Modelling Time-Varying First and Second-Order Structure of Time Series via Wavelets and Differencing
arxiv(2022)
摘要
Most time series observed in practice exhibit time-varying trend (first-order) and autocovariance (second-order) behaviour. Differencing is a commonly-used technique to remove the trend in such series, in order to estimate the time-varying second-order structure (of the differenced series). However, often we require inference on the second-order behaviour of the original series, for example, when performing trend estimation. In this article, we propose a method, using differencing, to jointly estimate the time-varying trend and second-order structure of a nonstationary time series, within the locally stationary wavelet modelling framework. We develop a wavelet-based estimator of the second-order structure of the original time series based on the differenced estimate, and show how this can be incorporated into the estimation of the trend of the time series. We perform a simulation study to investigate the performance of the methodology, and demonstrate the utility of the method by analysing data examples from environmental and biomedical science.
更多查看译文
关键词
and phrases, Differencing, locally stationary time series, trend estimation, wavelet thresholding, wavelet spectrum
AI 理解论文
溯源树
样例
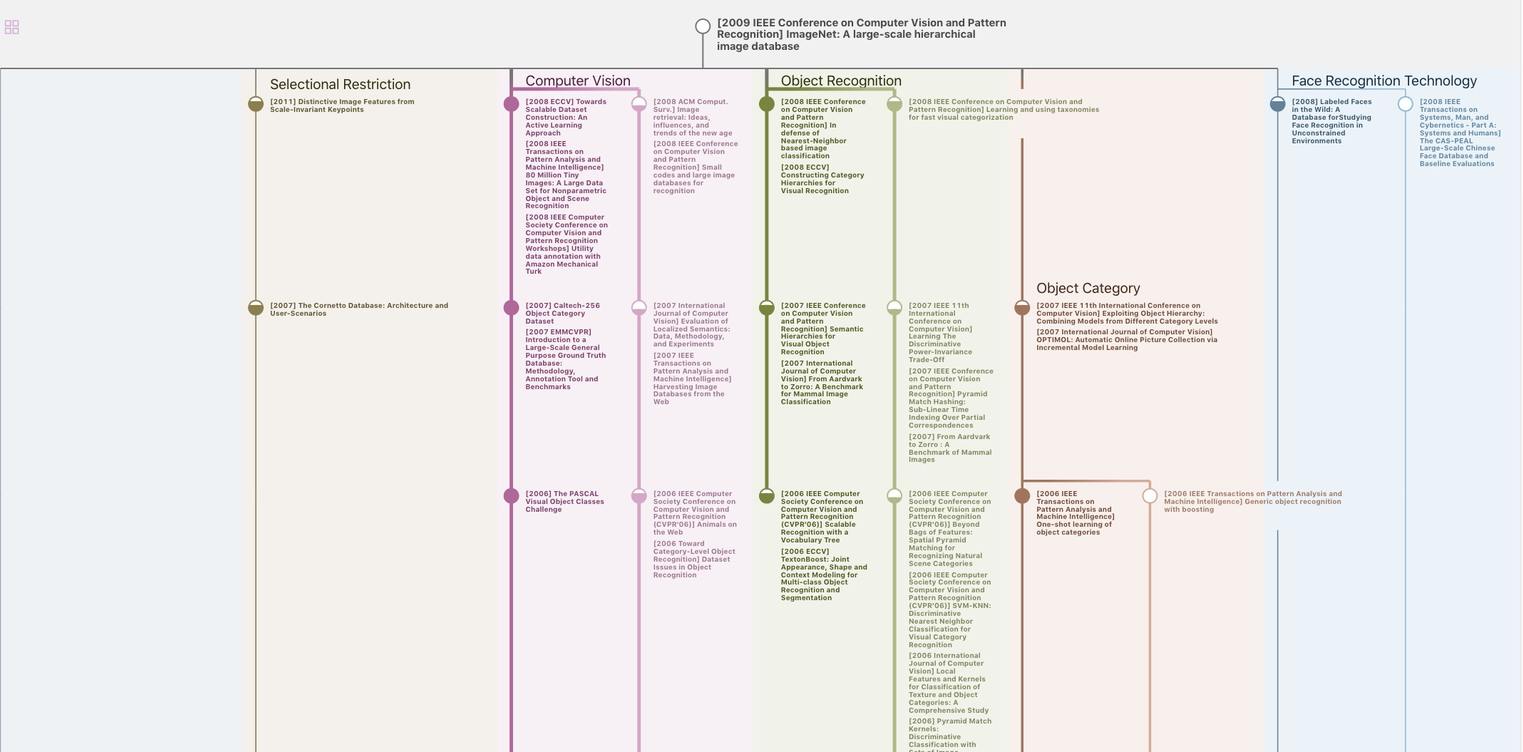
生成溯源树,研究论文发展脉络
Chat Paper
正在生成论文摘要