Parallel Evolutionary Algorithm for EEG Optimization Problems
2021 IEEE CONGRESS ON EVOLUTIONARY COMPUTATION (CEC 2021)(2021)
摘要
Big data optimization has become an important research topic in many disciplines. These optimization problems involve a large volume of data, from different sources, in different formats, that are generated at a high speed. For example, in the healthcare sector, electroencephalography (EEG), which is a method for monitoring brain signals and typically used to diagnose neurological disorders, generates a large amount of data which, however, is often captured with artifacts added from non-brain sources. Evolutionary algorithms are considered one of the most successful approaches for solving many such complex optimization problems. In this paper, a differential evolution algorithm is developed to remove artifacts from EEG signals of interest, by using the parallel computing ability of a Graphics Processing Unit. Two levels of parallelization, variable and individual, are implemented, with a gradient-based local search and adaptive control parameters incorporated in order to enhance a search’s convergence. The proposed algorithm is tested using six single objective problems from the 2015 big data optimization competition problems with 1024, 3072 and 4864 decision variables, as both noise-free and with white noise. The results presented in this paper indicate that the proposed algorithm is capable of achieving high-quality solutions, and is up to 374.7 faster than the state-of-the-art algorithms.
更多查看译文
关键词
Big optimization problems, big data, differential evolution, EEG signals
AI 理解论文
溯源树
样例
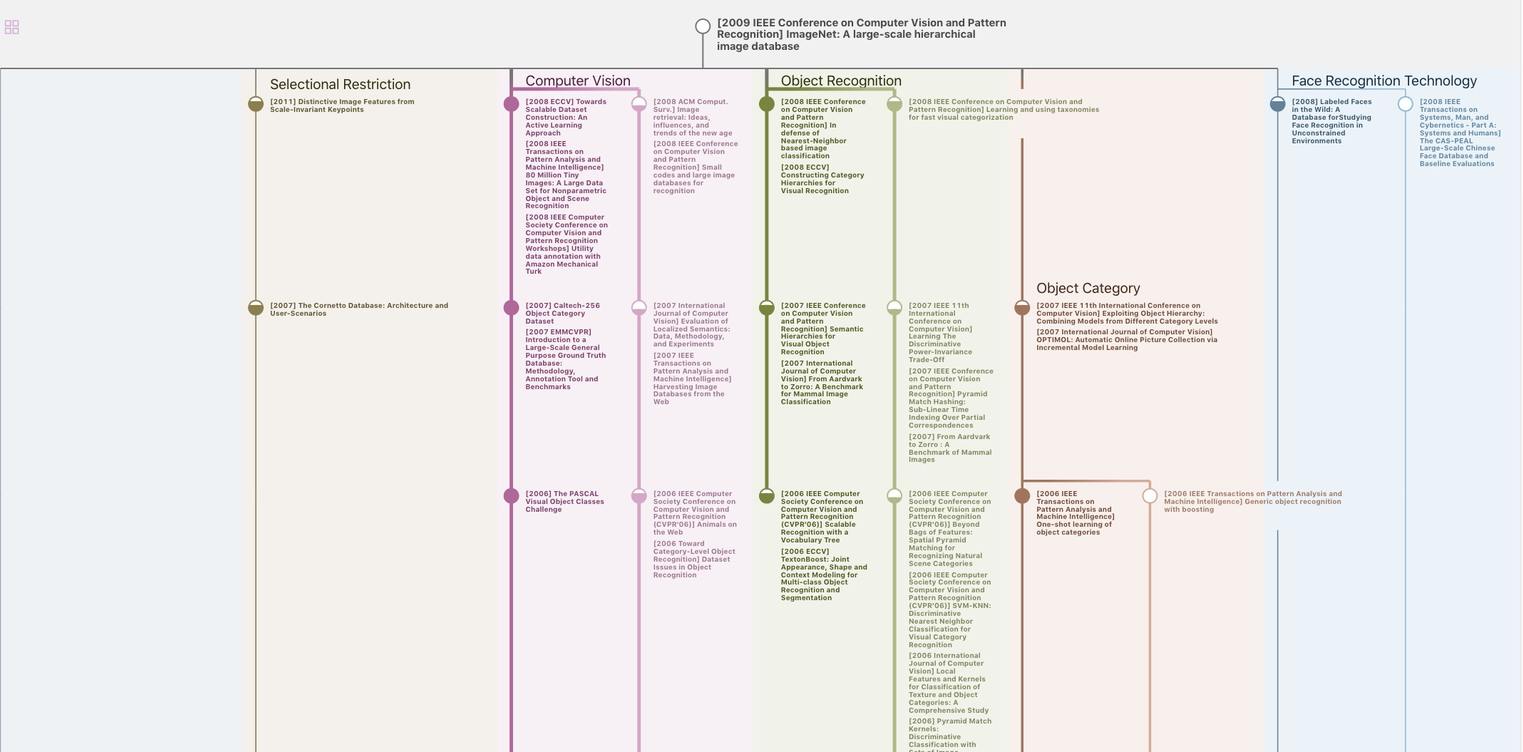
生成溯源树,研究论文发展脉络
Chat Paper
正在生成论文摘要