Applying Semi-Automated Hyperparameter Tuning for Clustering Algorithms
arXiv (Cornell University)(2021)
Abstract
When approaching a clustering problem, choosing the right clustering algorithm and parameters is essential, as each clustering algorithm is proficient at finding clusters of a particular nature. Due to the unsupervised nature of clustering algorithms, there are no ground truth values available for empirical evaluation, which makes automation of the parameter selection process through hyperparameter tuning difficult. Previous approaches to hyperparameter tuning for clustering algorithms have relied on internal metrics, which are often biased towards certain algorithms, or having some ground truth labels available, moving the problem into the semi-supervised space. This preliminary study proposes a framework for semi-automated hyperparameter tuning of clustering problems, using a grid search to develop a series of graphs and easy to interpret metrics that can then be used for more efficient domain-specific evaluation. Preliminary results show that internal metrics are unable to capture the semantic quality of the clusters developed and approaches driven by internal metrics would come to different conclusions than those driven by manual evaluation.
MoreTranslated text
Key words
clustering algorithms,semi-automated
AI Read Science
Must-Reading Tree
Example
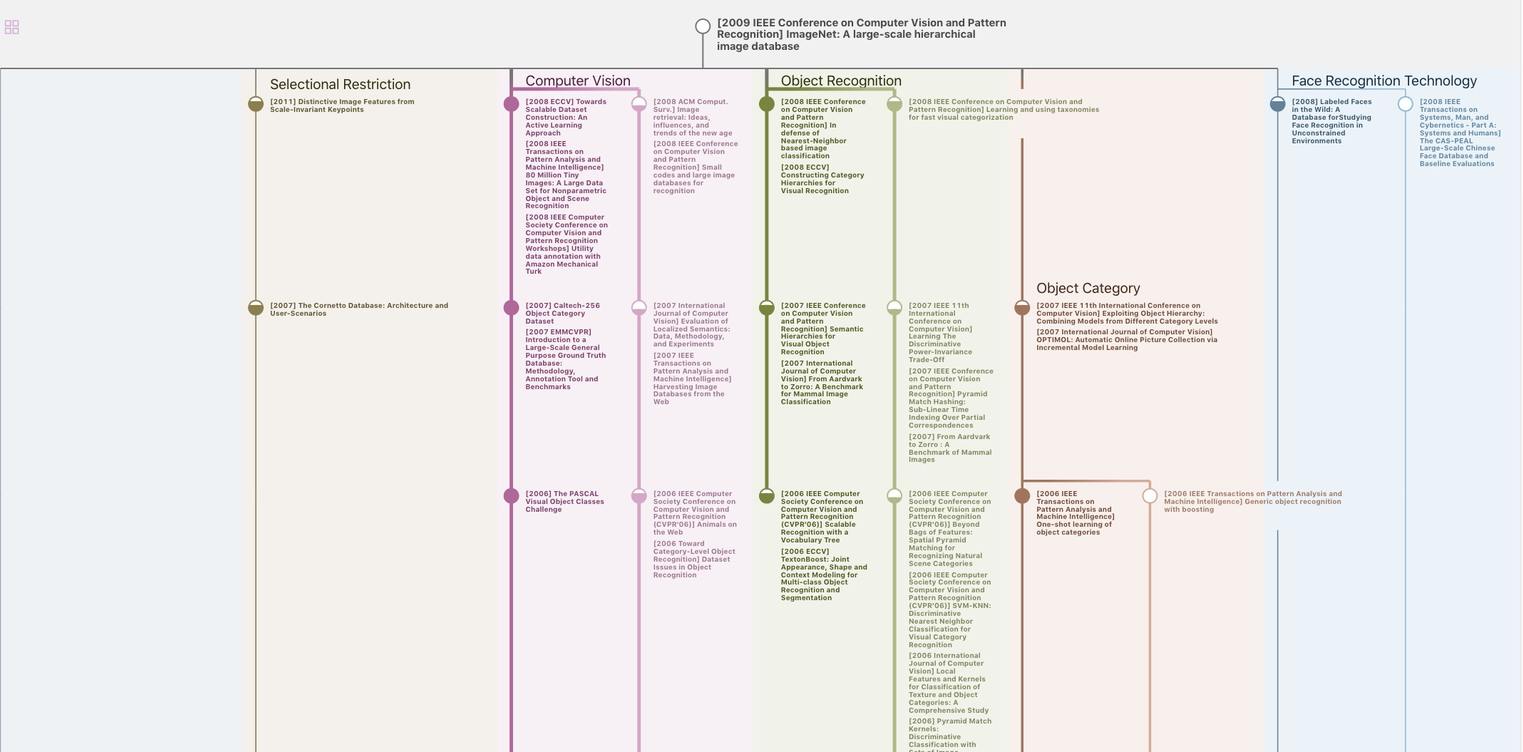
Generate MRT to find the research sequence of this paper
Chat Paper
Summary is being generated by the instructions you defined