Cube Sampled K-Prototype Clustering for Featured Data
2021 IEEE 18th India Council International Conference (INDICON)(2021)
摘要
Clustering large amount of data is becoming increasingly important in the current times. Due to the large sizes of data, clustering algorithm often take too much time. Sampling this data before clustering is commonly used to reduce this time. In this work, we propose a probabilistic sampling technique called cube sampling along with K-Prototype clustering. Cube sampling is used because of its accurate sample selection. K-Prototype is most frequently used clustering algorithm when the data is numerical as well as categorical (very common in today’s time). The novelty of this work is in obtaining the crucial inclusion probabilities for cube sampling using Principal Component Analysis (PCA). Experiments on multiple datasets from the UCI repository demonstrate that cube sampled K-Prototype algorithm gives the best clustering accuracy among similarly sampled other popular clustering algorithms (K-Means, Hierarchical Clustering (HC), Spectral Clustering (SC)). When compared with unsampled K-Prototype, K-Means, HC and SC, it still has the best accuracy with the added advantage of reduced computational complexity (due to reduced data size).
更多查看译文
关键词
Sampling,Cube Sampling,Clustering,K-Prototype Clustering,Principal Component Analysis,Clustering Accuracy
AI 理解论文
溯源树
样例
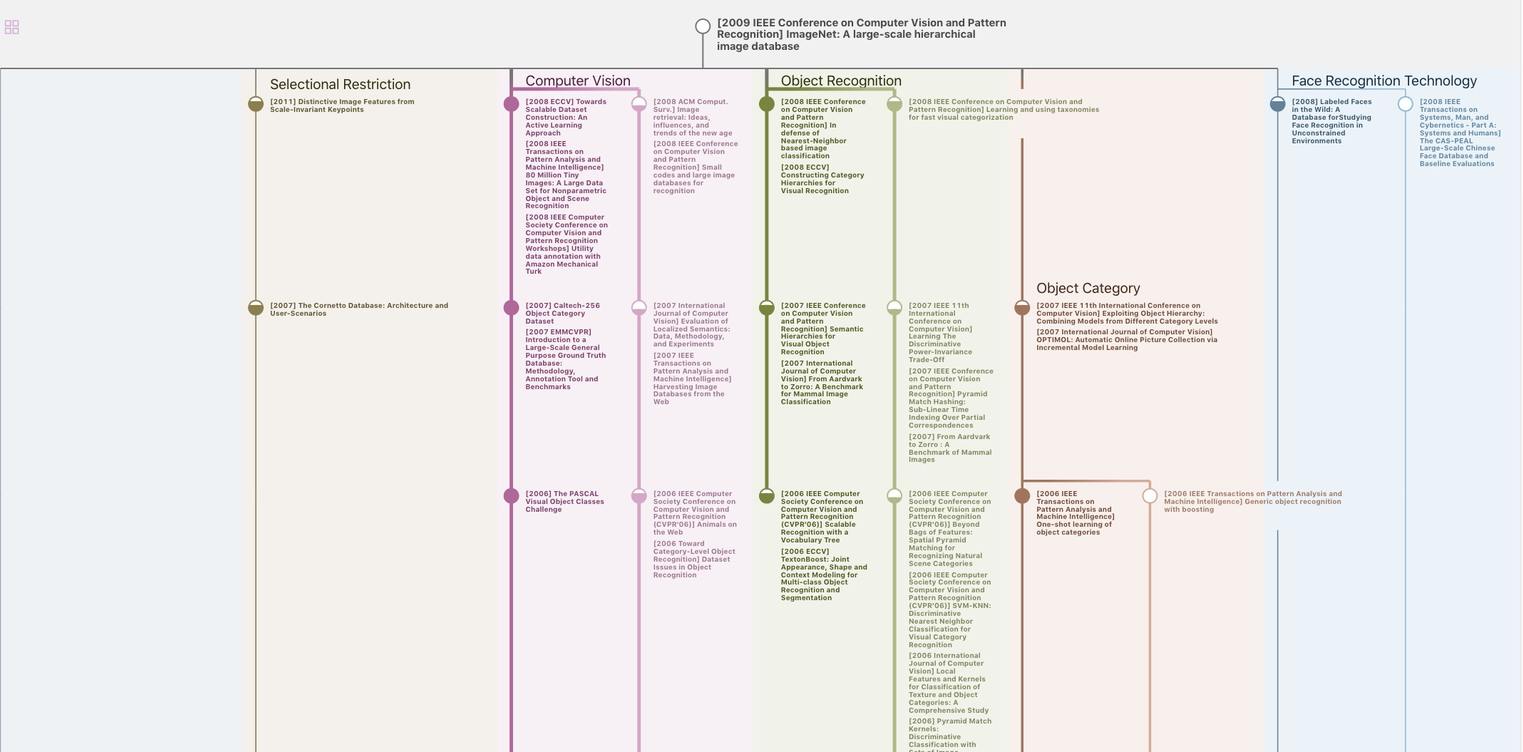
生成溯源树,研究论文发展脉络
Chat Paper
正在生成论文摘要