Deep Learning Approach for Quantification of Fluorescently Labeled Blood Cells in Danio rerio (Zebrafish)
BIOINFORMATICS AND BIOLOGY INSIGHTS(2021)
摘要
Neutrophils are a type of white blood cell essential for the function of the innate immune system. To elucidate mechanisms of neutrophil biology, many studies are performed in vertebrate animal model systems. In Danio rerio (zebrafish), in vivo imaging of neutrophils is possible due to transgenic strains that possess fluorescently labeled leukocytes. However, due to the relative abundance of neutrophils, the counting process is laborious and subjective. In this article, we propose the use of a custom trained "you only look once" (YOLO) machine learning algorithm to automate the identification and counting of fluorescently labeled neutrophils in zebrafish. Using this model, we found the correlation coefficient between human counting and the model equals r = 0.8207 with an 8.65% percent error, while variation among human counters was 5% to 12%. Importantly, the model was able to correctly validate results of a previously published article that quantitated neutrophils manually. While the accuracy can be further improved, this model notably achieves these results in mere minutes compared with hours via standard manual counting protocols and can be performed by anyone with basic programming knowledge. It further supports the use of deep learning models for high throughput analysis of fluorescently labeled blood cells in the zebrafish model system.
更多查看译文
关键词
Zebrafish, myeloid cells, quantitation of blood cells, deep learning, YOLO
AI 理解论文
溯源树
样例
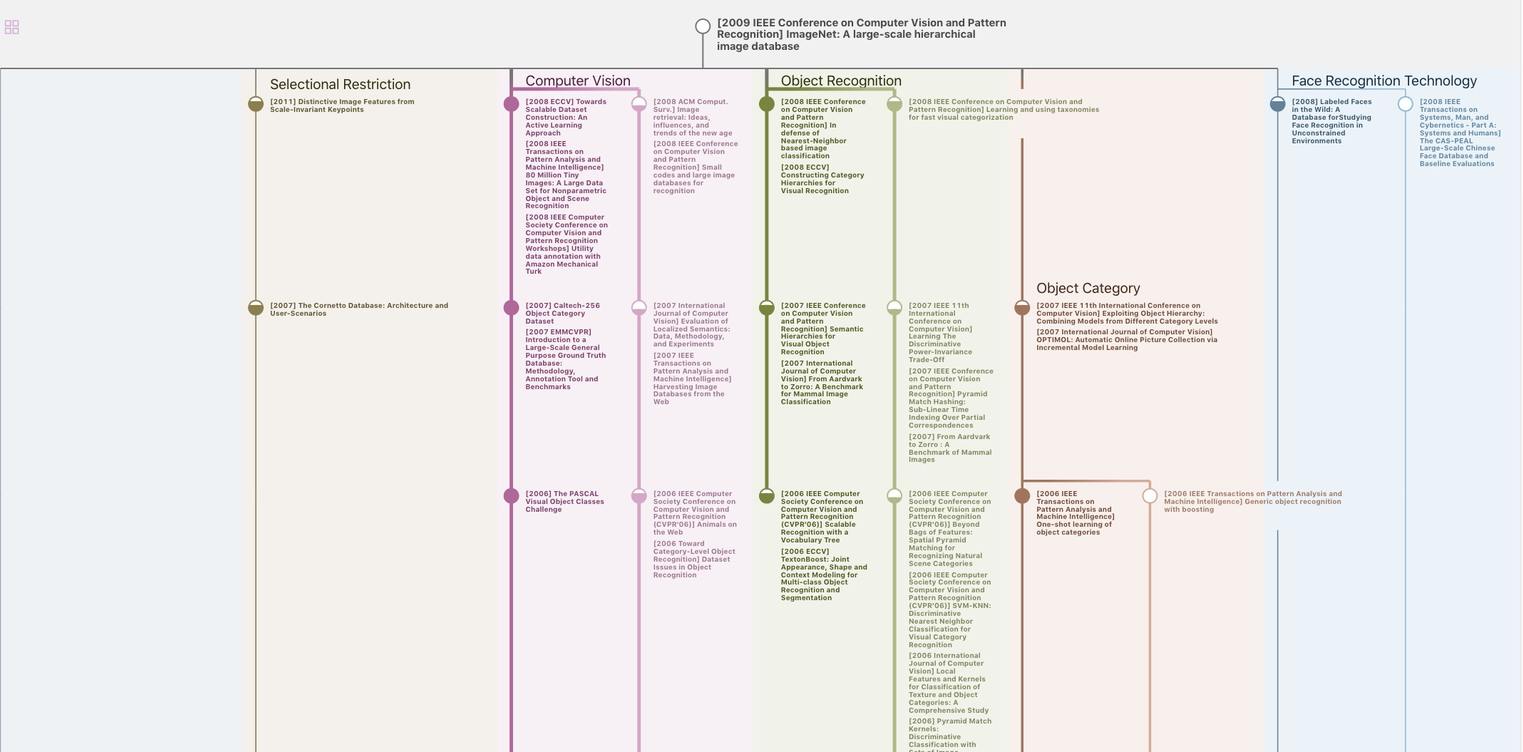
生成溯源树,研究论文发展脉络
Chat Paper
正在生成论文摘要