Aegis: A Trusted, Automatic and Accurate Verification Framework for Vertical Federated Learning
arXiv (Cornell University)(2021)
摘要
Vertical federated learning (VFL) leverages various privacy-preserving algorithms, e.g., homomorphic encryption or secret sharing based SecureBoost, to ensure data privacy. However, these algorithms all require a semi-honest secure definition, which raises concerns in real-world applications. In this paper, we present Aegis, a trusted, automatic, and accurate verification framework to verify the security of VFL jobs. Aegis is separated from local parties to ensure the security of the framework. Furthermore, it automatically adapts to evolving VFL algorithms by defining the VFL job as a finite state machine to uniformly verify different algorithms and reproduce the entire job to provide more accurate verification. We implement and evaluate Aegis with different threat models on financial and medical datasets. Evaluation results show that: 1) Aegis can detect 95% threat models, and 2) it provides fine-grained verification results within 84% of the total VFL job time.
更多查看译文
关键词
vertical federated learning,accurate verification framework
AI 理解论文
溯源树
样例
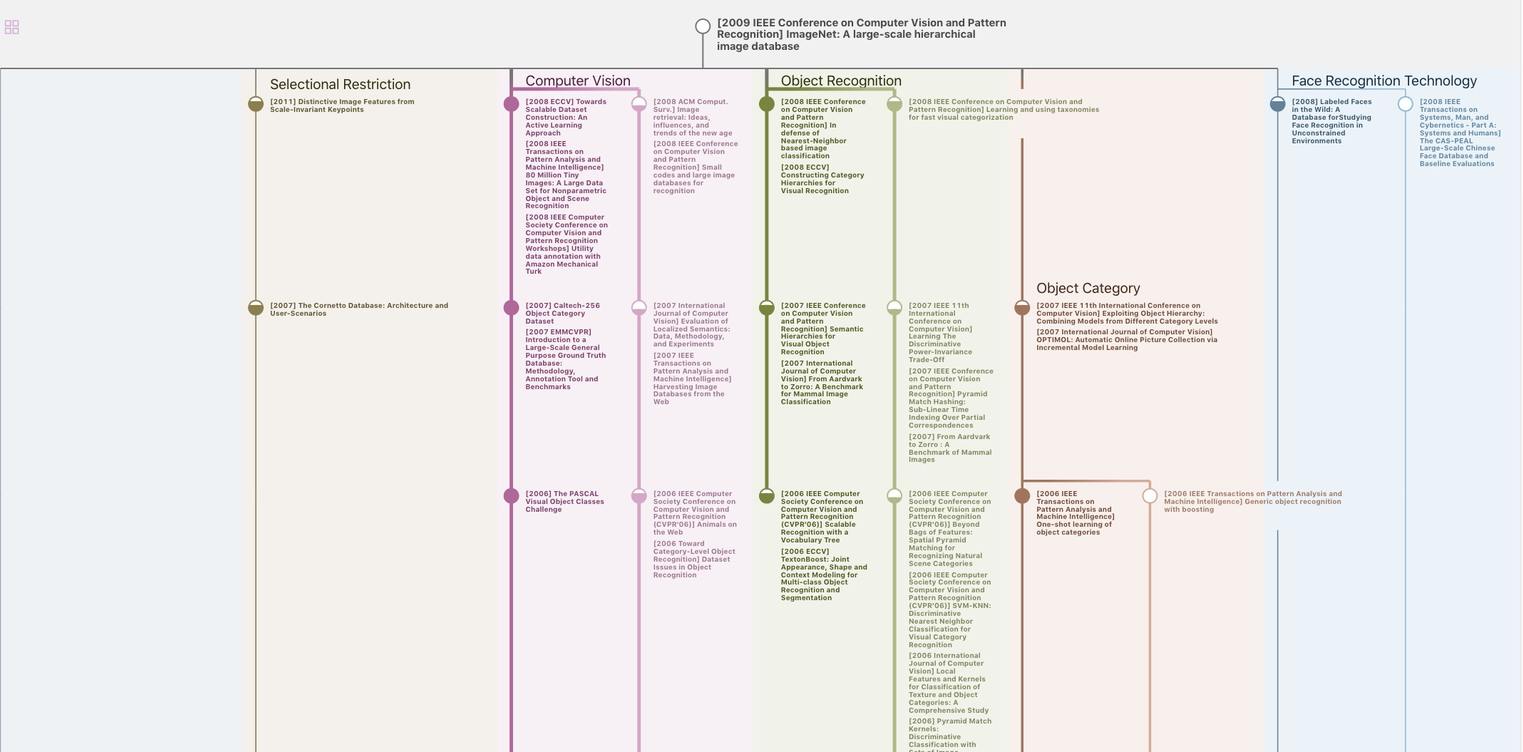
生成溯源树,研究论文发展脉络
Chat Paper
正在生成论文摘要