Linking CVE's to MITRE ATT&CK Techniques
ARES 2021: 16TH INTERNATIONAL CONFERENCE ON AVAILABILITY, RELIABILITY AND SECURITY(2021)
摘要
The MITRE Corporation is a non-profit organization that has made substantial efforts into creating and maintaining knowledge bases relevant to cybersecurity and has been widely adopted by the community. ATT&CK "Adversarial Tactics, Techniques, and Common Knowledge" is a popular taxonomy by MITRE, which describes threat actor behaviors. Techniques are the foundation of the ATT&CK model, they are the actions that adversaries perform to accomplish goals, which translate into the model's tactics. The aim of ATT&CK is to categorize adversary behavior to help improve the post-compromise detection of advanced intrusions. Software vulnerabilities (CVE) play an important role in cyber-intrusions, mostly classified into 4 ATT&CK techniques, which cover the exploitation phase of the attack chain. Identifying vulnerabilities that are actively exploited by the attackers, and understanding how a vulnerability can enable the attacker at each stage of the attack life cycle is absolutely critical for vulnerability assessments. Given the sparse classification of a CVE into ATT&CK taxonomy, lack of methods to extract labels from threat reports and, the volume of vulnerabilities disclosed defenders lack a concrete approach to prioritize CVE's based on their role in the attack chain and in the context of controls in place. In this work, we propose a Multi-Head Joint Embedding Neural Network model to automatically map CVE's to ATT&CK techniques. We address the problem of lack of labels for this task, by a novel unsupervised labeling technique. We enrich CVE's with a curated knowledgebase 50 mitigation strategies, which help the model to learn both attacker and defender view of a given CVE. We evaluate our approach with the dataset containing CVE's disclosed from the past 10 years and compare it with standard baseline models and ablation analysis. Using the proposed model, we mapped 62, 000 CVE records to 37 different ATT&CK techniques and show that the proposed multi head design performs well in the absence of labels in the training dataset.
更多查看译文
关键词
CVE, Attack Models, Deep Learning, ATT&CK, unsupervised labeling
AI 理解论文
溯源树
样例
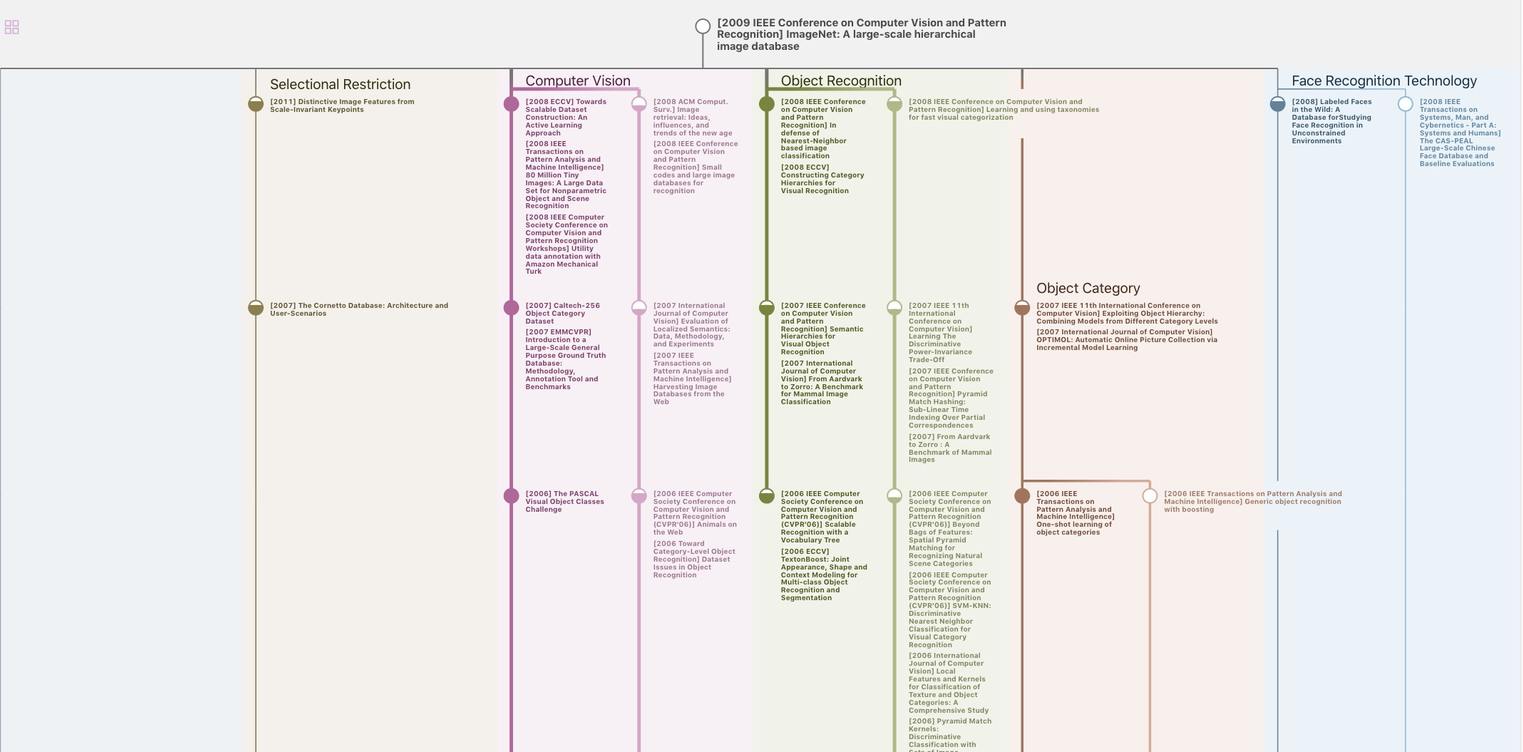
生成溯源树,研究论文发展脉络
Chat Paper
正在生成论文摘要