Parameters not empirically identifiable or distinguishable, including correlation between Gaussian observations
STATISTICAL PAPERS(2023)
摘要
It is shown that some theoretically identifiable parameters cannot be empirically identified, meaning that no consistent estimator of them can exist. An important example is a constant correlation between Gaussian observations (in presence of such correlation not even the mean can be empirically identified). Empirical identifiability and three versions of empirical distinguishability are defined. Two different constant correlations between Gaussian observations cannot even be empirically distinguished. A further example are cluster membership parameters in k-means clustering. Several existing results in the literature are connected to the new framework. General conditions are discussed under which independence can be distinguished from dependence.
更多查看译文
关键词
Independence testing,Random effect,Estimability,K-means clustering,Model assumptions
AI 理解论文
溯源树
样例
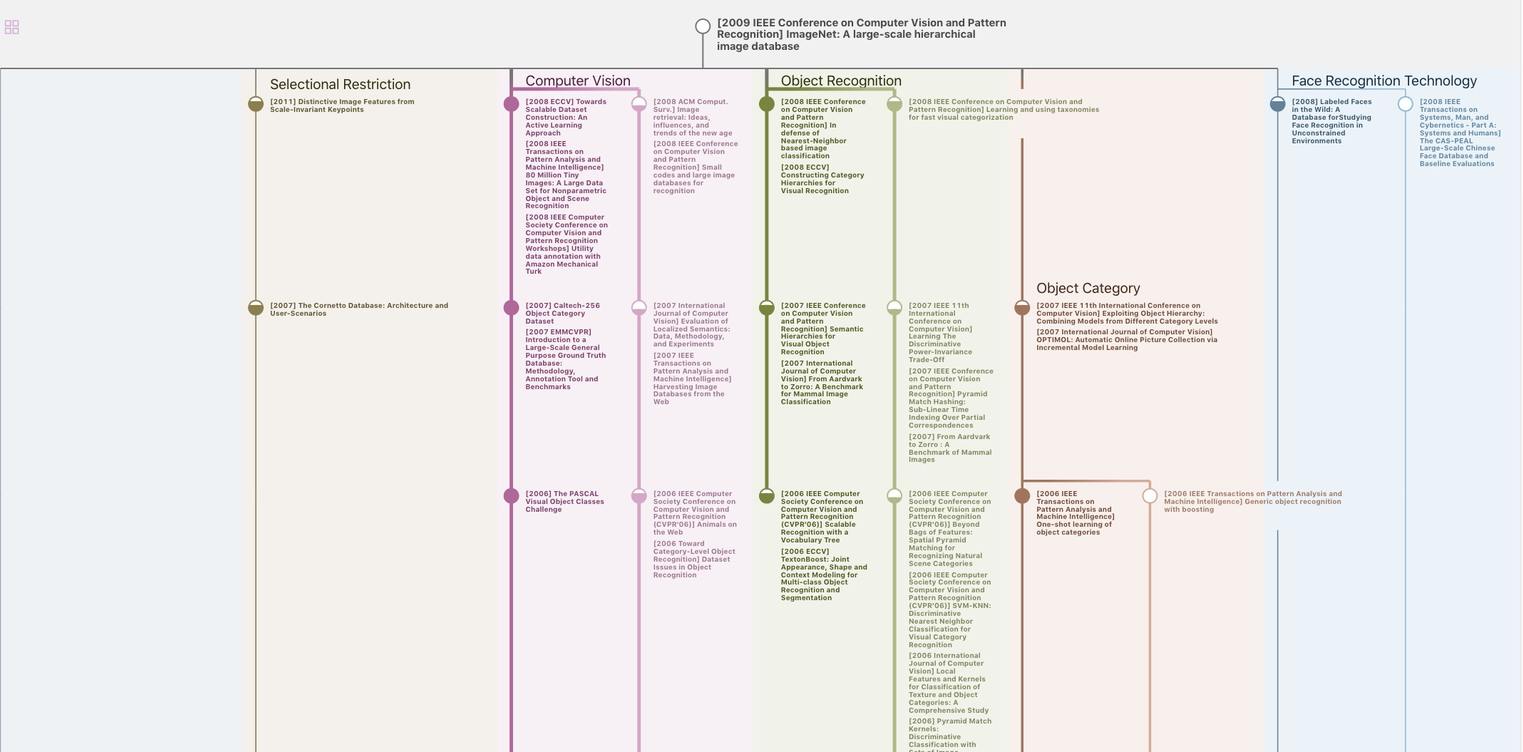
生成溯源树,研究论文发展脉络
Chat Paper
正在生成论文摘要