DRQN-based 3D Obstacle Avoidance with a Limited Field of View
2021 IEEE/RSJ INTERNATIONAL CONFERENCE ON INTELLIGENT ROBOTS AND SYSTEMS (IROS)(2021)
Abstract
In this paper, we propose a map-based end-to-end DRL approach for three-dimensional (3D) obstacle avoidance in a partially observed environment, which is applied to achieve autonomous navigation for an indoor mobile robot using a depth camera with a narrow field of view. We first train a neural network with LSTM units in a 3D simulator of mobile robots to approximate the Q-value function in double DRQN. We also use a curriculum learning strategy to accelerate and stabilize the training process. Then we deploy the trained model to a real robot to perform 3D obstacle avoidance in its navigation. We evaluate the proposed approach both in the simulated environment and on a robot in the real world. The experimental results show that the approach is efficient and easy to be deployed, and it performs well for 3D obstacle avoidance with a narrow observation angle, which outperforms other existing DRL-based models by 15.5% on success rate.
MoreTranslated text
Key words
DRQN-based 3D obstacle avoidance,map-based end-to-end DRL approach,three-dimensional obstacle avoidance,partially observed environment,autonomous navigation,indoor mobile robot,curriculum learning strategy,trained model,simulated environment,narrow observation angle,DRL-based models,limited field of view,depth camera,LSTM units,Q-value function
AI Read Science
Must-Reading Tree
Example
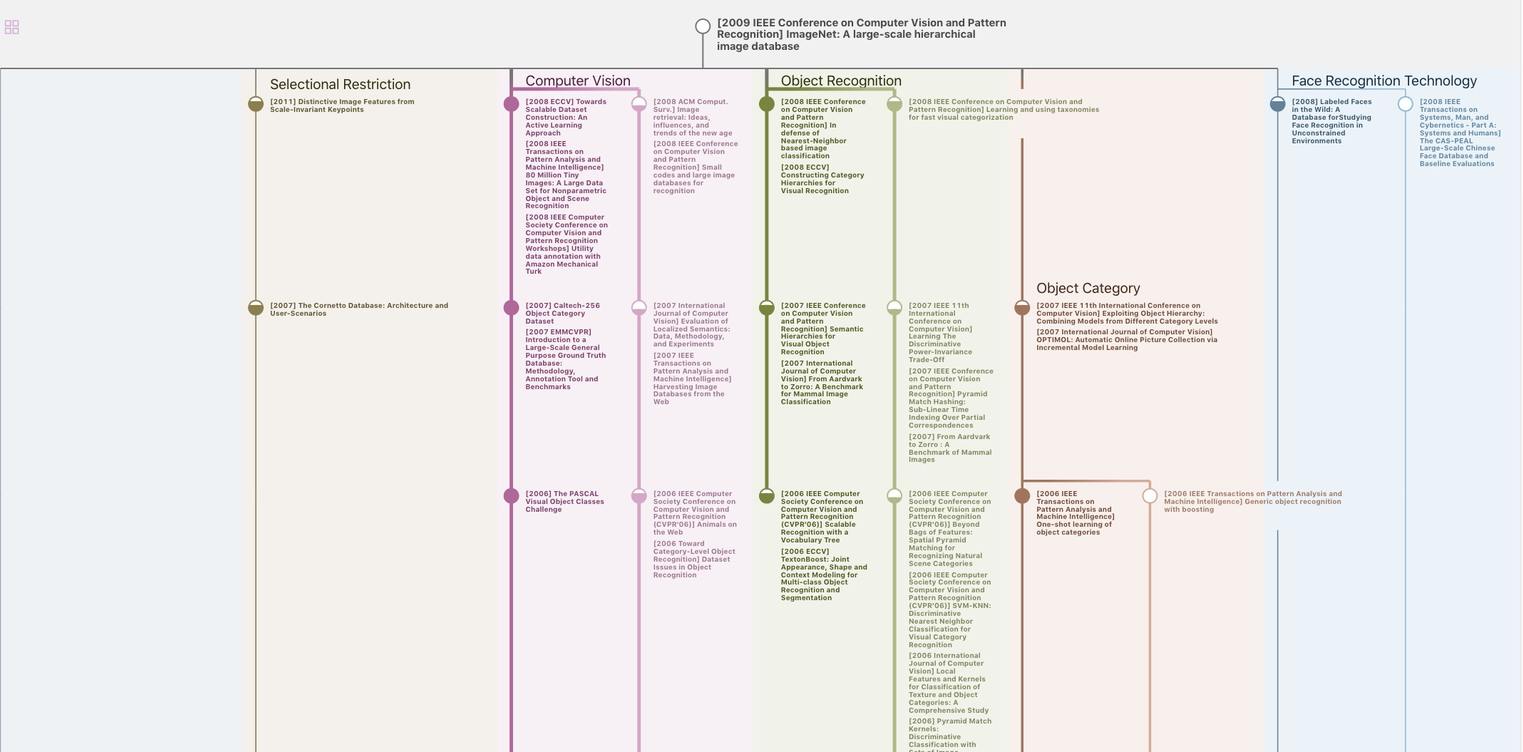
Generate MRT to find the research sequence of this paper
Chat Paper
Summary is being generated by the instructions you defined