MimicNet: fast performance estimates for data center networks with machine learning
ACM SIGCOMM(2021)
Abstract
ABSTRACTAt-scale evaluation of new data center network innovations is becoming increasingly intractable. This is true for testbeds, where few, if any, can afford a dedicated, full-scale replica of a data center. It is also true for simulations, which while originally designed for precisely this purpose, have struggled to cope with the size of today's networks. This paper presents an approach for quickly obtaining accurate performance estimates for large data center networks. Our system,MimicNet, provides users with the familiar abstraction of a packet-level simulation for a portion of the network while leveraging redundancy and recent advances in machine learning to quickly and accurately approximate portions of the network that are not directly visible. MimicNet can provide over two orders of magnitude speedup compared to regular simulation for a data center with thousands of servers. Even at this scale, MimicNet estimates of the tail FCT, throughput, and RTT are within 5% of the true results.
MoreTranslated text
Key words
Network simulation, Data center networks, Approximation, Machine learning, Network modeling
AI Read Science
Must-Reading Tree
Example
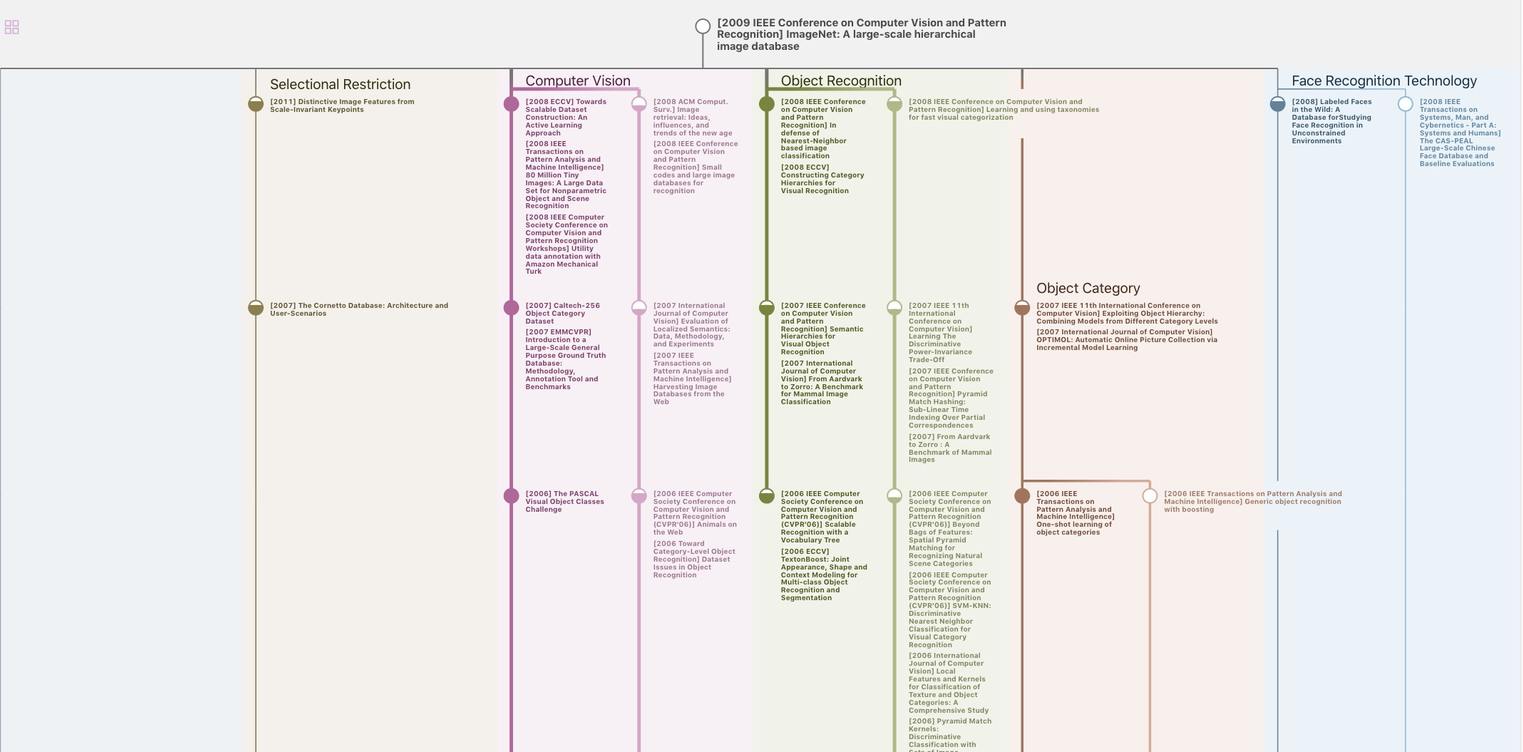
Generate MRT to find the research sequence of this paper
Chat Paper
Summary is being generated by the instructions you defined