An empirical investigation of practical log anomaly detection for online service systems
Foundations of Software Engineering(2021)
摘要
ABSTRACTLog data is an essential and valuable resource of online service systems, which records detailed information of system running status and user behavior. Log anomaly detection is vital for service reliability engineering, which has been extensively studied. However, we find that existing approaches suffer from several limitations when deploying them into practice, including 1) inability to deal with various logs and complex log abnormal patterns; 2) poor interpretability; 3) lack of domain knowledge. To help understand these practical challenges and investigate the practical performance of existing work quantitatively, we conduct the first empirical study and an experimental study based on large-scale real-world data. We find that logs with rich information indeed exhibit diverse abnormal patterns (e.g., keywords, template count, template sequence, variable value, and variable distribution). However, existing approaches fail to tackle such complex abnormal patterns, producing unsatisfactory performance. Motivated by obtained findings, we propose a generic log anomaly detection system named LogAD based on ensemble learning, which integrates multiple anomaly detection approaches and domain knowledge, so as to handle complex situations in practice. About the effectiveness of LogAD, the average F1-score achieves 0.83, outperforming all baselines. Besides, we also share some success cases and lessons learned during our study. To our best knowledge, we are the first to investigate practical log anomaly detection in the real world deeply. Our work is helpful for practitioners and researchers to apply log anomaly detection to practice to enhance service reliability.
更多查看译文
关键词
Log Anomaly Detection, Online Service Systems, Practical Challenges
AI 理解论文
溯源树
样例
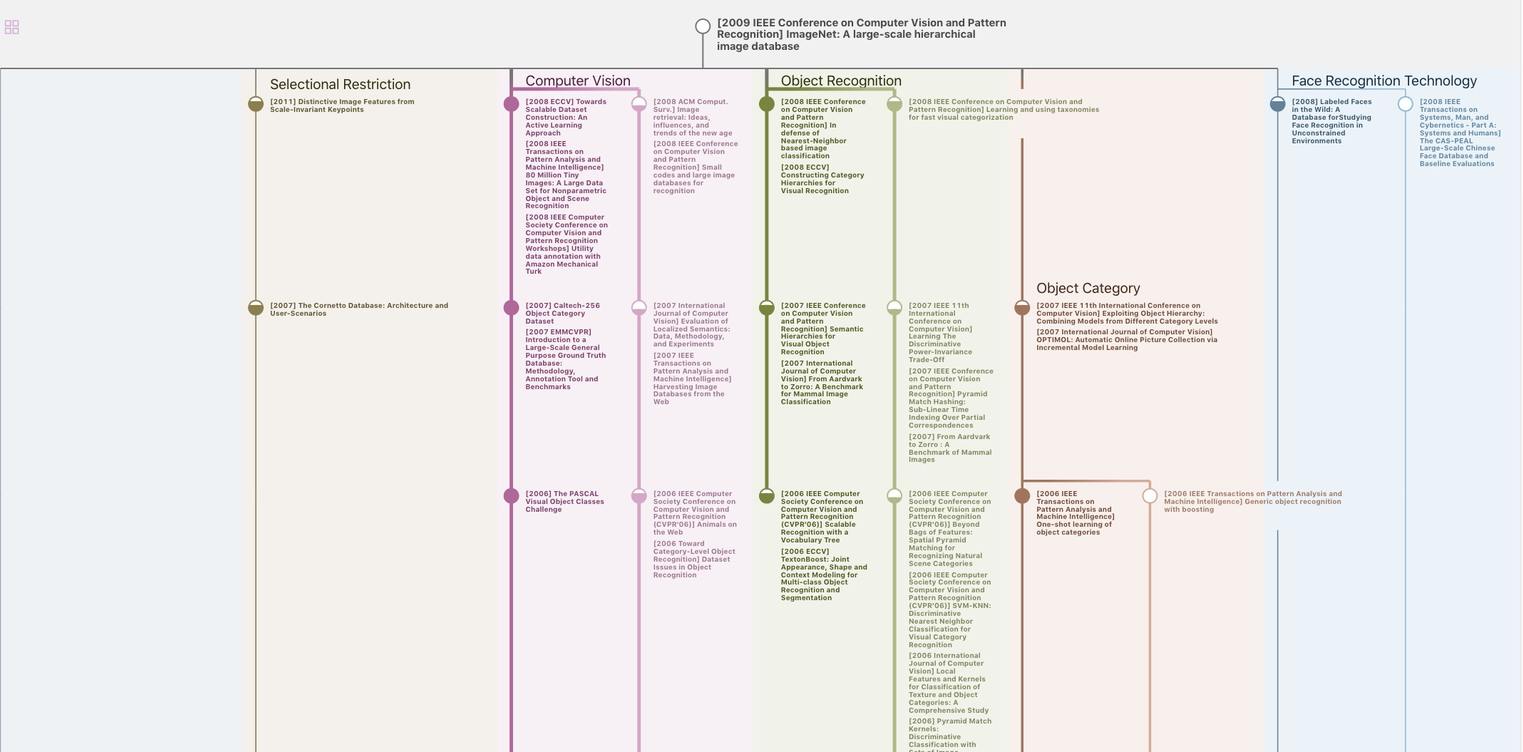
生成溯源树,研究论文发展脉络
Chat Paper
正在生成论文摘要