Implicit Regularization and Entrywise Convergence of Riemannian Optimization for Low Tucker-Rank Tensor Completion
JOURNAL OF MACHINE LEARNING RESEARCH(2023)
摘要
This paper is concerned with the low Tucker-rank tensor completion problem, which is about reconstructing a tensor T is an element of Rnxnxn of low multilinear rank from partially observed entries. Riemannian optimization algorithms are a class of efficient methods for this prob-lem, but the theoretical convergence analysis is still lacking. In this manuscript, we establish the entrywise convergence of the vanilla Riemannian gradient method for low Tucker-rank tensor completion under the nearly optimal sampling complexity O(n3/2). Meanwhile, the implicit regularization phenomenon of the algorithm has also been revealed. As far as we know, this is the first work that has shown the entrywise convergence and implicit regu-larization property of a non-convex method for low Tucker-rank tensor completion. The analysis relies on the leave-one-out technique, and some of the technical results developed in the paper might be of broader interest in investigating the properties of other non-convex methods for this problem.
更多查看译文
关键词
low rank tensor completion,Tucker decomposition,Riemannian gradient,entrywise convergence,implicit regularization,leave-one-out
AI 理解论文
溯源树
样例
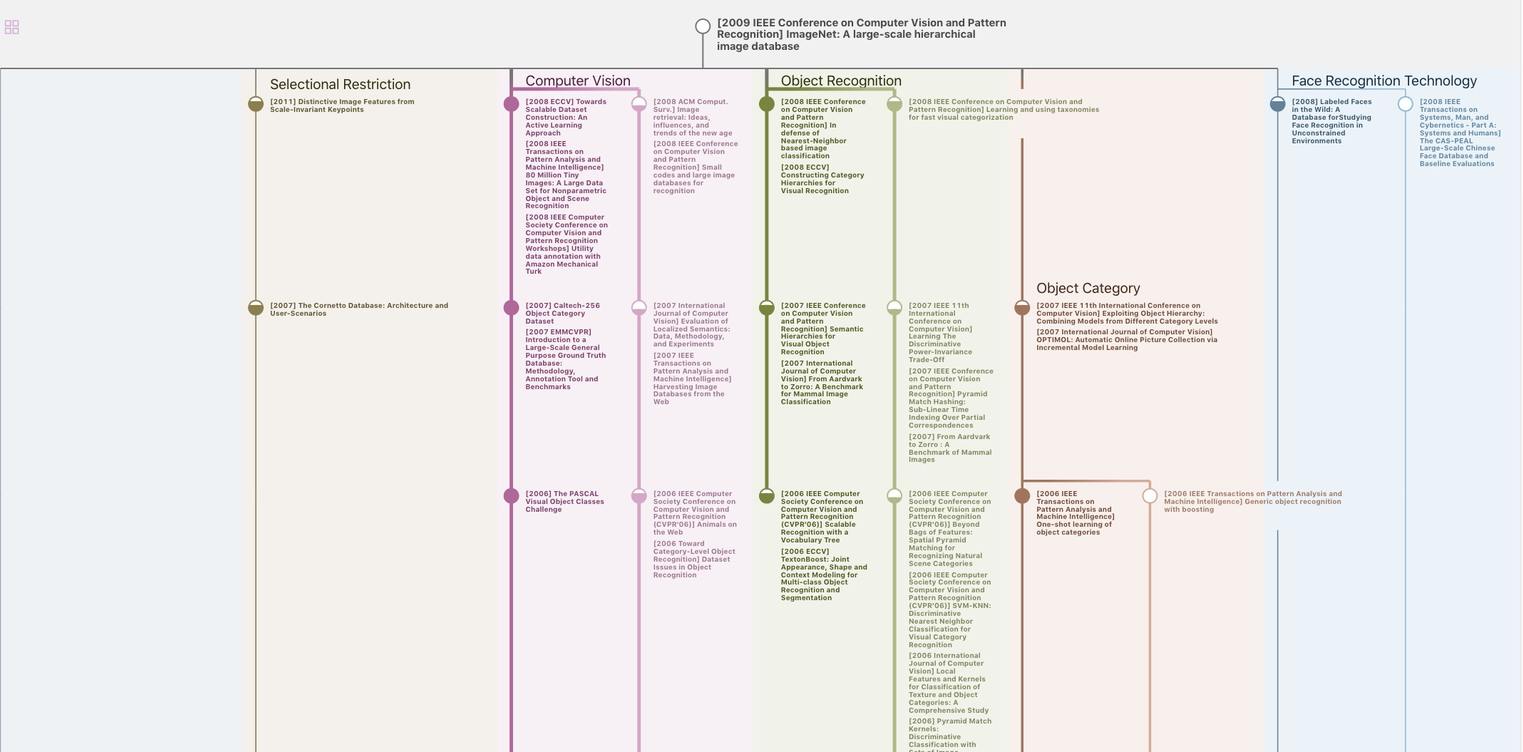
生成溯源树,研究论文发展脉络
Chat Paper
正在生成论文摘要