Disentangling ODE parameters from dynamics in VAEs
arXiv (Cornell University)(2021)
摘要
Deep networks have become increasingly of interest in dynamical system prediction, but generalization remains elusive. In this work, we consider the physical parameters of ODEs as factors of variation of the data generating process. By leveraging ideas from supervised disentanglement in VAEs, we aim to separate the ODE parameters from the dynamics in the latent space. Experiments show that supervised disentanglement allows VAEs to capture the variability in the dynamics and extrapolate better to ODE parameter spaces that were not present in the training data.
更多查看译文
关键词
ode parameters,dynamics
AI 理解论文
溯源树
样例
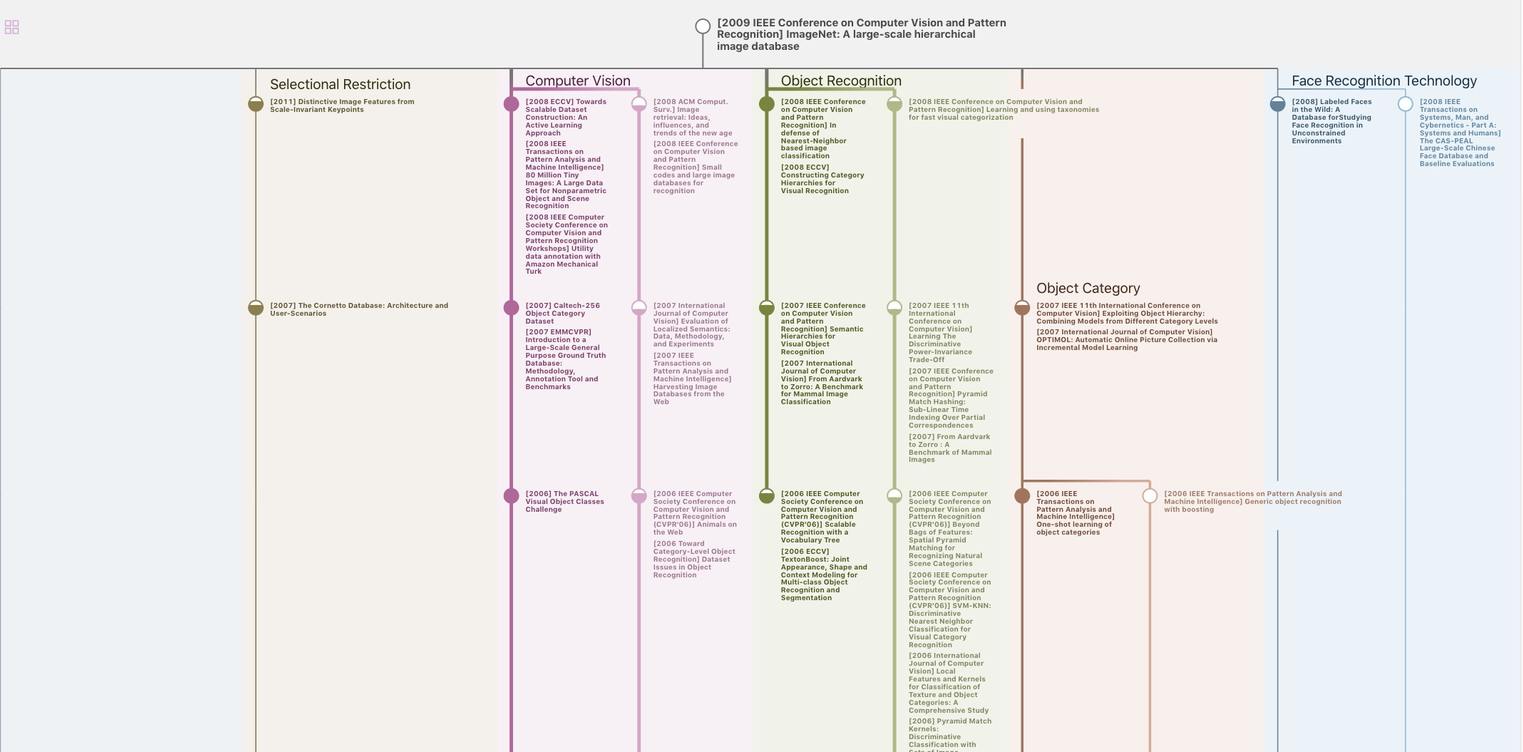
生成溯源树,研究论文发展脉络
Chat Paper
正在生成论文摘要