A semi-supervised autoencoder framework for joint generation and classification of breathing.
COMPUTER METHODS AND PROGRAMS IN BIOMEDICINE(2021)
摘要
BACKGROUND AND OBJECTIVE:One of the main problems with biomedical signals is the limited amount of patient-specific data and the significant amount of time needed to record the sufficient number of samples needed for diagnostic and treatment purposes. In this study, we present a framework to simultaneously generate and classify biomedical time series based on a modified Adversarial Autoencoder (AAE) algorithm and one-dimensional convolutions. Our work is based on breathing time series, with specific motivation to capture breathing motion during radiotherapy lung cancer treatments.
METHODS:First, we explore the potential in using the Variational Autoencoder (VAE) and AAE algorithms to model breathing signals from individual patients. We then extend the AAE algorithm to allow joint semi-supervised classification and generation of different types of signals within a single framework. To simplify the modeling task, we introduce a pre-processing and post-processing compressing algorithm that transforms the multi-dimensional time series into vectors containing time and position values, which are transformed back into time series through an additional neural network.
RESULTS:The resulting models are able to generate realistic and varied samples of breathing. By incorporating 4% and 12% of the labeled samples during training, our model outperforms other purely discriminative networks in classifying breathing baseline shift irregularities from a dataset completely different from the training set, achieving an average macro F1-score of 94.91% and 96.54%, respectively.
CONCLUSION:To our knowledge, the presented framework is the first approach that unifies generation and classification within a single model for this type of biomedical data, enabling both computer aided diagnosis and augmentation of labeled samples within a single framework.
更多查看译文
关键词
Deep learning, Respiratory motion, Breathing signals, Convolutional neural network, Probabilistic autoencoder, Semi-supervised learning
AI 理解论文
溯源树
样例
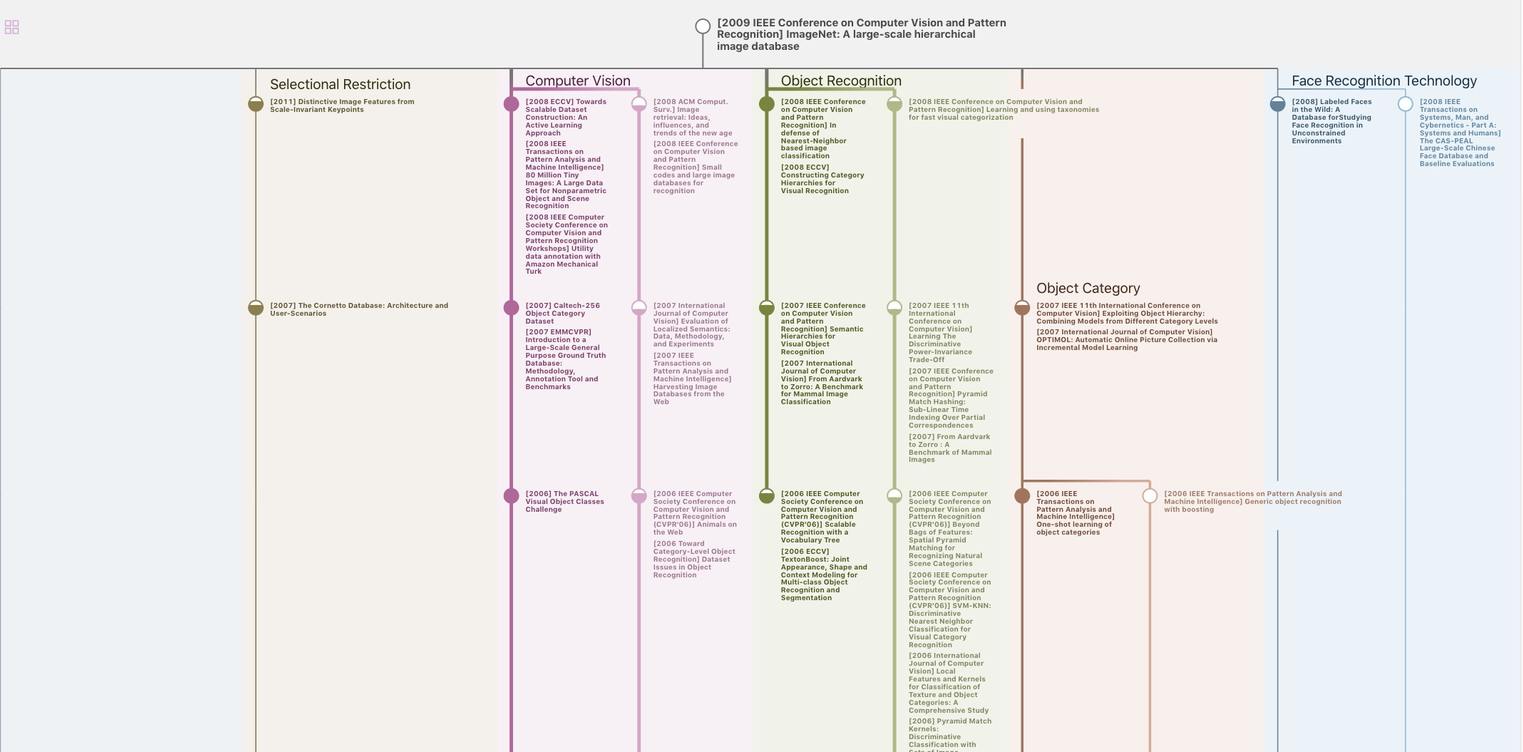
生成溯源树,研究论文发展脉络
Chat Paper
正在生成论文摘要