Deep learning-based model for diagnosing Alzheimer's disease and tauopathies
NEUROPATHOLOGY AND APPLIED NEUROBIOLOGY(2022)
摘要
Aims This study aimed to develop a deep learning-based model for differentiating tauopathies, including Alzheimer's disease (AD), progressive supranuclear palsy (PSP), corticobasal degeneration (CBD) and Pick's disease (PiD), based on tau-immunostained digital slide images. Methods We trained the YOLOv3 object detection algorithm to detect five tau lesion types: neuronal inclusions, neuritic plaques, tufted astrocytes, astrocytic plaques and coiled bodies. We used 2522 digital slide images of CP13-immunostained slides of the motor cortex from 10 cases each of AD, PSP and CBD for training. Data augmentation was performed to increase the size of the training dataset. We next constructed random forest classifiers using the quantitative burdens of each tau lesion from motor cortex, caudate nucleus and superior frontal gyrus, ascertained from the object detection model. We split 120 cases (32 AD, 36 PSP, 31 CBD and 21 PiD) into training (90 cases) and test (30 cases) sets to train random forest classifiers. Results The resultant random forest classifier achieved an average test score of 0.97, indicating that 29 out of 30 cases were correctly diagnosed. A validation study using hold-out datasets of CP13- and AT8-stained slides from 50 cases (10 AD, 17 PSP, 13 CBD and 10 PiD) showed >92% (without data augmentation) and >95% (with data augmentation) diagnostic accuracy in both CP13- and AT8-stained slides. Conclusion Our diagnostic model trained with CP13 also works for AT8; therefore, our diagnostic tool can be potentially used by other investigators and may assist medical decision-making in neuropathological diagnoses of tauopathies.
更多查看译文
关键词
Alzheimer's disease, corticobasal degeneration, machine learning, object detection, Pick's disease, progressive supranuclear palsy, random forest classifier
AI 理解论文
溯源树
样例
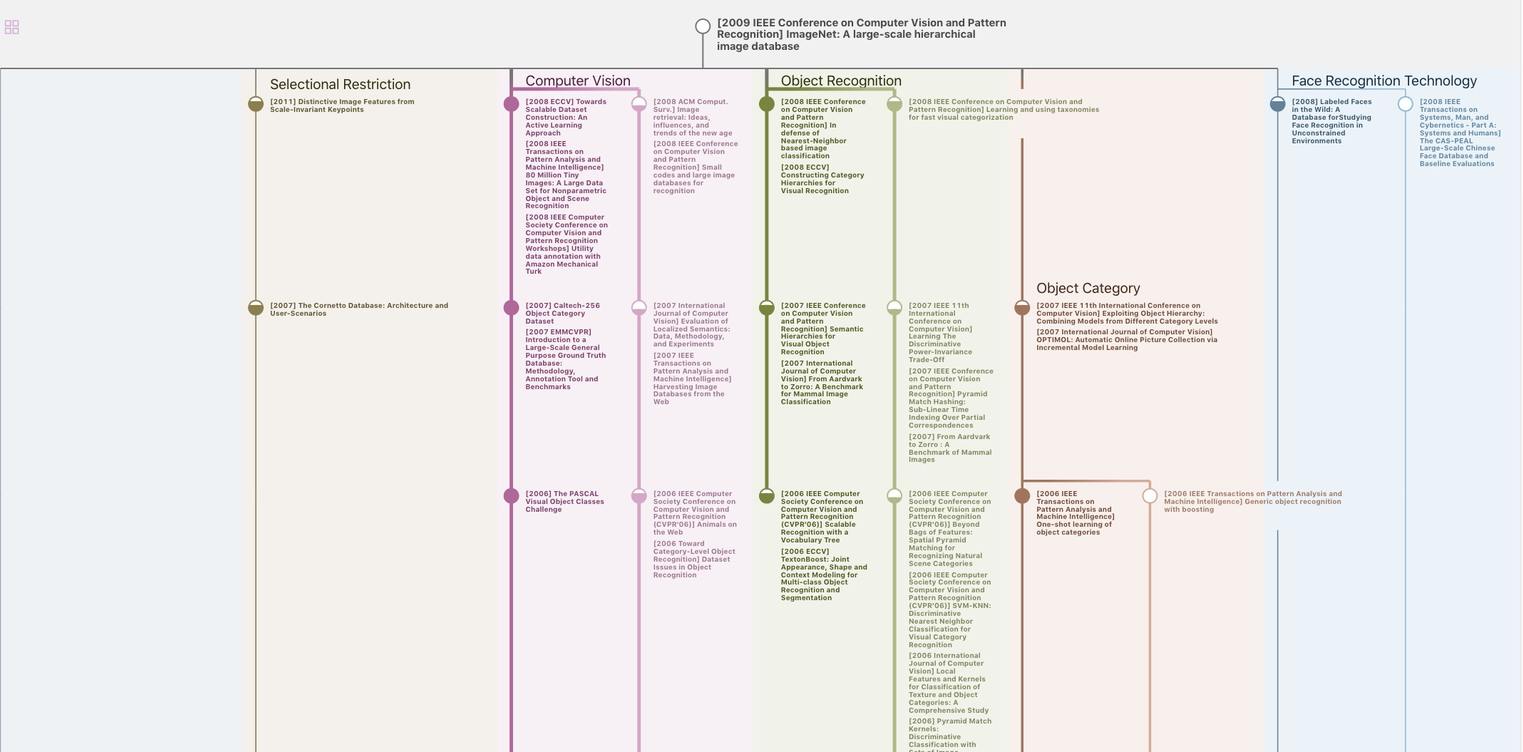
生成溯源树,研究论文发展脉络
Chat Paper
正在生成论文摘要