Sparse Regularization with a Non-Convex Penalty for SAR Imaging and Autofocusing
REMOTE SENSING(2022)
摘要
In this paper, SAR image reconstruction with joint phase error estimation (autofocusing) is formulated as an inverse problem. An optimization model utilising a sparsity-enforcing Cauchy regularizer is proposed, and an alternating minimization framework is used to solve it, in which the desired image and the phase errors are estimated alternatively. For the image reconstruction sub-problem (f-sub-problem), two methods are presented that are capable of handling the problem's complex nature. Firstly, we design a complex version of the forward-backward splitting algorithm to solve the f-sub-problem iteratively, leading to a complex forward-backward autofocusing method (CFBA). For the second variant, techniques of Wirtinger calculus are utilized to minimize the cost function involving complex variables in the f-sub-problem in a direct fashion, leading to Wirtinger alternating minimization autofocusing (WAMA) method. For both methods, the phase error estimation sub-problem is solved by simply expanding and observing its cost function. Moreover, the convergence of both algorithms is discussed in detail. Experiments are conducted on both simulated and real SAR images. In addition to the synthetic scene employed, the other SAR images focus on the sea surface, with two being real images with ship targets, and another two being simulations of the sea surface (one of them containing ship wakes). The proposed method is demonstrated to give impressive autofocusing results on these datasets compared to state-of-the-art methods.
更多查看译文
关键词
SAR autofocusing, Cauchy regularization, Wirtinger calculus, forward-backward splitting, KL property, sea surface
AI 理解论文
溯源树
样例
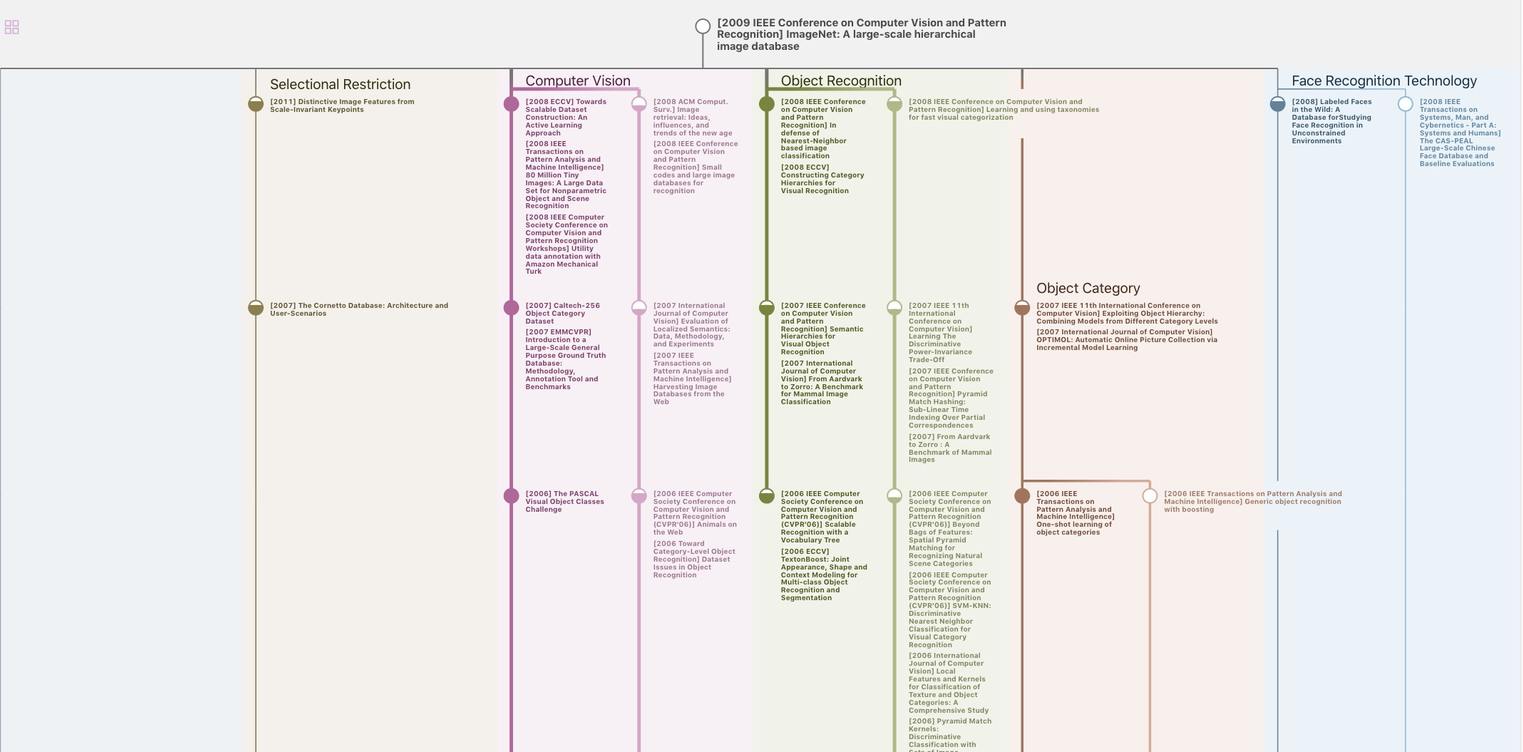
生成溯源树,研究论文发展脉络
Chat Paper
正在生成论文摘要