Stochastic Scene-Aware Motion Prediction
ICCV(2021)
摘要
A long-standing goal in computer vision is to capture, model, and realistically synthesize human behavior. Specifically, by learning from data, our goal is to enable virtual humans to navigate within cluttered indoor scenes and naturally interact with objects. Such embodied behavior has applications in virtual reality, computer games, and robotics, while synthesized behavior can be used as training data. The problem is challenging because real human motion is diverse and adapts to the scene. For example, a person can sit or lie on a sofa in many places and with varying styles. We must model this diversity to synthesize virtual humans that realistically perform human-scene interactions. We present a novel data-driven, stochastic motion synthesis method that models different styles of performing a given action with a target object. Our Scene-Aware Motion Prediction method (SAMP) generalizes to target objects of various geometries while enabling the character to navigate in cluttered scenes. To train SAMP, we collected MoCap data covering various sitting, lying down, walking, and running styles. We demonstrate SAMP on complex indoor scenes and achieve superior performance than existing solutions. Code and data are available for research at https://samp.is.tue.mpg.de.
更多查看译文
关键词
motion,prediction,scene-aware
AI 理解论文
溯源树
样例
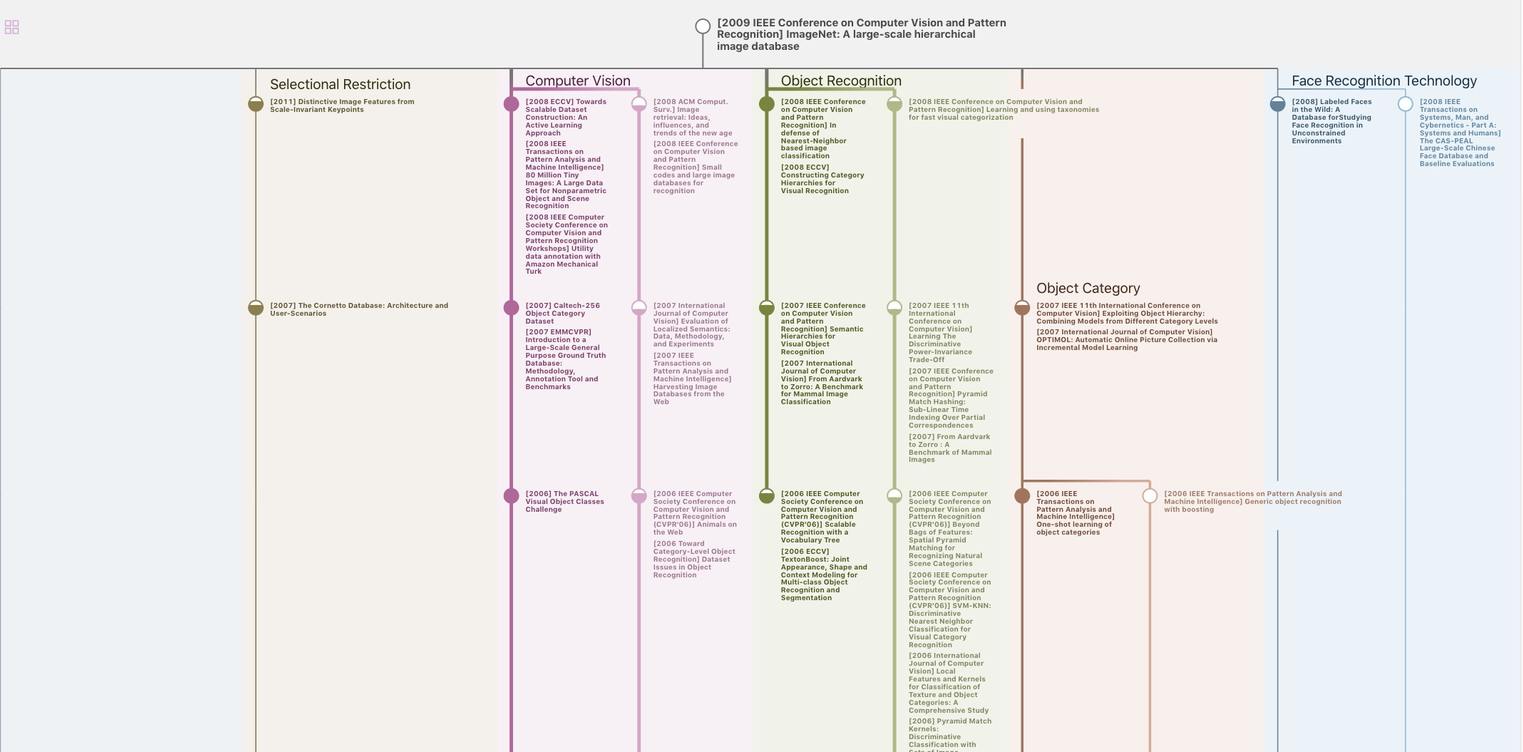
生成溯源树,研究论文发展脉络
Chat Paper
正在生成论文摘要