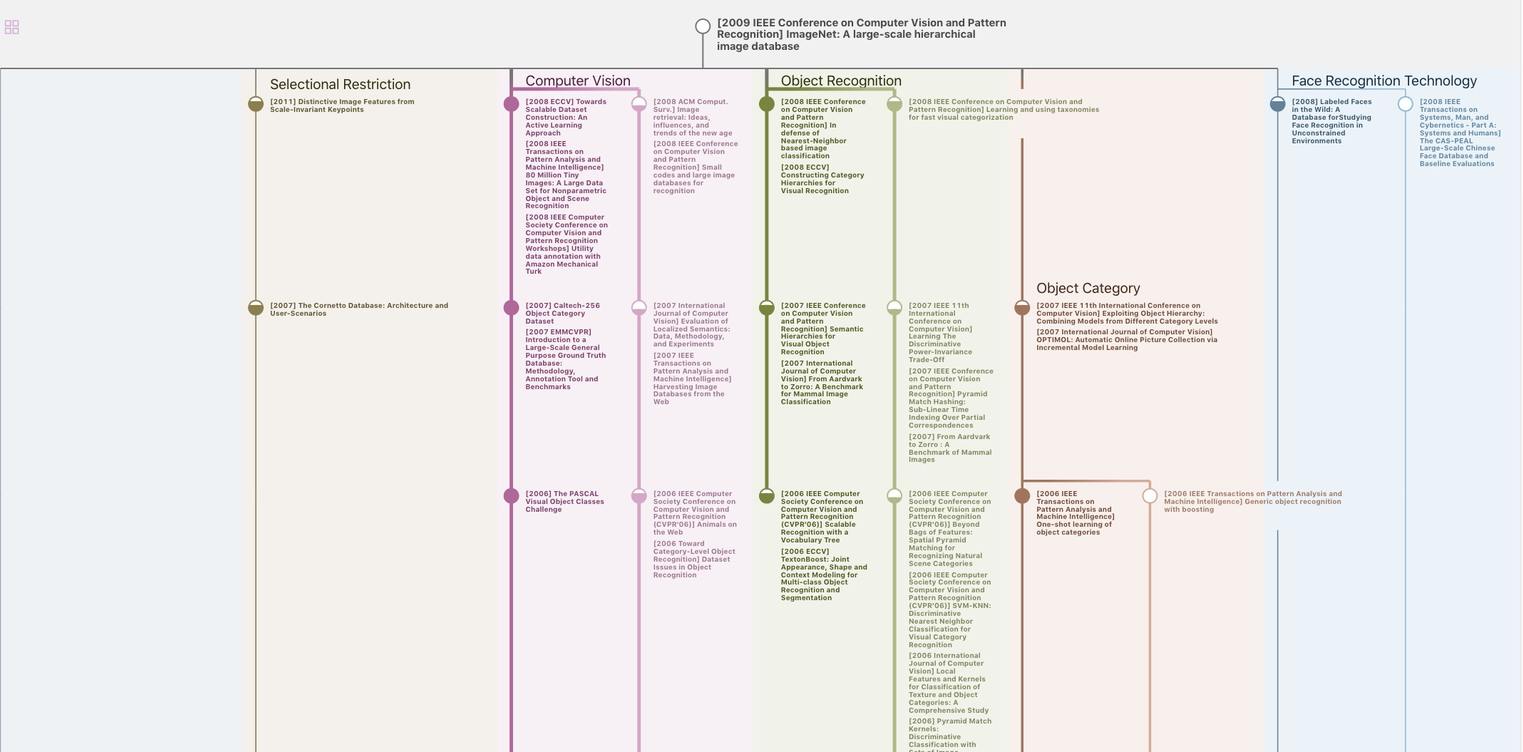
生成溯源树,研究论文发展脉络
Chat Paper
正在生成论文摘要