A residual-driven adaptive Gaussian mixture approximation for Bayesian inverse problems
Journal of Computational and Applied Mathematics(2022)
摘要
In this article, we develop a residual-driven adaptive Gaussian mixture approximation (RD-AGMA) for Bayesian inverse problems. The posterior distribution is often non-Gaussian in practical Bayesian inference. To obtain a good approximation of the posterior, we provide the adaptive Gaussian mixture approximation (GMA) based on a residual. For GMA, the clustering of ensemble samples provides the predictor of means, covariances and weights by smoothed expectation–maximization (SmEM). SmEM can overcome the singularity of covariance matrix for small ensemble size. Then the parameters of GMA are updated by an iterative ensemble smoother (IES). To enhance clustering efficiency, the ensemble samples for the clustering are updated by IES as well. Since the goal of inverse problems is to minimize the residual between the observation data and the model response, the adaptive GMA of the posterior is constructed through a residual threshold. The mixture components with large residuals will be discarded in the adaptive procedure. When the prior is incorporated into the likelihood model, small residuals can drive the AGMA close to the true posterior. In the proposed method, a large number of samples can be efficiently drawn from the posterior distribution of GMA. A few numerical examples are presented to demonstrate the efficacy of RD-AGMA with applications in multimodal inversion and channel identification for subsurface flow problems in porous media.
更多查看译文
关键词
Residual-driven,Gaussian mixture approximation,Smoothed expectation–maximization,Multimodal identification
AI 理解论文
溯源树
样例
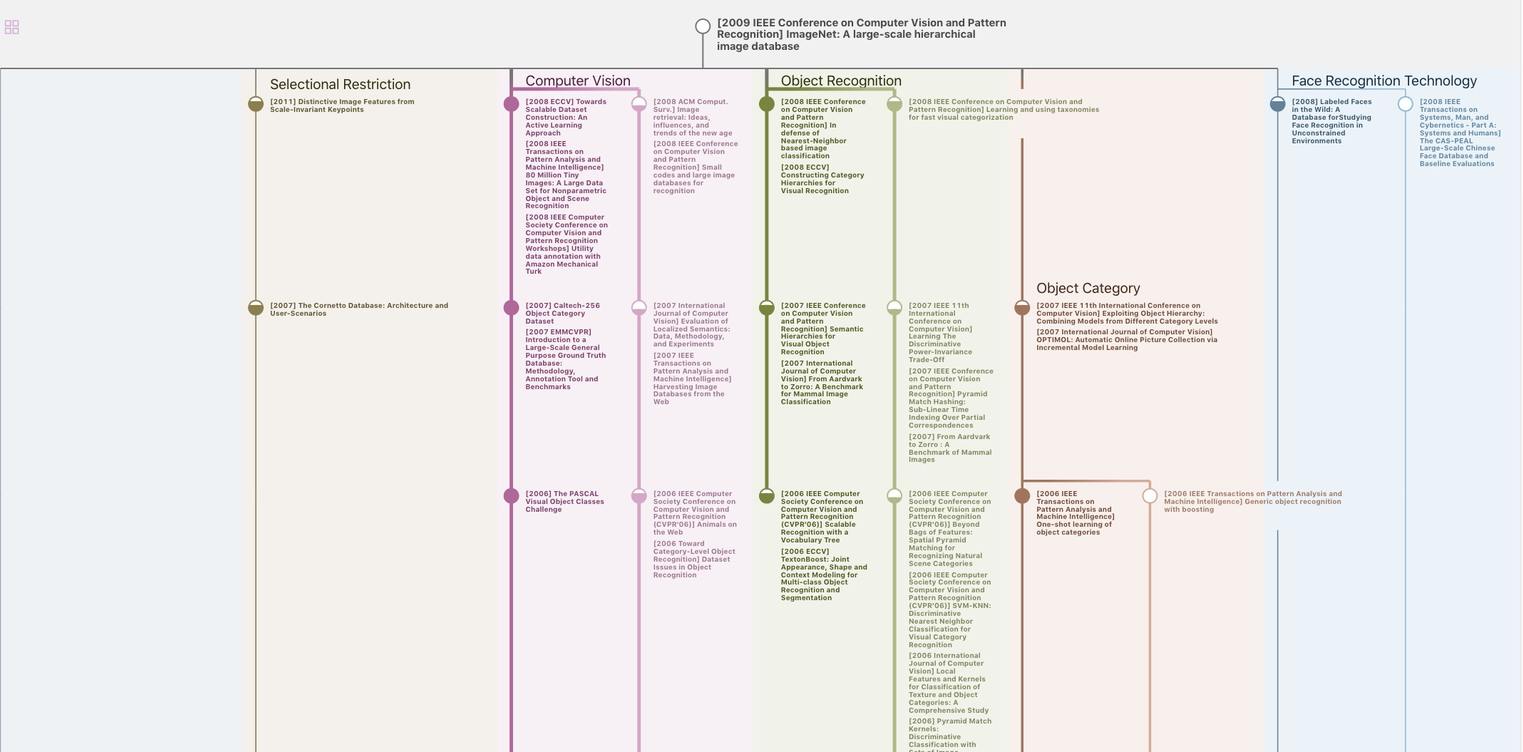
生成溯源树,研究论文发展脉络
Chat Paper
正在生成论文摘要