Advancing Imbalanced Domain Adaptation: Cluster-Level Discrepancy Minimization With a Comprehensive Benchmark
IEEE Transactions on Cybernetics(2023)
摘要
Unsupervised domain adaptation methods have been proposed to tackle the problem of covariate shift by minimizing the distribution discrepancy between the feature embeddings of source domain and target domain. However, the standard evaluation protocols assume that the conditional label distributions of the two domains are invariant, which is usually not consistent with the real-world scenarios such as long-tailed distribution of visual categories. In this article, the imbalanced domain adaptation (IDA) is formulated for a more realistic scenario where both label shift and covariate shift occur between the two domains. Theoretically, when label shift exists, aligning the marginal distributions may result in negative transfer. Therefore, a novel cluster-level discrepancy minimization (CDM) is developed. CDM proposes cross-domain similarity learning to learn tight and discriminative clusters, which are utilized for both feature-level and distribution-level discrepancy minimization, palliating the negative effect of label shift during domain transfer. Theoretical justifications further demonstrate that CDM minimizes the target risk in a progressive manner. To corroborate the effectiveness of CDM, we propose two evaluation protocols according to the real-world situation and benchmark existing domain adaptation approaches. Extensive experiments demonstrate that negative transfer does occur due to label shift, while our approach achieves significant improvement on imbalanced datasets, including Office-31, Image-CLEF, and Office-Home.
更多查看译文
关键词
Cross-domain classification,deep clustering,domain adaptation,label shift,long-tailed distribution
AI 理解论文
溯源树
样例
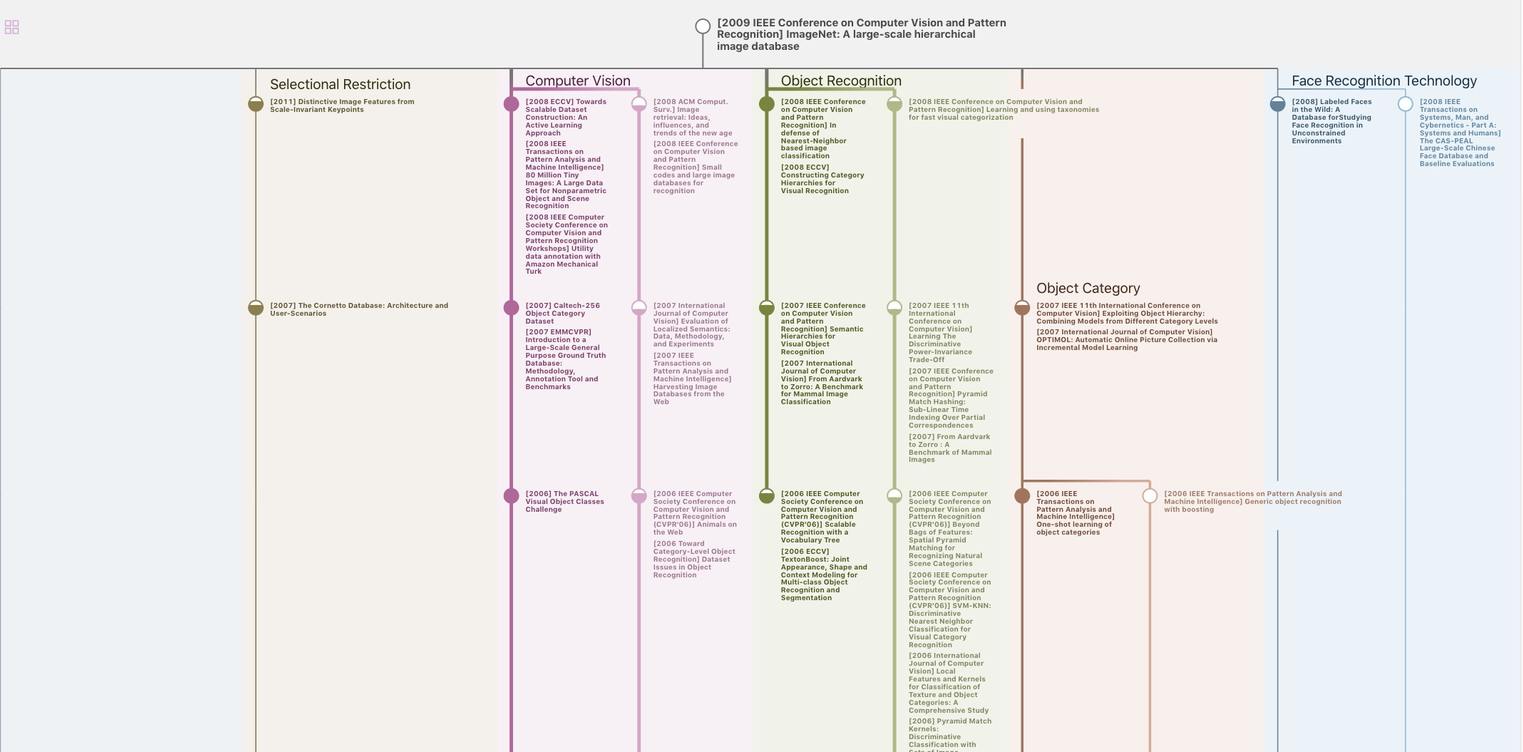
生成溯源树,研究论文发展脉络
Chat Paper
正在生成论文摘要