Genetic Programming with Archive for Dynamic Flexible Job Shop Scheduling
2021 IEEE CONGRESS ON EVOLUTIONARY COMPUTATION (CEC 2021)(2021)
摘要
Genetic programming (GP) has achieved great success in evolving effective scheduling rules to make real-time decisions in dynamic flexible job shop scheduling (DFJSS). To improve generalization, a commonly used strategy is to change the training simulation(s) at each generation of the GP process. However, with such a simulation rotation, GP may lose potentially promising individuals that happen to perform poorly in one particular generation. To address this issue, this paper proposed a new multi-tree GP with archive (MTAGP) to evolve the routing and sequencing rules for DFJSS. The archive is used to store the potentially promising individuals of each generation during evolution of genetic programming. The individuals in the archive can then be fully utilized when the simulation is changed in subsequent generations. Through extensive experimental tests, the MTAGP algorithm proposed in this paper is more effective than the multi-tree GP without archive algorithm in a few scenarios. Further experiments were carried out to analyze the use of the archive and some possible guesses were ruled out. We argue that the use of archives does increase the diversity of the population. However, the number of individuals in the archive that ranked in the top five of the new population is small. Therefore, the archive may not be able to greatly improve the performance. In the future, we will investigate better ways to use the archive and better ways to update individuals in the archive.
更多查看译文
关键词
dynamic flexible job shop scheduling, genetic programming, archive
AI 理解论文
溯源树
样例
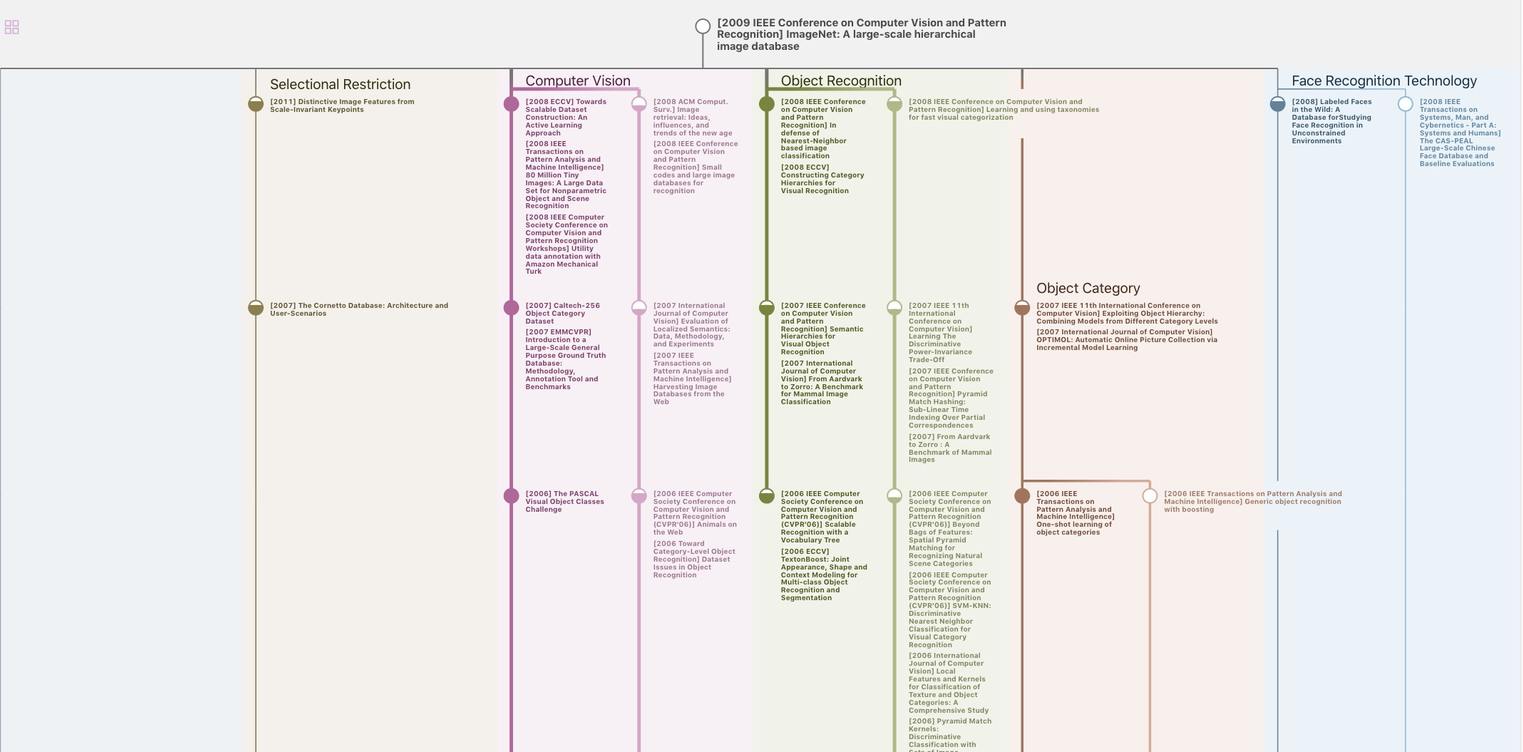
生成溯源树,研究论文发展脉络
Chat Paper
正在生成论文摘要