Towards Efficient Exploration in Unknown Spaces: A Novel Hierarchical Approach Based on Intrinsic Rewards
2021 6th International Conference on Automation, Control and Robotics Engineering (CACRE)(2021)
Abstract
Exploration in unknown environments using deep reinforcement learning (DRL) often suffers from sampling inefficiency due to notoriously sparse extrinsic rewards and complex spatial structures. To this end, we present a hierarchical and modular spatial exploration model that integrates the recently popular concept of intrinsic motivation (IM). The approach addresses the problem in two levels. On the higher level, a DRL based global module learns to determine a distant but easily reachable target that maximizes the current exploration progress, once such a target is needed by the local controller. On the lower level, a classical path planner is used to produce locally smooth movements between targets based on the known areas and free space assumption. This segmented and sequential decision-making paradigm, with an informative intrinsic reward signal, dramatically reduces training difficulty. Experimental results on diverse and challenging 2D maps show that the proposed model has consistently better exploration efficiency and generality than a state-of-the-art IM based DRL and some other heuristic methods.
MoreTranslated text
Key words
hierarchical exploration,deep reinforcement learning,intrinsic motivation,path planning
AI Read Science
Must-Reading Tree
Example
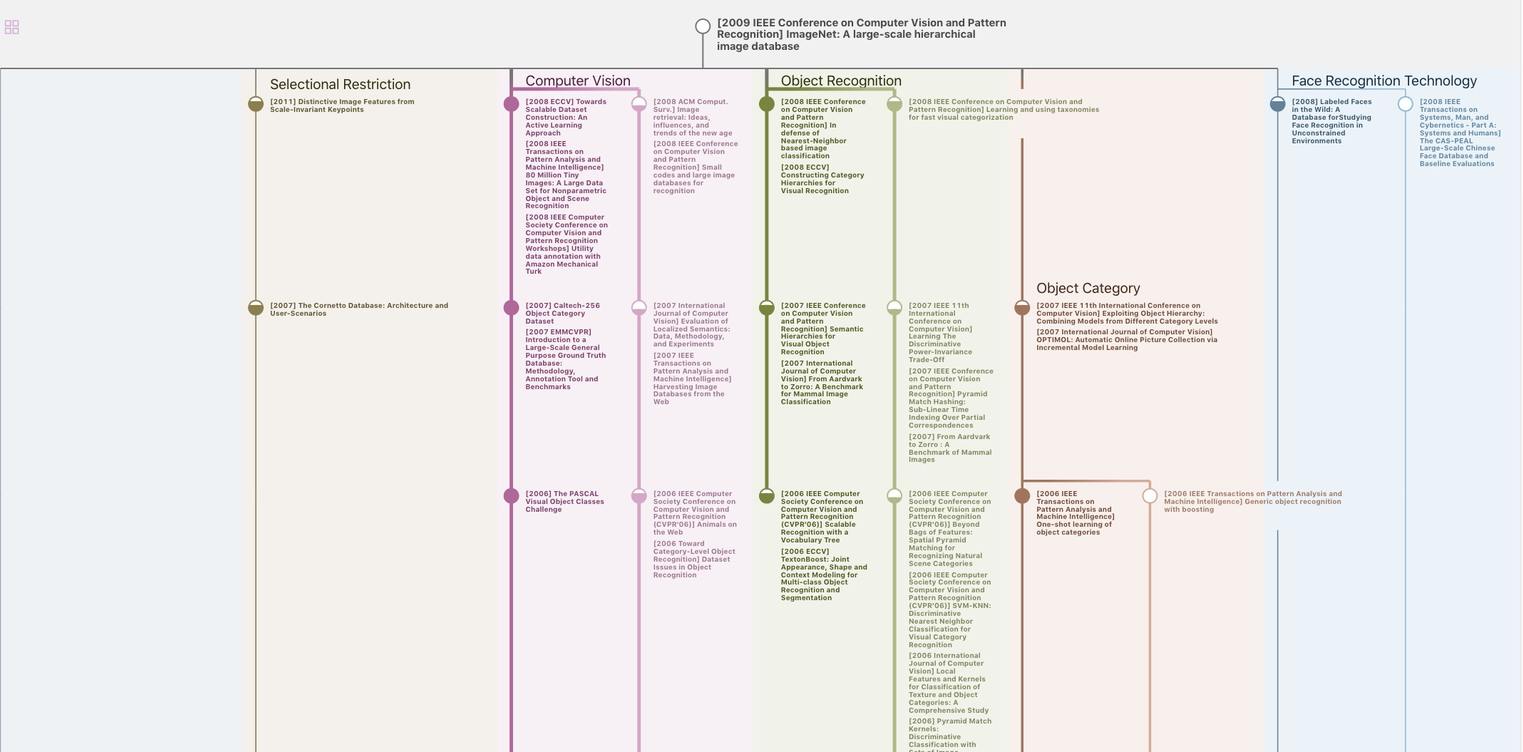
Generate MRT to find the research sequence of this paper
Chat Paper
Summary is being generated by the instructions you defined