GASP: Graph-Based Approximate Sequential Pattern Mining for Electronic Health Records
NEW TRENDS IN DATABASE AND INFORMATION SYSTEMS, ADBIS 2021(2021)
摘要
Sequential pattern mining can be used to extract meaningful sequences from electronic health records. However, conventional sequential pattern mining algorithms that discover all frequent sequential patterns can incur a high computational and be susceptible to noise in the observations. Approximate sequential pattern mining techniques have been introduced to address these shortcomings yet, existing approximate methods fail to reflect the true frequent sequential patterns or only target single-item event sequences. Multi-item event sequences are prominent in healthcare as a patient can have multiple interventions for a single visit. To alleviate these issues, we propose GASP, a graph-based approximate sequential pattern mining, that discovers frequent patterns for multi-item event sequences. Our approach compresses the sequential information into a concise graph structure which has computational benefits. The empirical results on two healthcare datasets suggest that GASP outperforms existing approximate models by improving recoverability and extracts better predictive patterns.
更多查看译文
关键词
Sequential pattern mining, Healthcare data
AI 理解论文
溯源树
样例
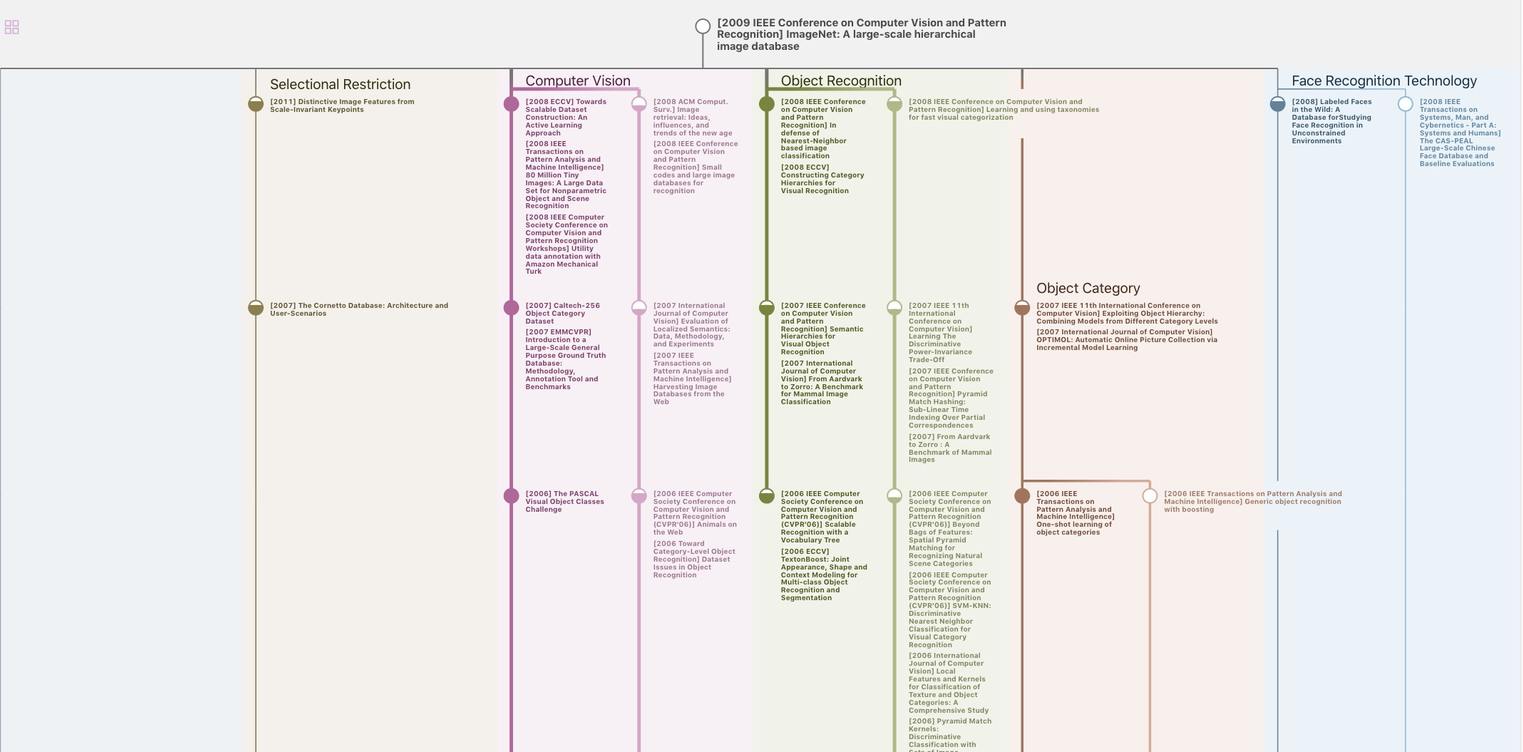
生成溯源树,研究论文发展脉络
Chat Paper
正在生成论文摘要