Fairness in Networks: Social Capital, Information Access, and Interventions
Knowledge Discovery and Data Mining(2021)
摘要
ABSTRACTAs ML systems have become more broadly adopted in high-stakes settings, our scrutiny of them should reflect their greater impact on real lives. The field of fairness in data mining and machine learning has blossomed in the last decade, but most of the attention has been directed at tabular and image data. In this tutorial, we will discuss recent advances in network fairness. Specifically, we focus on problems where one's position in a network holds predictive value (e.g., in a classification or regression setting) and favorable network position can lead to a cascading loop of positive outcomes, leading to increased inequality. We start by reviewing important sociological notions such as social capital, information access, and influence, as well as the now-standard definitions of fairness in ML settings. We will discuss the formalizations of these concepts in the network fairness setting, presenting recent work in the field, and future directions.
更多查看译文
关键词
fairness, information flow, networks
AI 理解论文
溯源树
样例
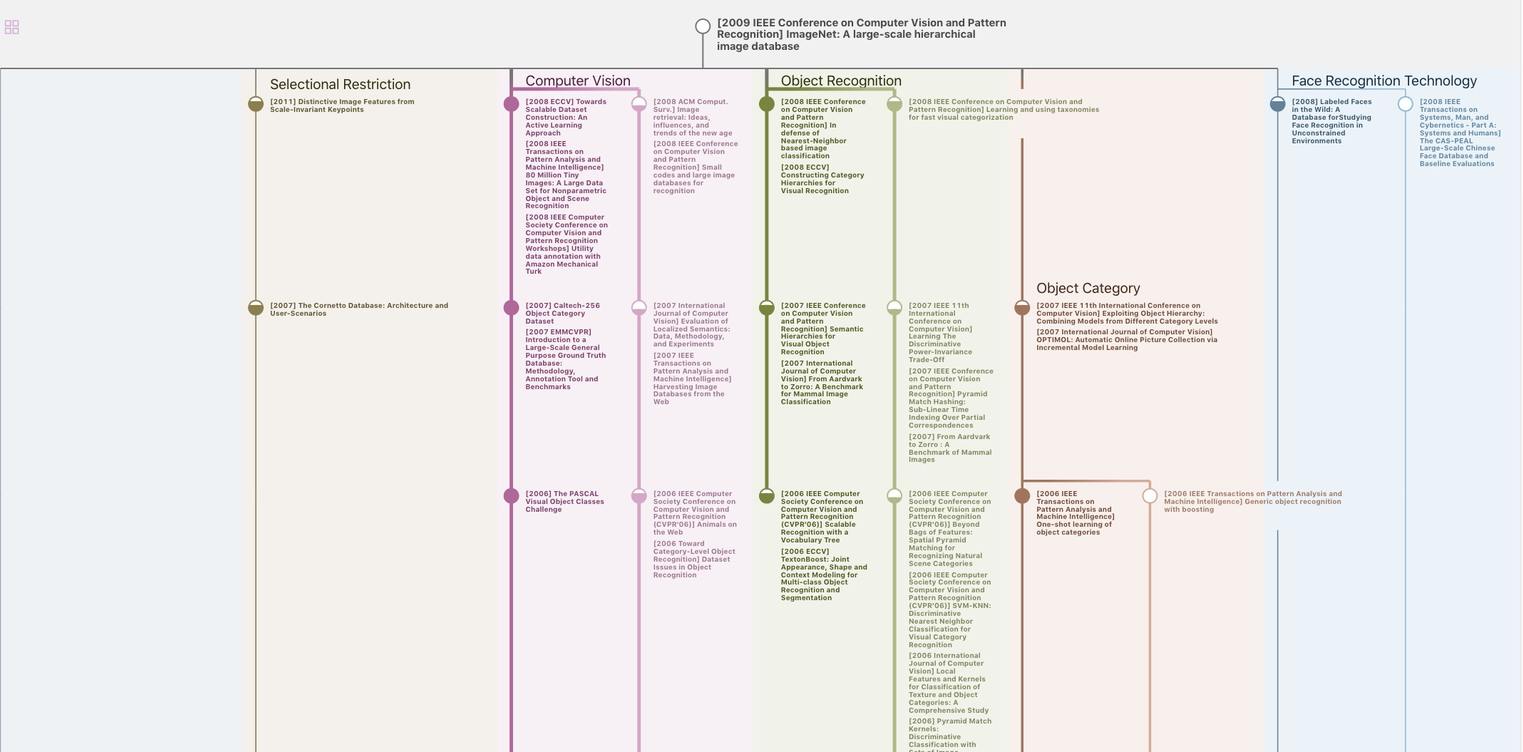
生成溯源树,研究论文发展脉络
Chat Paper
正在生成论文摘要